Nonlinear ensemble filtering with diffusion models: Application to the surface quasi-geostrophic dynamics
arxiv(2024)
摘要
The intersection between classical data assimilation methods and novel
machine learning techniques has attracted significant interest in recent years.
Here we explore another promising solution in which diffusion models are used
to formulate a robust nonlinear ensemble filter for sequential data
assimilation. Unlike standard machine learning methods, the proposed
Ensemble Score Filter (EnSF) is completely training-free and can
efficiently generate a set of analysis ensemble members. In this study, we
apply the EnSF to a surface quasi-geostrophic model and compare its performance
against the popular Local Ensemble Transform Kalman Filter (LETKF), which makes
Gaussian assumptions on the posterior distribution. Numerical tests demonstrate
that EnSF maintains stable performance in the absence of localization and for a
variety of experimental settings. We find that EnSF achieves competitive
performance relative to LETKF in the case of linear observations, but leads to
significant advantages when the state is nonlinearly observed and the numerical
model is subject to unexpected shocks. A spectral decomposition of the analysis
results shows that the largest improvements over LETKF occur at large scales
(small wavenumbers) where LETKF lacks sufficient ensemble spread. Overall, this
initial application of EnSF to a geophysical model of intermediate complexity
is very encouraging, and motivates further developments of the algorithm for
more realistic problems.
更多查看译文
AI 理解论文
溯源树
样例
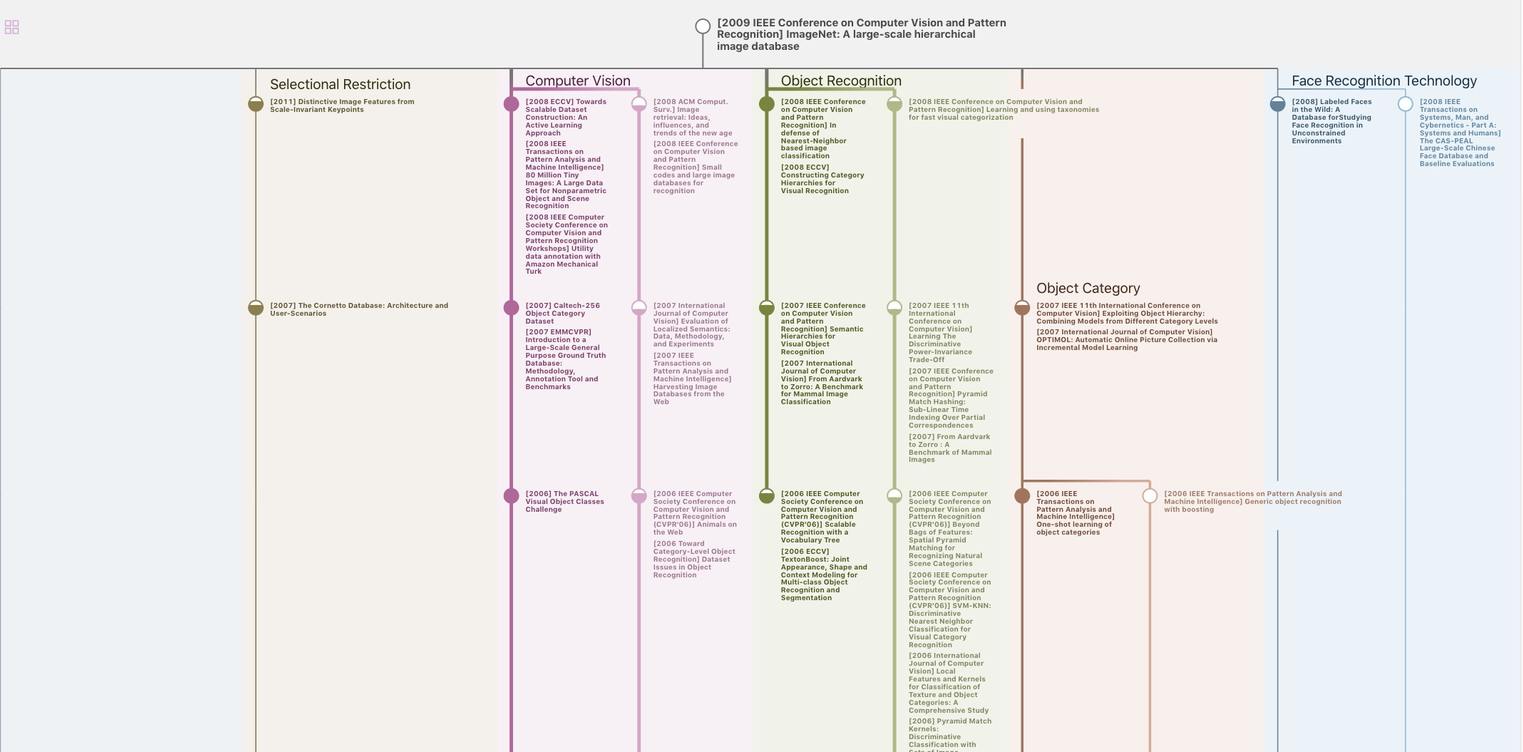
生成溯源树,研究论文发展脉络
Chat Paper
正在生成论文摘要