3D-GhostNet: A novel spatial-spectral algorithm to improve foodborne bacteria classification coupled with hyperspectral microscopic imaging technology
Sensors and Actuators B: Chemical(2024)
摘要
A novel 3D-GhostNet has been developed for hyperspectral microscopic imaging (HMI) data analysis to improve classification of foodborne pathogens. The HMI, leveraging microscopic technology, surpasses traditional spectral imaging in terms of sensitivity and resolution by utilizing visible/near-infrared spectroscopy integrated with high-resolution single-cell images. The newly constructed 3D-Ghost network directly processes and recognizes single-cell hypercube, enables HMI technology to become near-real-time detection by combining with automated data processing pipeline. 3D-GhostNet incorporates Ghost modules as its backbone and adds convolutional block attention module (CBAM) for adaptively extracting high-dimensional depth spatial-spectral information. In the task of identifying four different foodborne bacteria cells, our 3D-GhostNet achieved 100% accuracy, surpassing spectral-only-based (SOB) classifiers such as linear discriminant analysis (LDA, 89.7%), support vector machine (SVM, 93.9%), 1D convolutional neural network (1D-CNN, 98%), and long short-term memory (LSTM, 98.5%). Furthermore, our independent blind test results indicate that 3D-GhostNet remains robust for identifying complex mixtures of pathogens. As a label-free detection tool, 3D-GhostNet-assisted HMI technology is fully competent in classification of different foodborne pathogens, suggesting broad applications in food safety and quality.
更多查看译文
关键词
Hyperspectral microscope imaging,Foodborne pathogen,Rapid detection,3D hypercube classification
AI 理解论文
溯源树
样例
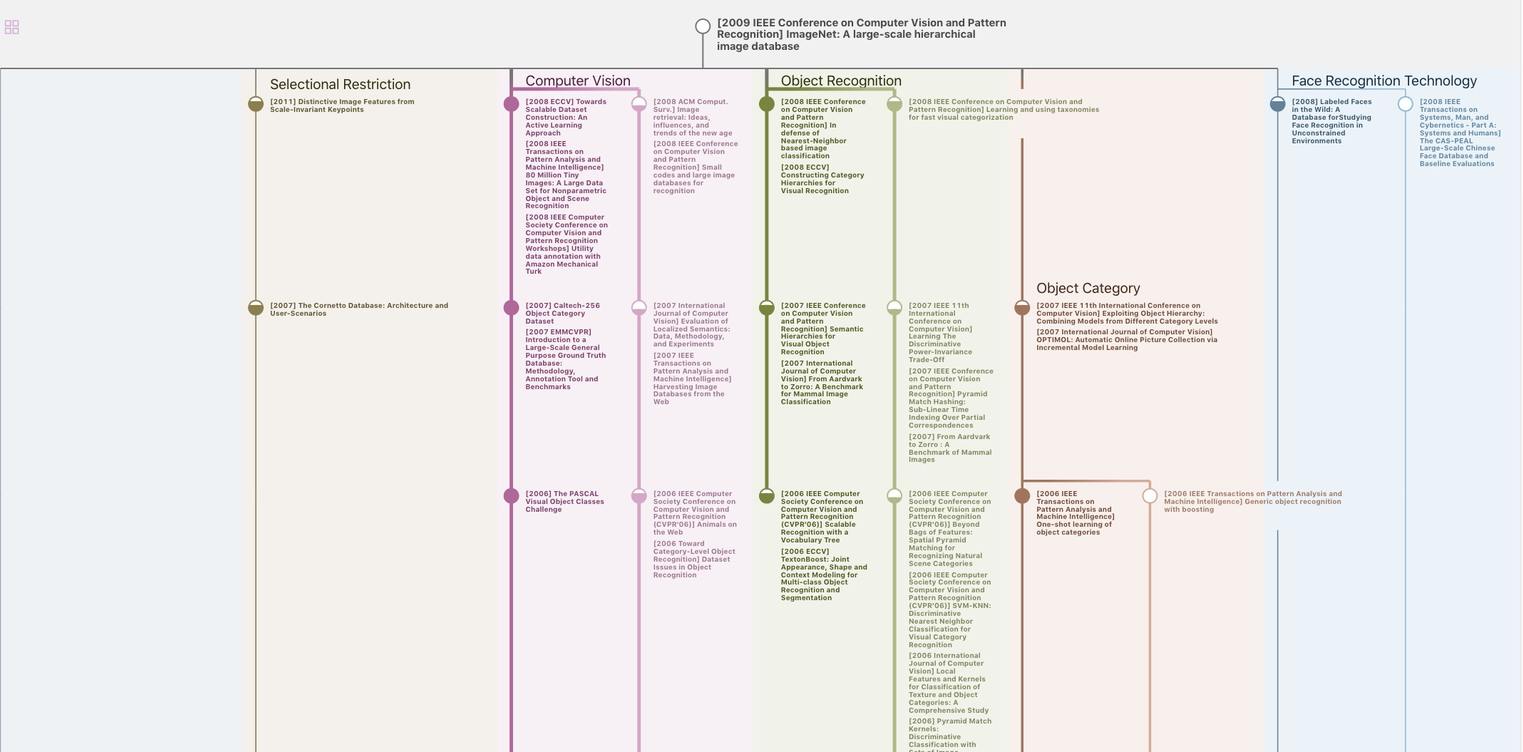
生成溯源树,研究论文发展脉络
Chat Paper
正在生成论文摘要