Parsimonious ultrametric Gaussian mixture models
Statistics and Computing(2024)
摘要
Gaussian mixture models represent a conceptually and mathematically elegant class of models for casting the density of a heterogeneous population where the observed data is collected from a population composed of a finite set of G homogeneous subpopulations with a Gaussian distribution. A limitation of these models is that they suffer from the curse of dimensionality, and the number of parameters becomes easily extremely large in the presence of high-dimensional data. In this paper, we propose a class of parsimonious Gaussian mixture models with constrained extended ultrametric covariance structures that are capable of exploring hierarchical relations among variables. The proposal shows to require a reduced number of parameters to be fit and includes constrained covariance structures across and within components that further reduce the number of parameters of the model.
更多查看译文
关键词
Model-based clustering,Hierarchical models,Ultrametricity,Parsimony,Dimensionality reduction
AI 理解论文
溯源树
样例
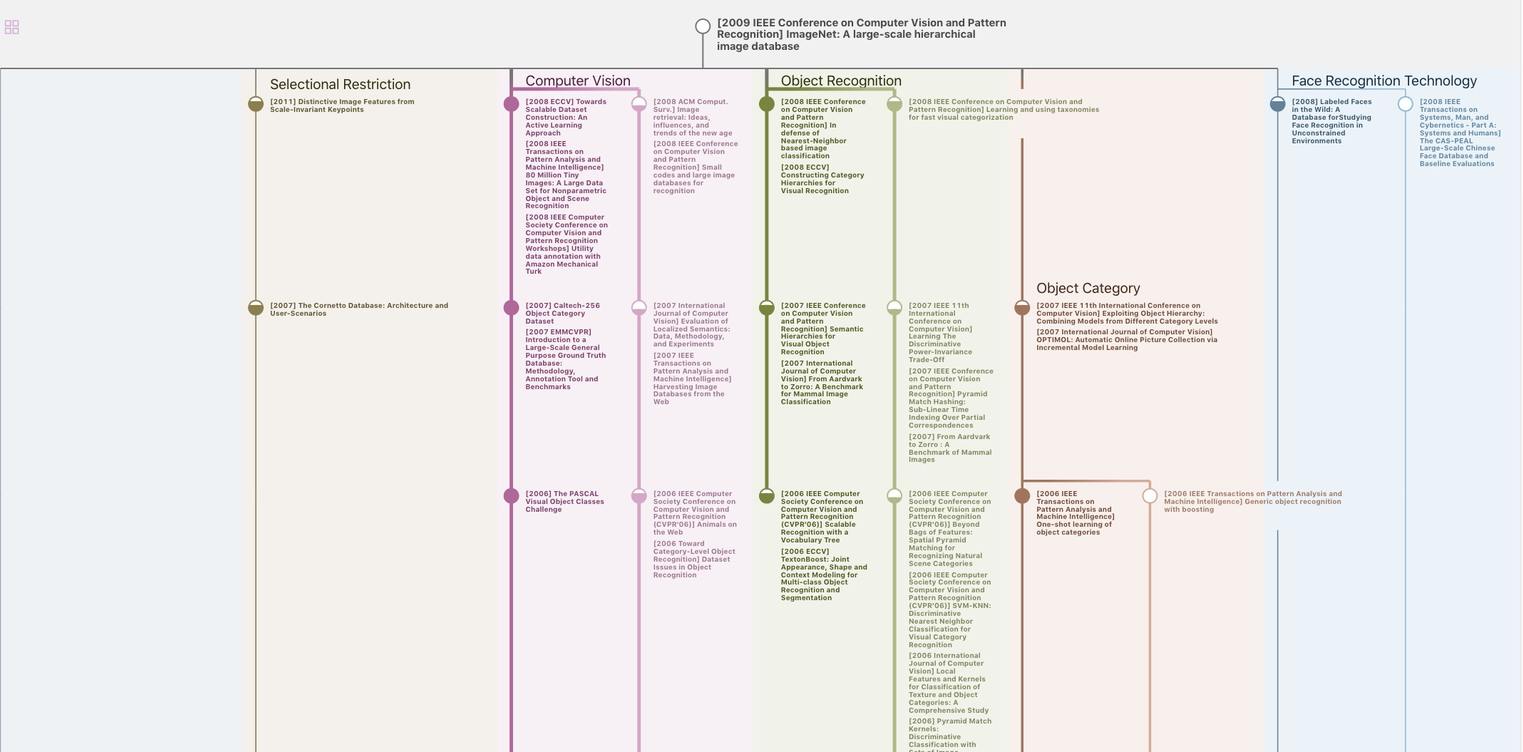
生成溯源树,研究论文发展脉络
Chat Paper
正在生成论文摘要