Enhancing extractive text summarization using natural language processing with an optimal deep learning model
AIMS MATHEMATICS(2024)
摘要
Natural language processing (NLP) performs a vital function in text summarization, a task targeted at refining the crucial information from the massive quantity of textual data. NLP methods allow computers to comprehend and process human language, permitting the development of advanced summarization methods. Text summarization includes the automatic generation of a concise and coherent summary of a specified document or collection of documents. Extracting significant insights from text data is crucial as it provides advanced solutions to end -users and business organizations. Automatic text summarization (ATS) computerizes text summarization by decreasing the initial size of the text without the loss of main data features. Deep learning (DL) approaches exhibited significant performance in abstractive and extractive summarization tasks. This research designed an extractive text summarization using NLP with an optimal DL (ETS-NLPODL) model. The major goal of the ETS-NLPODL technique was to exploit feature selection with a hyperparameter-tuned DL model for summarizing the text. In the ETS-NLPODL technique, an initial step of data preprocessing was involved to convert the input text into a compatible format. Next, a feature extraction process was carried out and the optimal set of features was chosen by the hunger games search optimization (HGSO) algorithm. For text summarization, the ETS-NLPODL model used an attention -based convolutional neural network with a gated recurrent unit (ACNN-GRU) model. Finally, the mountain gazelle optimization (MGO) algorithm was employed for the optimal hyperparameter selection of the ACNNGRU model. The experimental results of the ETS-NLPODL system were examined under the benchmark dataset. The experimentation outcomes pointed out that the ETS-NLPODL technique gained better performance over other methods concerning diverse performance measures.
更多查看译文
关键词
text summarization,natural language processing,mountain gazelle optimization,feature extraction,deep learning
AI 理解论文
溯源树
样例
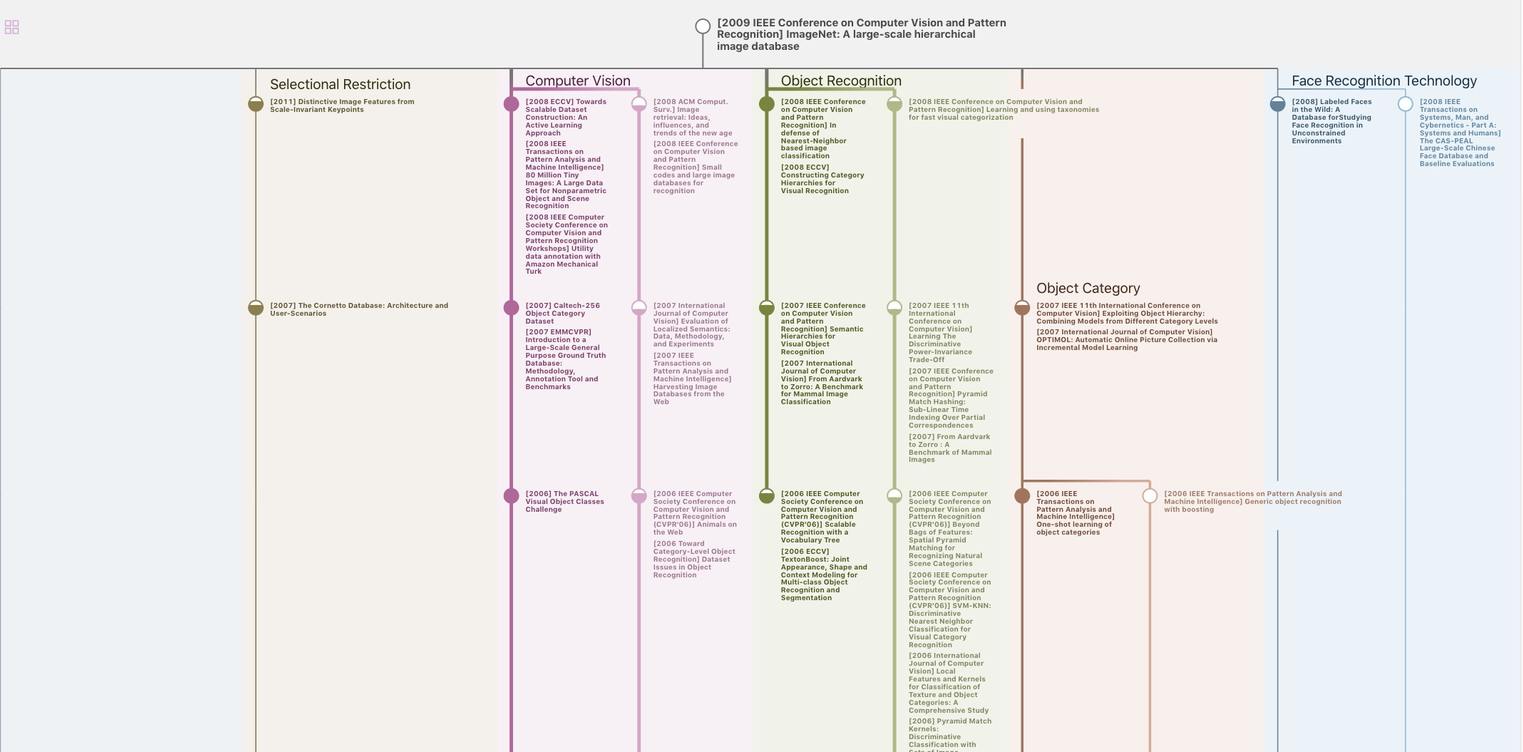
生成溯源树,研究论文发展脉络
Chat Paper
正在生成论文摘要