Lithium-ion battery SOH prediction based on VMD-PE and improved DBO optimized temporal convolutional network model
Journal of Energy Storage(2024)
摘要
Accurately estimating the state of health (SOH) in lithium-ion batteries is crucial for optimizing performance, cost management, enhancing safety, extending lifespan, and promoting environmental sustainability. However, due to factors such as capacity regeneration, battery capacity degradation data exhibits nonlinear and non-stationary characteristics, making it challenging to accurately predict SOH. In order to improve prediction accuracy, this paper proposes a novel SOH prediction model based on VMD-PE-IDBO-TCN. Firstly, the variational mode decomposition-permutation entropy (VMD-PE) method is innovatively introduced to decompose the original battery SOH data and reorganize it into a series of subsequences, reducing the impact of non-stationary components on the prediction results. Moreover, temporal convolutional network (TCN) prediction models are used for each subsequence, and an improved dung beetle optimization (IDBO) algorithm is used to optimize the TCN parameters, where this IDBO algorithm incorporates SPM chaotic mapping, golden sine strategy, and adaptive Gaussian-Cauchy mixed mutation perturbation. The IDBO algorithm rapidly and accurately identifies the optimal parameter combination, significantly enhancing the predictive performance of the TCN model. Finally, the predicted values of each subsequence are superimposed to obtain the final prediction result. Two different lithium-ion battery datasets from NASA and CALCE are used for experimental validation. Experimental results show that the proposed model has higher prediction accuracy, with a maximum RMSE of only 0.0072 and a maximum MAPE of only 0.67 %, and has good adaptability to different types of batteries and batteries operating at high temperatures.
更多查看译文
关键词
Lithium-ion batteries,State of health,Temporal convolutional network,Improved dung beetle optimization,Variational modal decomposition,Permutation entropy
AI 理解论文
溯源树
样例
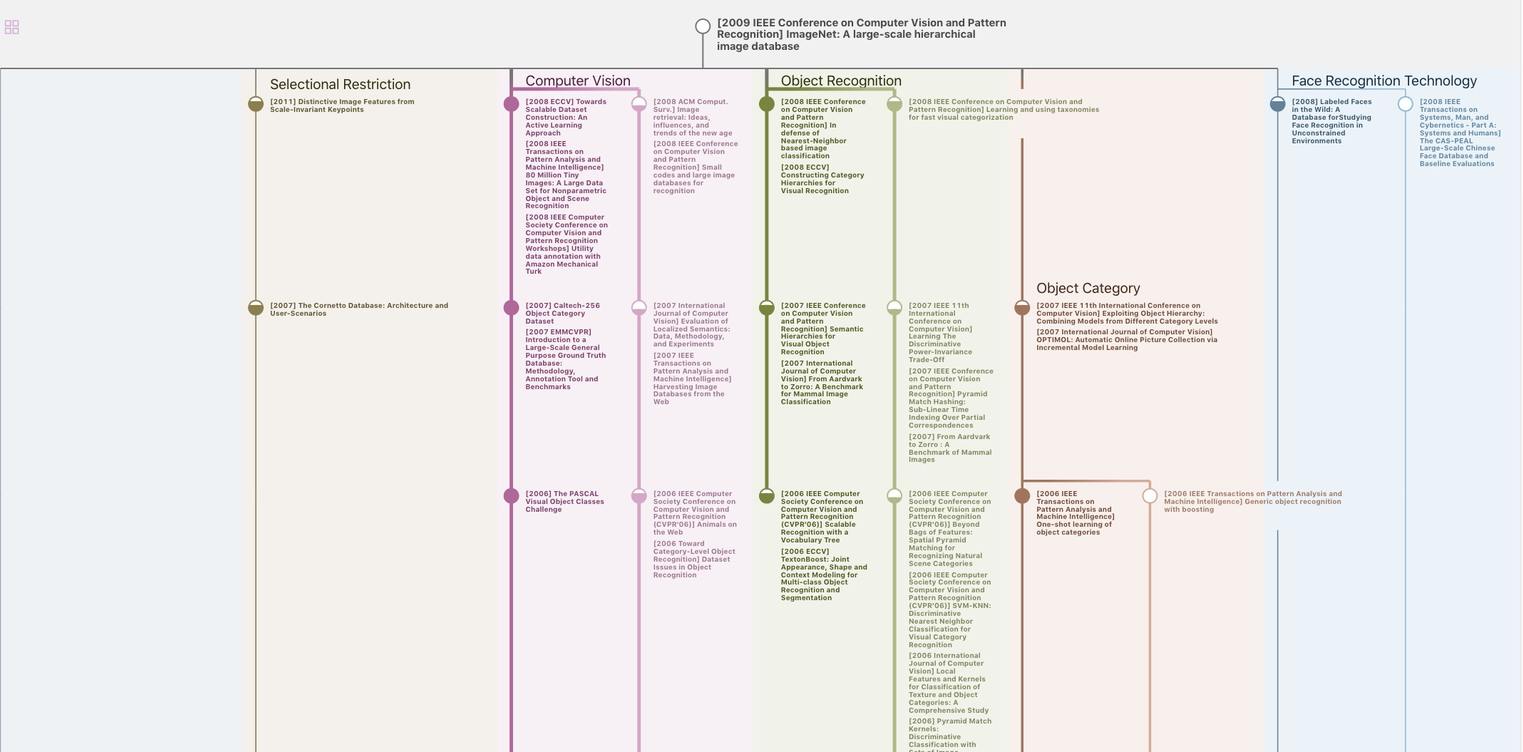
生成溯源树,研究论文发展脉络
Chat Paper
正在生成论文摘要