Exploring DeepDream and XAI Representations for Classifying Histological Images
SN Computer Science(2024)
摘要
The application of machine learning methods to analyze histological images has led to significant advances in computer-aided diagnosis. In this work, a study was carried out to classify patterns present in histological samples stained with hematoxylin-eosin, representative of breast cancer, colorectal cancer, liver tissue, and oral dysplasia. The proposed method explored convolutional neural networks (VGG19, ResNet50, InceptionV3, and EfficientNet B2), gradient-weighted class activation mapping, local interpretable model-agnostic explanations and representations based on the inceptionism effect (DeepDream). The deep features were analyzed via multiple classifiers (rotation forest, multilayer perceptron, logistic, random forest, decorate, k-nearest neighbor, KStar, and support vector machine) to indicate the main associations. The best solution was obtained through the ResNet50 model with the representations from the VGG19 architecture, exploring feature vectors with 40 and 100 attributes. The results were AUC rates ranging from 0.994 (breast cancer dataset) to 1.0 (colorectal dataset). The most explored deep features were obtained via original images (highest occurrence), followed by local interpretable model-agnostic explanations, DeepDream, and gradient-weighted class activation mapping representations. These results can support the development of techniques addressed to pattern recognition of H E images with more robust computer-aided diagnoses.
更多查看译文
关键词
Deep features,DeepDream representations,XAI,Histological images
AI 理解论文
溯源树
样例
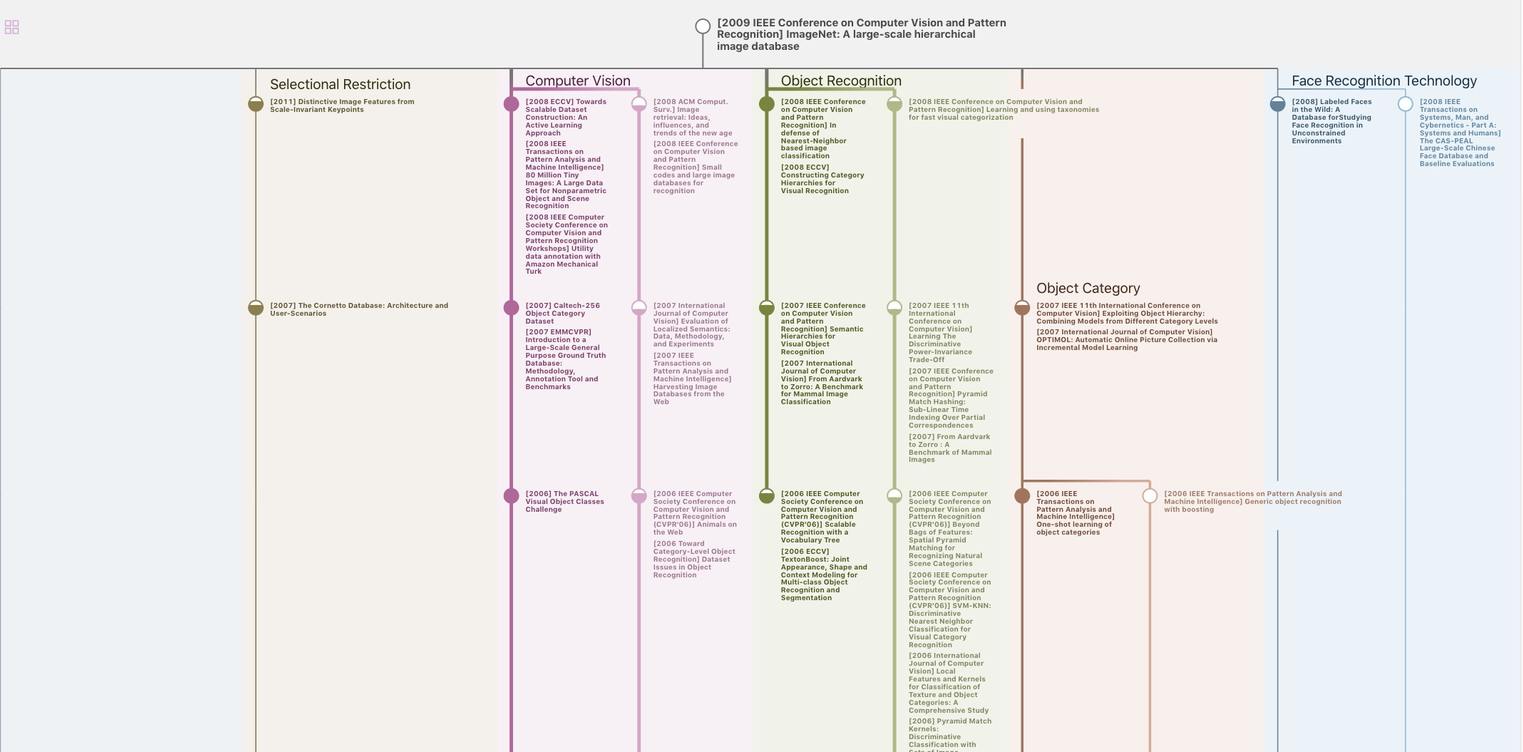
生成溯源树,研究论文发展脉络
Chat Paper
正在生成论文摘要