Leveraging Nucleotide Dependencies for Improved mRNA Vaccine Degradation Prediction.
ACS/IEEE International Conference on Computer Systems and Applications(2023)
摘要
RNA sequence properties prediction is significant for understanding RNA function and its potential applications in medicine and biotechnology. In this study, we developed a novel Gated Recurrent Unit (GRU) deep learning model to predict mRNA vaccine degradation with improved accuracy over traditional machine learning methods and previously reported deep learning approaches. A notable contribution of our approach is the innovative method of feature engineering that accounts for dependencies between nucleotides by shifting the feature values. Our proposed GRU model outperformed XGBoost, Random Forest, and LightGBM models. The GRU Network showed a Mean Columnwise Root Mean Squared Error (MCRMSE) of 0.275 and 0.389 for the public and the private sets, respectively. Despite some limitations, our model provides a strong foundation for future work to refine and expand the capabilities of RNA sequence property prediction. The results of this study have significant implications for RNA research, potentially leading to advancements in understanding RNA function and the development of RNA-targeting therapeutics and diagnostic tools.
更多查看译文
关键词
mRNA vaccine degradation,deep learning,gated recurrent units (GRU),vaccine development,RNA sequence properties
AI 理解论文
溯源树
样例
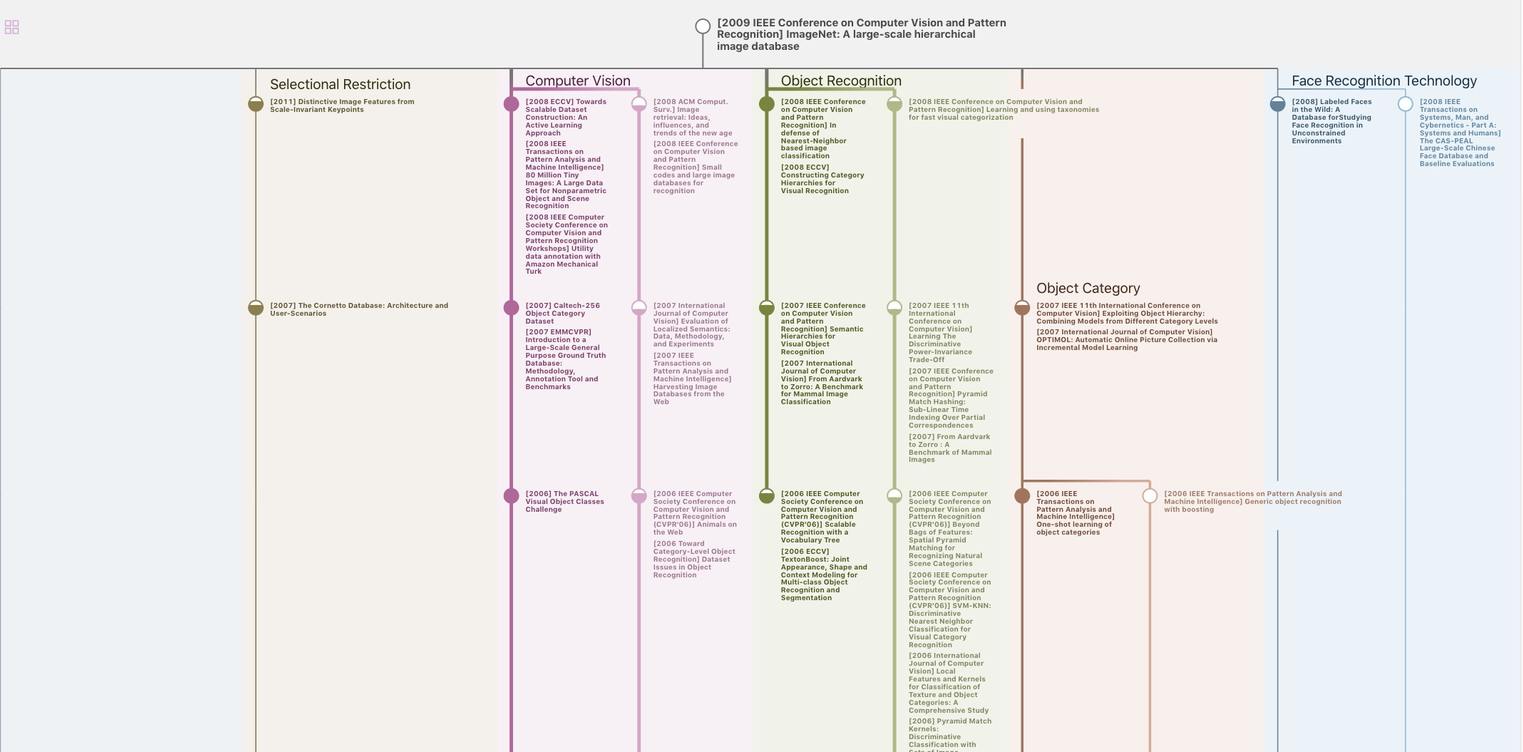
生成溯源树,研究论文发展脉络
Chat Paper
正在生成论文摘要