Mobility-Assisted Federated Learning for Vehicular Edge Computing.
Asilomar Conference on Signals, Systems and Computers(2023)
摘要
This paper addresses the unique challenges of machine learning in smart public transportation systems, where data processing and model training are decentralized across smart buses. Traditional federated learning (FL) algorithms, mostly synchronous, struggle in this environment due to asynchronous communication patterns and limited Roadside Units (RSUs) coverage. To overcome these challenges, we introduce MARLIN (Mobility Assisted fedeRated LearnINg), an innovative asynchronous FL approach that utilizes the predictable movement of buses and the potential for Vehicle-to-Vehicle (V2V) communication. MARLIN employs buses as relays for server communication, enhancing interaction frequency and expediting FL convergence. Our experiments on the FMNIST dataset demonstrate that MARLIN significantly outperforms the existing asynchronous FL method [1], offering a viable solution for efficient data processing in intelligent public transportation systems.
更多查看译文
关键词
Edge Computing,Federated Learning,Vehicular Edge Computing,Data Processing,Machine Learning,Public System,Intelligent Systems,Communication Patterns,Intelligent Transportation,Smart Systems,Intelligent Transportation Systems,Public Transport System,Roadside Units,Smart Transportation,Learning Rate,Convergence Rate,Global Model,Time Slot,Updated Model,Local Updates,Intelligent Vehicles,Search Interval,Training Round,Indirect Communication
AI 理解论文
溯源树
样例
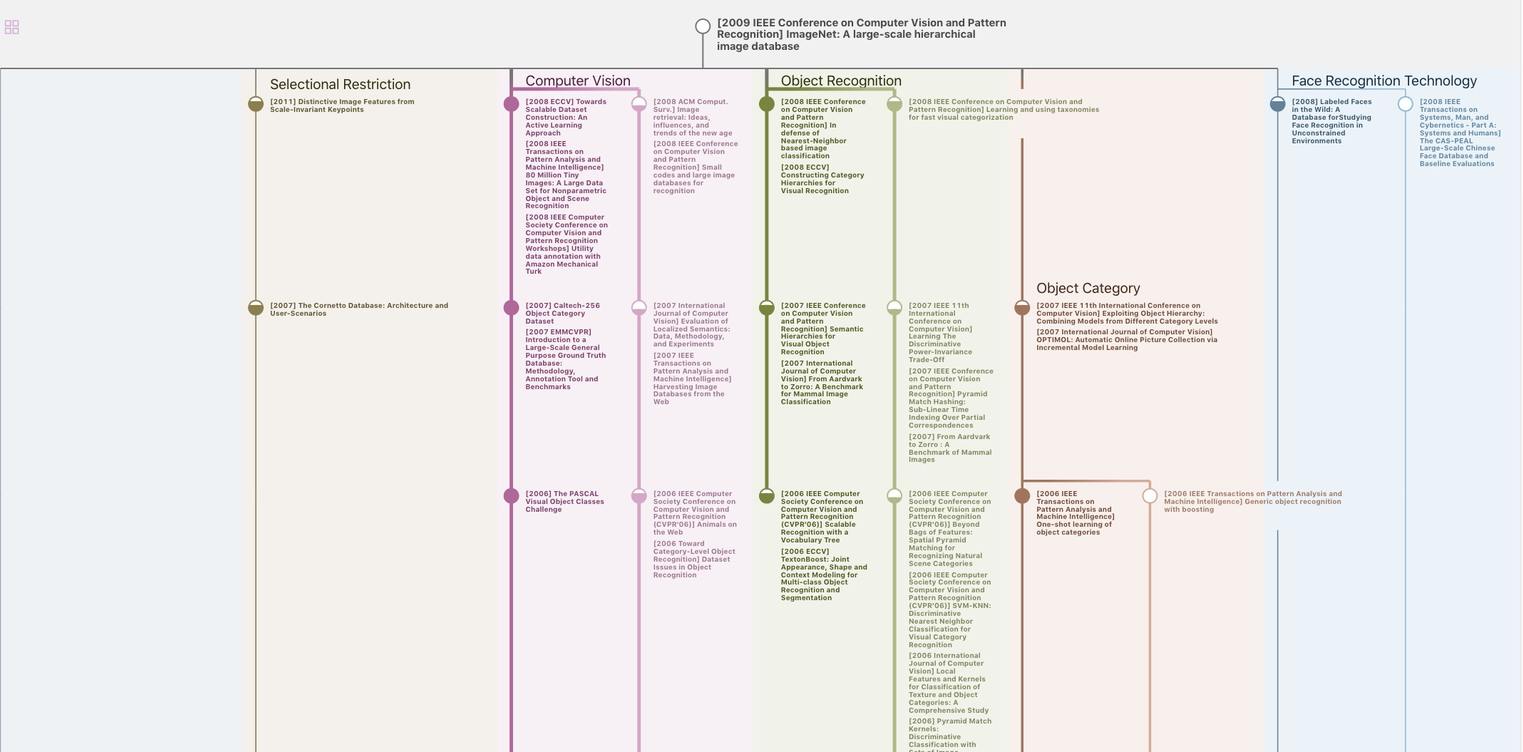
生成溯源树,研究论文发展脉络
Chat Paper
正在生成论文摘要