Prediction of Large-Scale Regional Evapotranspiration Based on Multi-Scale Feature Extraction and Multi-Headed Self-Attention
REMOTE SENSING(2024)
摘要
Accurately predicting actual evapotranspiration (ETa) at the regional scale is crucial for efficient water resource allocation and management. While previous studies mainly focused on predicting site-scale ETa, in-depth studies on regional-scale ETa are relatively scarce. This study aims to address this issue by proposing a MulSA-ConvLSTM model, which combines the multi-headed self-attention module with the Pyramidally Attended Feature Extraction (PAFE) method. By extracting feature information and spatial dependencies in various dimensions and scales, the model utilizes remote sensing data from ERA5-Land and TerraClimate to attain regional-scale ETa prediction in Shandong, China. The MulSA-ConvLSTM model enhances the efficiency of capturing the trend of ETa successfully, and the prediction results are more accurate than those of the other contrast models. The Pearson's correlation coefficient between observed and predicted values reaches 0.908. The study has demonstrated that MulSA-ConvLSTM yields superior performance in forecasting various ETa scenarios and is more responsive to climatic changes than other contrast models. By using a convolutional network feature extraction method, the PAFE method extracts global features via various convolutional kernels. The customized MulSAM module allows the model to concentrate on data from distinct subspaces, focusing on feature changes in multiple directions. The block-based training method is employed for the large-scale regional ETa prediction, proving to be effective in mitigating the constraints posed by limited hardware resources. This research provides a novel and effective method for accurately predicting regional-scale ETa.
更多查看译文
关键词
multi-headed self-attention,actual evapotranspiration (ETa) prediction,large regional scale,climate change
AI 理解论文
溯源树
样例
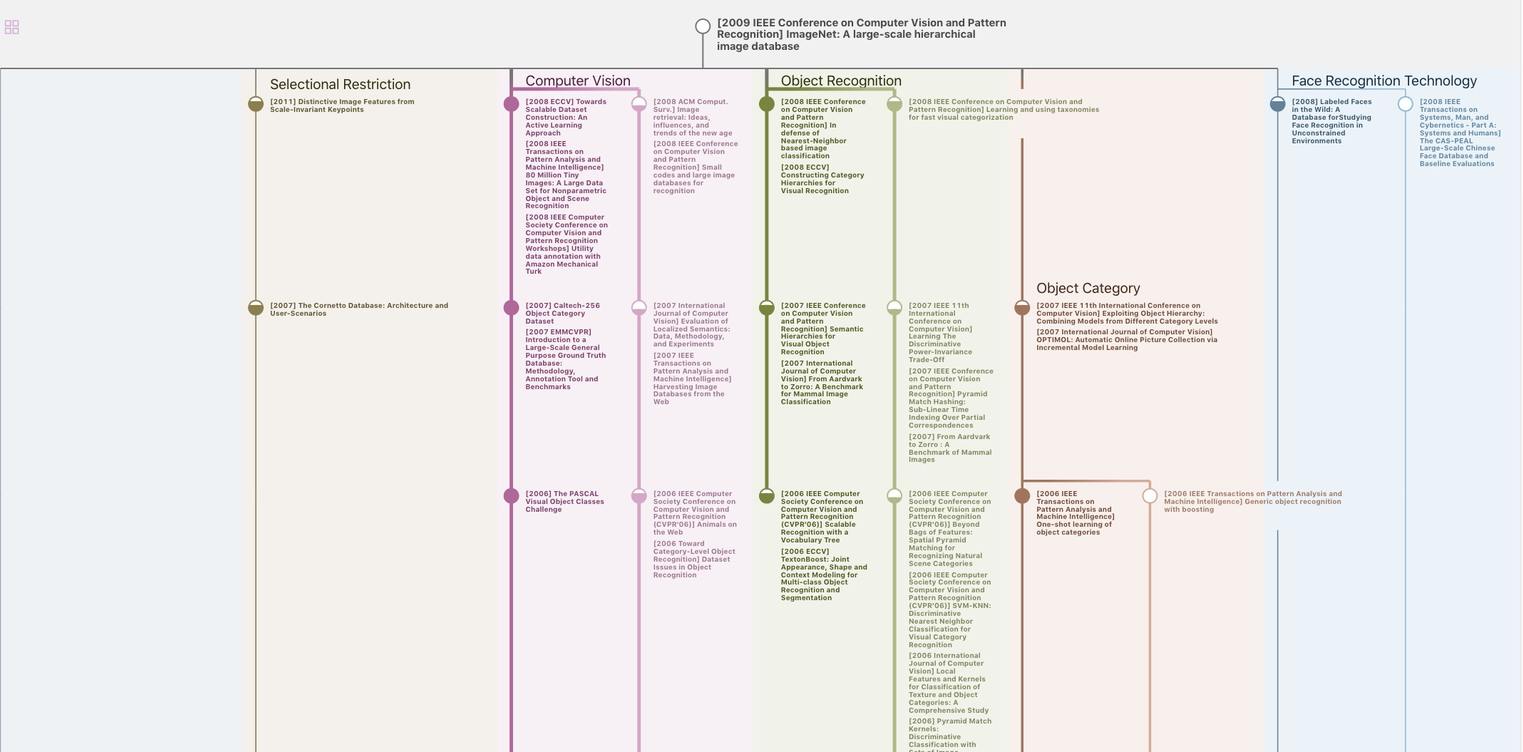
生成溯源树,研究论文发展脉络
Chat Paper
正在生成论文摘要