Classification of Libya Floods and Morocco Earthquakes Using CNN-Based Deep Learning Models
2023 11th International Japan-Africa Conference on Electronics, Communications, and Computations (JAC-ECC)(2023)
摘要
Natural disaster's impact on human lives has been costly. The Libya floods and Morocco earthquakes affected more than two million people. Deep learning and computer vision techniques can mitigate the challenges brought by natural disasters. To enhance this field, a dataset from these two natural disasters, including aerial and lateral images, has been collected, augmented, and developed where 13 deep learning models, including AlexNet, VGG16, VGG19, GoogleNet, InceptionV3, ResNet-1S, ResNet-50, ResNet-101, DenseNet-201, NasNetLarge, EfficientNet-b0, MobileNetV2, and SqueezeNet have been tested. In addition, the newly developed YOLOvS deep learning models have been applied to the dataset. The YOLOvS-m model achieves the highest accuracy of 97.36 %. On the other hand, the SqueezeNet and YOLOvS-n model stand out for their lightweight nature, boasting a compact size of 2.658 MB and 3 MB, respectively. The AlexN et model exhibits a short training time of 367 seconds relative to other models that have been implemented. The YOLOvS-x model demonstrates the highest precision rate of 9S.4 %. Conversely, the YOLOvS-1 model exhibits the highest recall rate of 9S.51 % and the best F1-Score of 97.8 %.
更多查看译文
关键词
Natural disasters,Deep learning,Artificial Intelligence,Flood,Earthquake
AI 理解论文
溯源树
样例
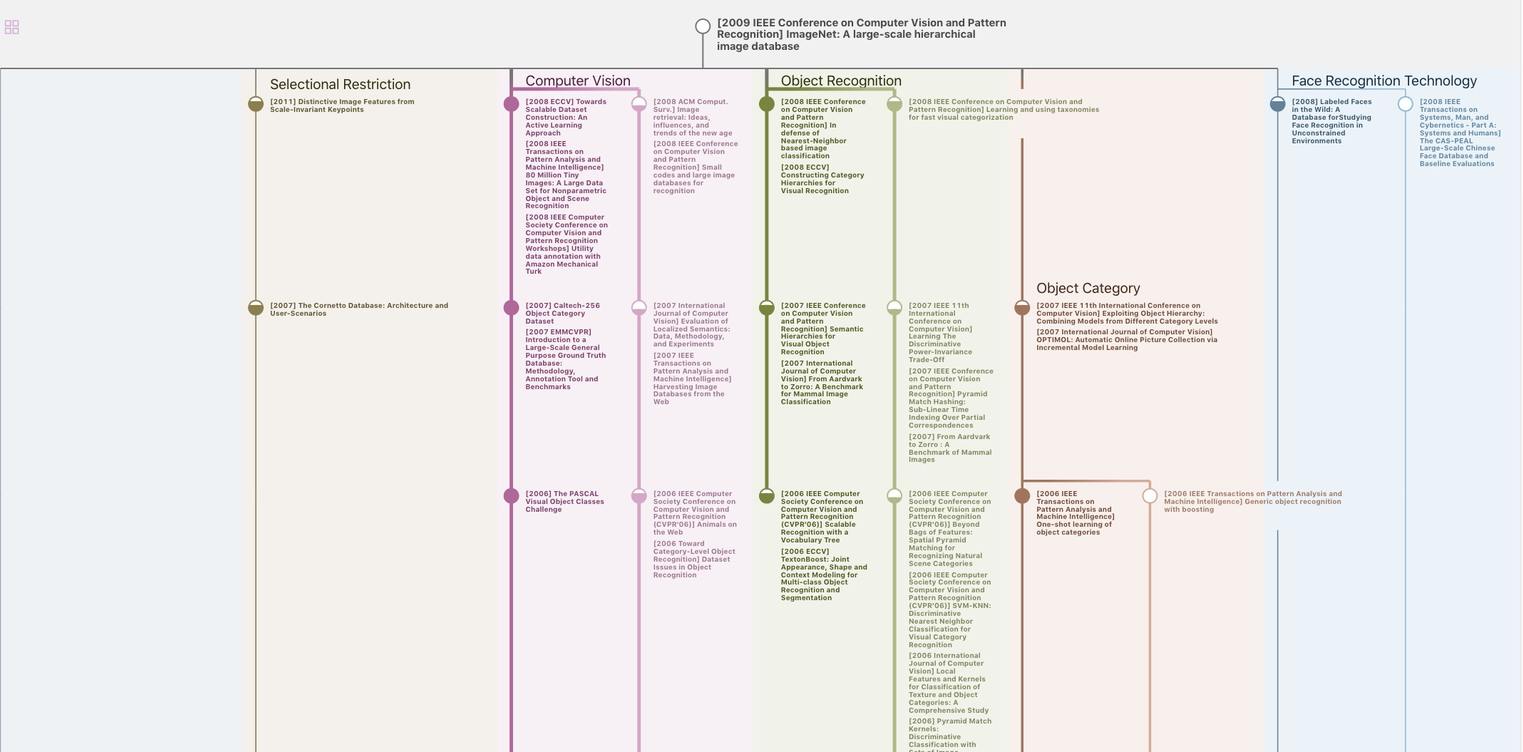
生成溯源树,研究论文发展脉络
Chat Paper
正在生成论文摘要