Landslide Susceptibility Zoning: Integrating Multiple Intelligent Models with SHAP Analysis
Journal of Science and Transport Technology(2024)
摘要
In this study, we aim to delineate landslide susceptibility zones within Dien Bien province, Vietnam, leveraging the capabilities of various machine learning models including Light Gradient Boosting Machine (LGBM), K-Nearest Neighbors (KNN), and Gradient Boosting (GB). Harnessing a dataset comprising 665 data points and encompassing 14 influential factors such as slope, aspect, curvature, elevation, geological composition, Normalized Difference Vegetation Index (NDVI), and proximity to geological features like faults, rivers, and roads, a comprehensive database for landslide modeling was constructed. The analysis entailed rigorous evaluation and comparison of model accuracy employing established statistical metrics, notably Receiver Operating Characteristic (ROC) curves and Area Under the Curve (AUC). The findings underscore the efficacy of the Light Gradient Boosting Machine model, exhibiting superior performance with an AUC score of 0.85, surpassing both the Gradient Boosting model (AUC = 0.81) and the K-Nearest Neighbors model (AUC = 0.79). Notably, the Light Gradient Boosting Machine model emerges as a promising tool for precise landslide prediction within the study area, offering significant potential for the creation of a robust landslide susceptibility map. The resulting spatial forecast map for Dien Bien province holds considerable utility for informing land use planning initiatives aimed at mitigating the impact of landslide disasters in the region. Moreover, the application of SHAP (Shapley Additive explanation) values to quantify the contribution of each factor to landslide susceptibility prediction, offering novel insights into model interpretation and feature importance. The resulting spatial forecast map holds significant implications for land use planning and disaster mitigation efforts in Dien Bien province, showcasing the potential of advanced machine learning techniques in enhancing landslide risk management strategies.
更多查看译文
AI 理解论文
溯源树
样例
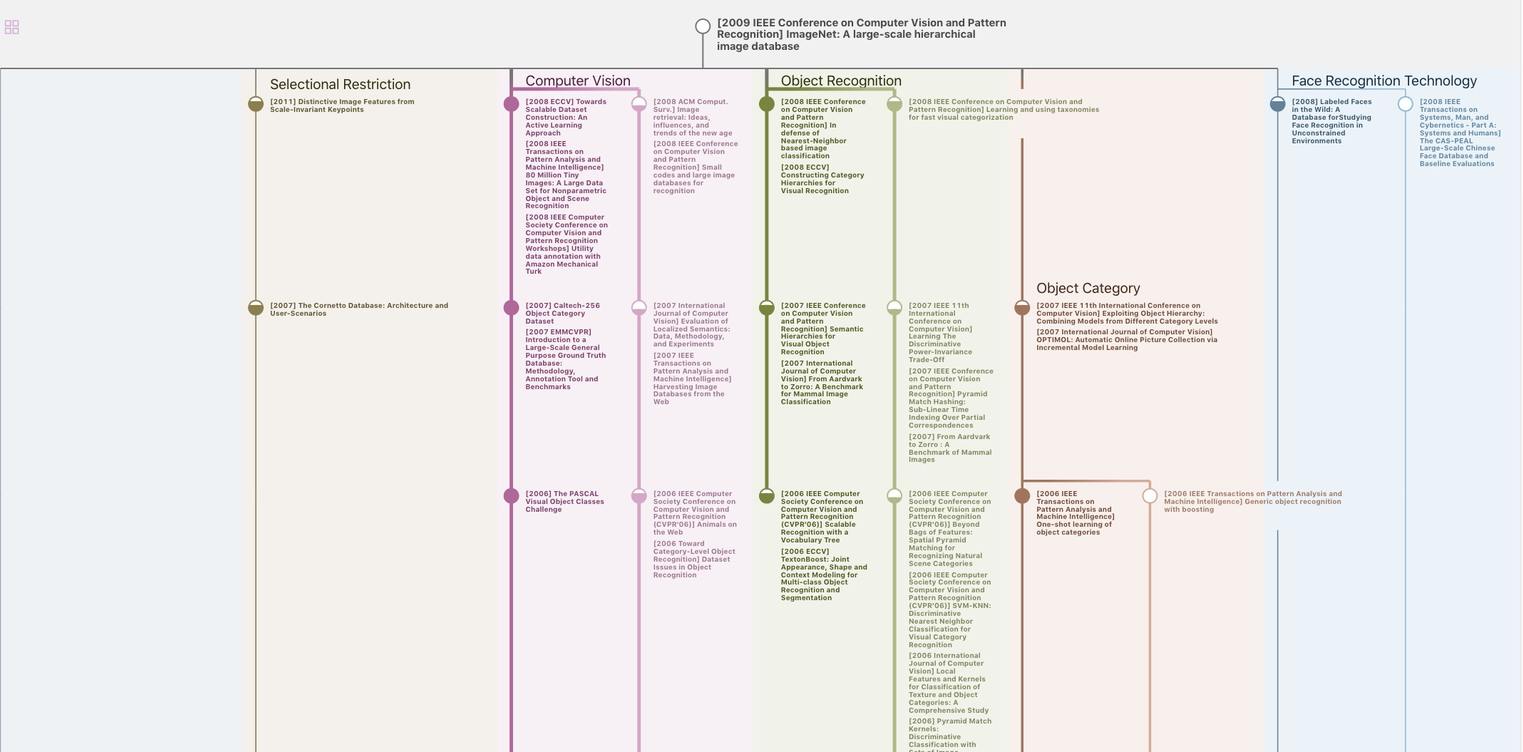
生成溯源树,研究论文发展脉络
Chat Paper
正在生成论文摘要