The covariance environment defines cellular niches for spatial inference
NATURE BIOTECHNOLOGY(2024)
摘要
A key challenge of analyzing data from high-resolution spatial profiling technologies is to suitably represent the features of cellular neighborhoods or niches. Here we introduce the covariance environment (COVET), a representation that leverages the gene-gene covariate structure across cells in the niche to capture the multivariate nature of cellular interactions within it. We define a principled optimal transport-based distance metric between COVET niches that scales to millions of cells. Using COVET to encode spatial context, we developed environmental variational inference (ENVI), a conditional variational autoencoder that jointly embeds spatial and single-cell RNA sequencing data into a latent space. ENVI includes two decoders: one to impute gene expression across the spatial modality and a second to project spatial information onto single-cell data. ENVI can confer spatial context to genomics data from single dissociated cells and outperforms alternatives for imputing gene expression on diverse spatial datasets. Using gene-gene covariance structure to represent cellular neighborhoods improves the integration of single-cell RNA sequencing and spatial transcriptomics data.
更多查看译文
AI 理解论文
溯源树
样例
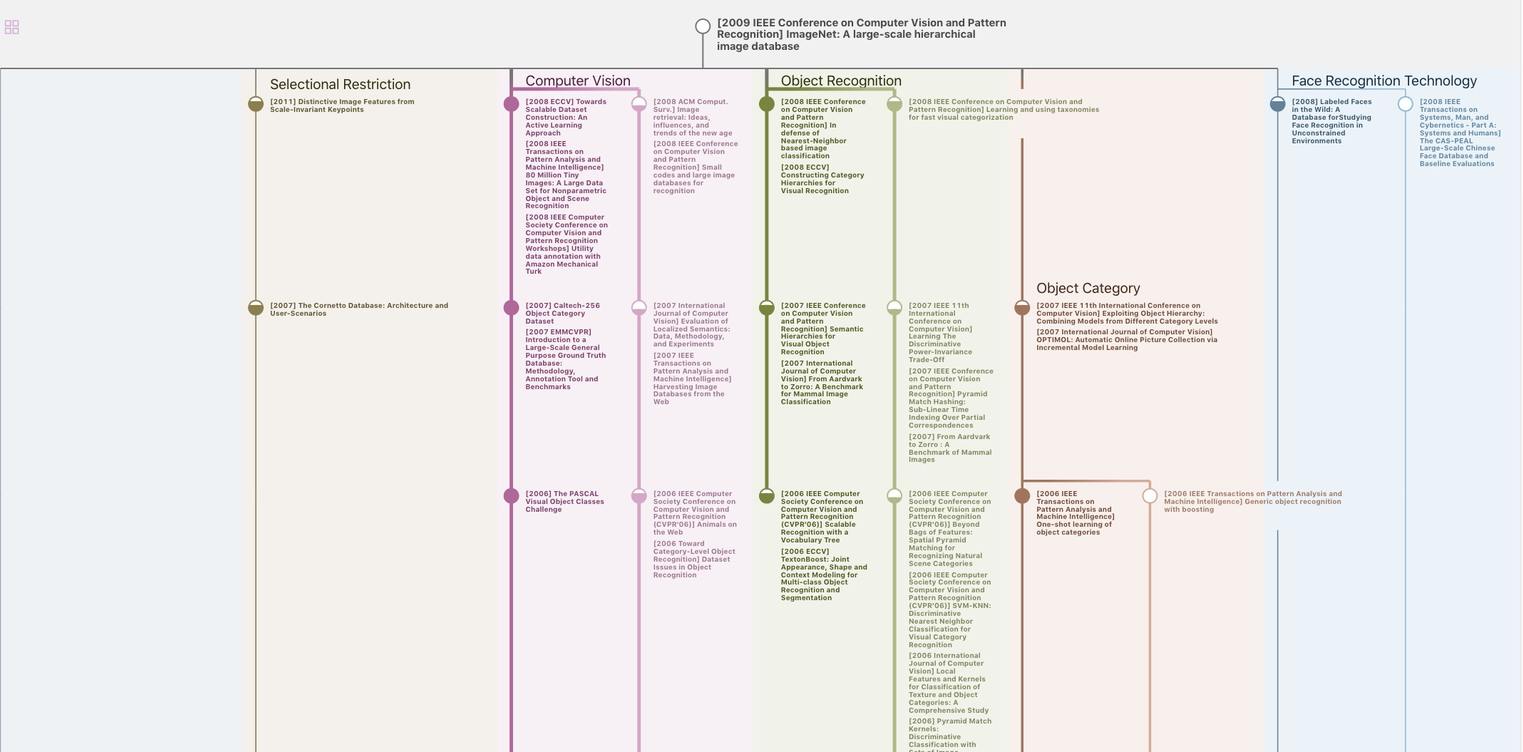
生成溯源树,研究论文发展脉络
Chat Paper
正在生成论文摘要