OctaveNet: An efficient multi-scale pseudo-siamese network for change detection in remote sensing images
Multimedia Tools and Applications(2024)
摘要
Urbanization and changes in land use are crucial for national development. Managing and monitoring these changes have become easier and more accurate due to advancements in imaging systems and change detection methods. In recent years, convolutional neural networks have gained significant attention and advancement in remote sensing change detection research. However, designing a model that reduces computational complexity while improving detection accuracy is still under discussion. In this regard, an efficient model based on pseudo-siamese convolutional networks had been developed in this research, which by combining multi-scale features at different levels, increased the accuracy of the final change map, especially in images with both different spatial resolutions and scales. Our model is inspired by Scale Invariant Feature Transform (SIFT), which uses multiple scale-spaces to identify scale-independent keypoints in images. We designed our model based on this concept, in which After passing the bi-temporal images through the encoder network and making the initial feature maps, the feature learning process takes place at several levels of different scales and finally, the change map is calculated. In addition, the proposed architecture leverages point-wise, depth-wise, and neighboring features across various scale levels to harness the optimal features present in high-resolution remote sensing images. Simplicity in design, effectiveness in calculations, and accuracy make our model surpass popular methods such as BIT, DSIFN, etc. The evaluation results on 4 different datasets and comparison with 12 state-of-the-art models demonstrate that the proposed method outperforms other popular models in change detection. Available at: https://github.com/farhadinima75/OctaveNet .
更多查看译文
关键词
Change detection,Remote sensing,High spatial-resolution images,Deep learning,Pseudo-siamese convolutional neural networks
AI 理解论文
溯源树
样例
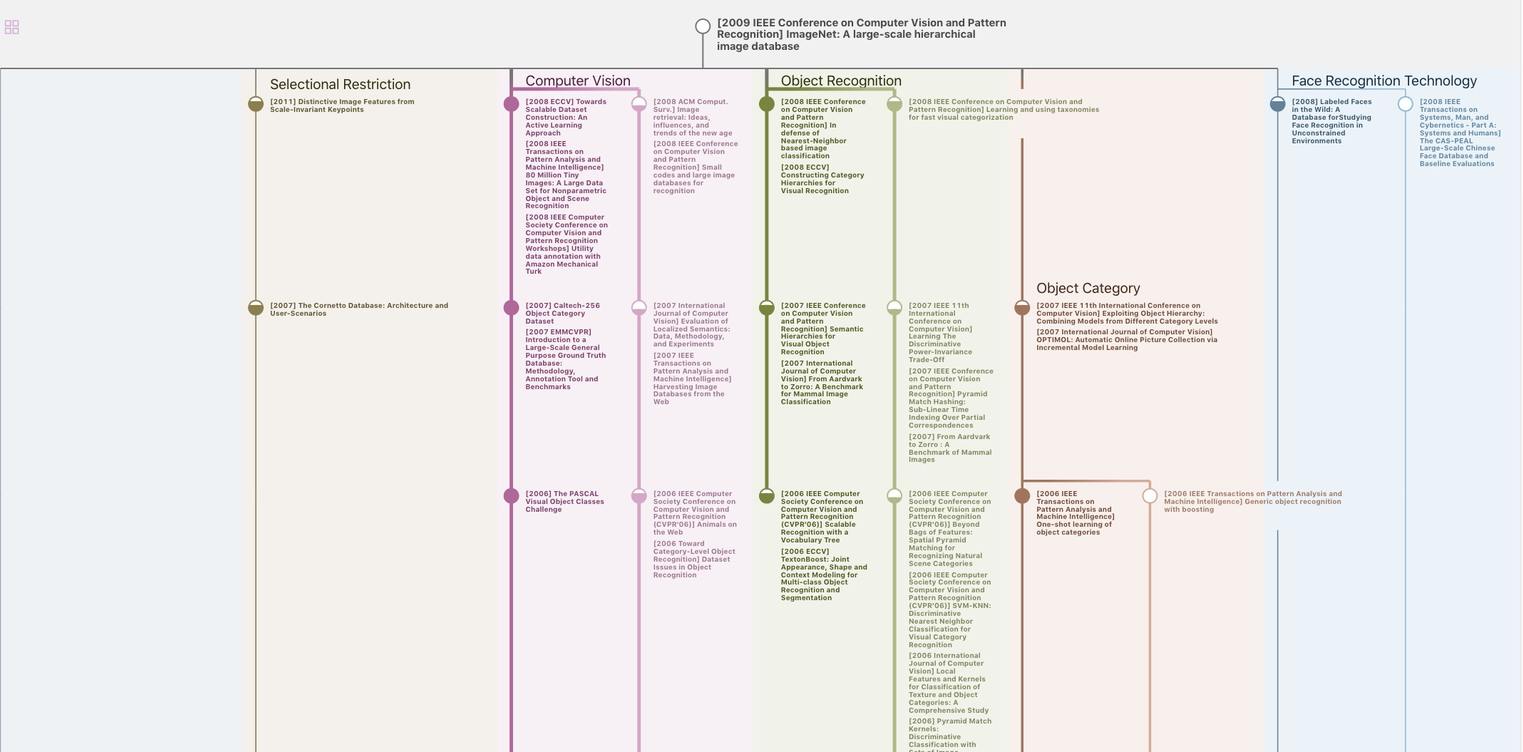
生成溯源树,研究论文发展脉络
Chat Paper
正在生成论文摘要