Dynamic Learnable Logit Adjustment for Long-Tailed Visual Recognition
IEEE Transactions on Circuits and Systems for Video Technology(2024)
摘要
Logit adjustment is an effective long-tailed visual recognition strategy to encourage a significant margin between rare and dominant labels. Existing methods typically employ the globally fixed label frequencies throughout the training to adjust margins. However, in practice, we observe that the local (in-batch) label frequencies change dynamically or even vanish for some classes (especially the tail classes) in batch-dependent training, which is inconsistent with global ones. Furthermore, our analyses reveal that the intra-class
collinear samples
actually do not contribute to the gradient update, but substantially increase the corresponding local label frequencies. Such contributions are spurious due to over-counting the label frequencies without contributing to the gradient. All of these will cause serious interference in precisely estimating local frequencies of the authentic contribution, leading to inauthentic margins. To simultaneously address the above issues, this paper innovatively proposes Dynamic Learnable Logit Adjustment (DLLA) loss to learn the local label frequencies within dynamic mini-batches precisely. Specifically, DLLA owns two complementary parts: 1)
rank-metric
eliminates spurious contributions from collinear samples by calculating the algebraic rank of the feature subspace in the mini-batch. 2)
class-supplement
ensures all classes appear in every mini-batch by inserting the corresponding learnable class prototype, for which we resort to neural collapse theory to make them align to the ideal regular simplex structure. Extensive experiments on standard benchmark datasets verify the effectiveness of our method.
更多查看译文
关键词
Long-tailed learning,Logit adjustment,Algebraic rank,Neural collapse
AI 理解论文
溯源树
样例
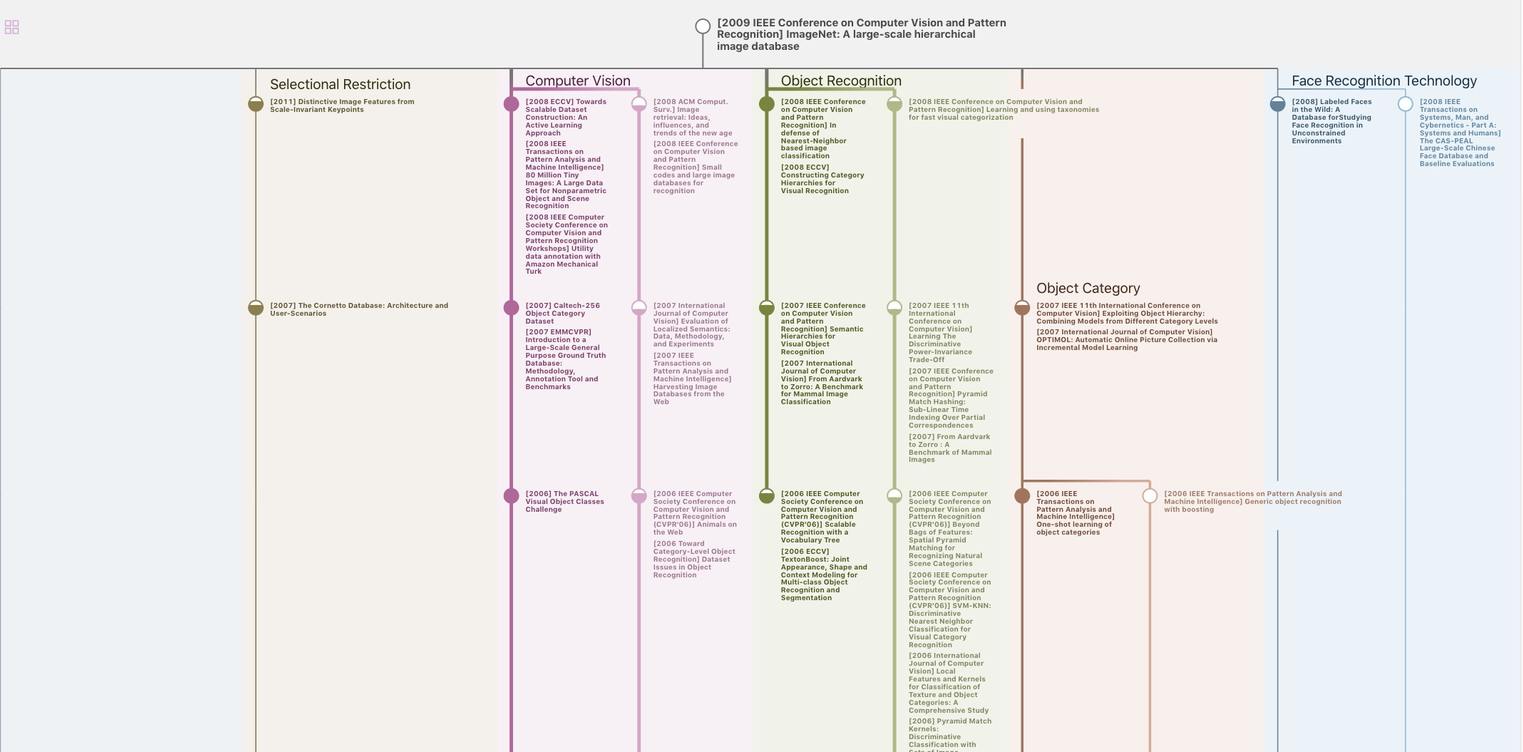
生成溯源树,研究论文发展脉络
Chat Paper
正在生成论文摘要