A Novel Methodology for Improving Applications of Modern Predictive Modeling Techniques to Linked Data Sets Subject to Mismatch Error
2023 Big Data Meets Survey Science (BigSurv)(2023)
摘要
In recent years, the rise of social media platforms such as Twitter/X has provided social scientists with a wealth of user-content data. Combining social media and survey data has the potential to produce a comprehensive source of information for social research. These data are often collected from multiple sources and combined by probabilistic record linkage. For the analysis of these linked data files, advanced machine learning techniques, such as random forests, boosting, and related ensemble methods, have become essential tools for survey methodologists and data scientists. There is, however, a potential pitfall in the widespread application of these techniques to linked data sets that needs more attention. Linkage errors such as mismatch and missed-match errors can distort the true relationships between variables and adversely alter the performance metrics routinely output by predictive modeling techniques, such as variable importance, confusion matrices, RMSE, etc. Thus, the actual predictive performance of these machine-learning techniques may not be realized. In this paper, we describe a methodology designed to adjust modern predictive modeling techniques for the presence of mismatch errors in linked data sets. The proposed approach, based on mixture modeling, is general enough to accommodate various predictive modeling techniques in a unified fashion. We evaluate the performance of our proposed methodology with simulations implemented in R. We conclude with recommendations for future work.
更多查看译文
关键词
record linkage,data integration,social media,Twitter/X,ensemble methods,bagging trees,random forests,mismatch error,mixture model,secondary analysis
AI 理解论文
溯源树
样例
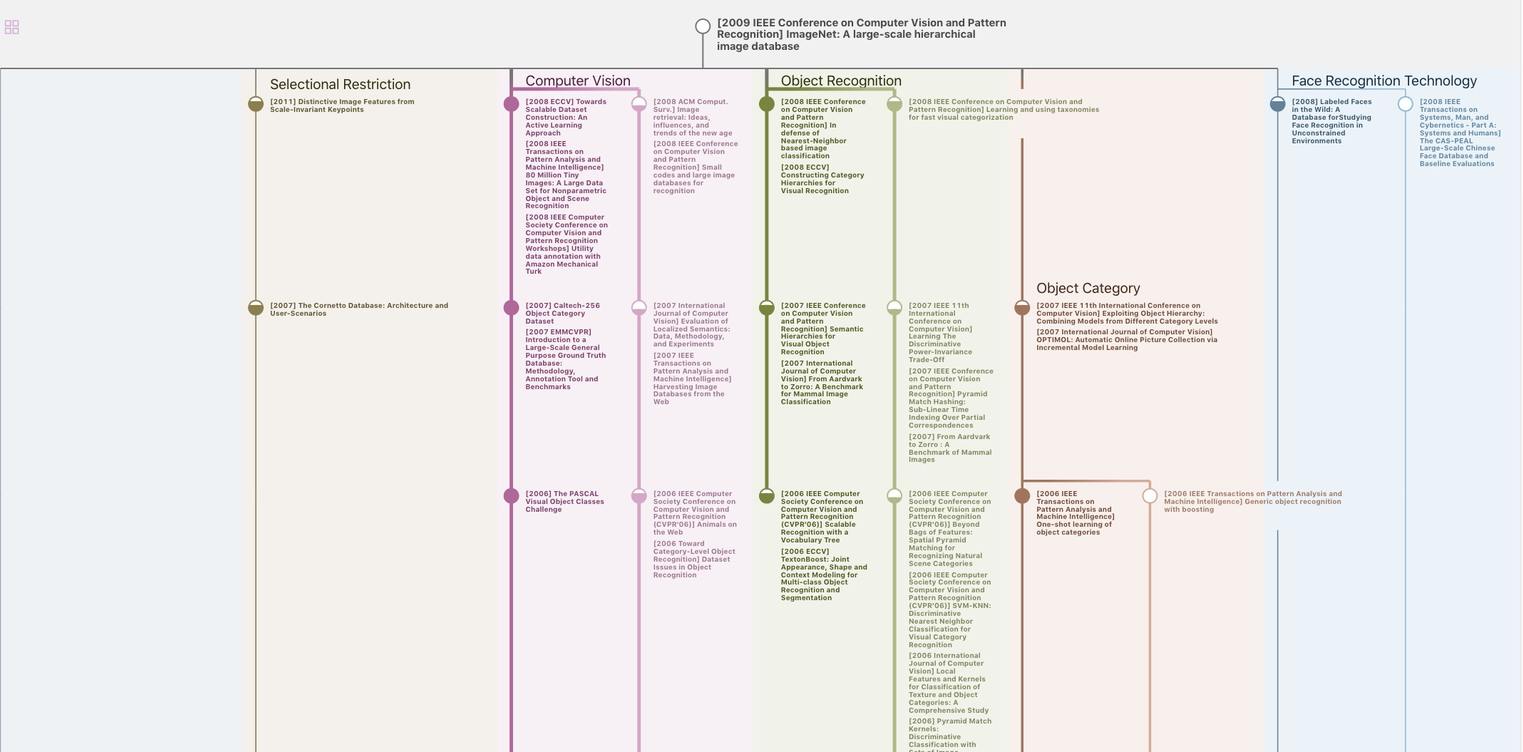
生成溯源树,研究论文发展脉络
Chat Paper
正在生成论文摘要