An Adaptive Compression and Communication Framework for Wireless Federated Learning
IEEE Transactions on Mobile Computing(2024)
摘要
Federated learning (FL) is a distributed privacy-preserving paradigm of machine learning that enables efficient and secure model training through the collaboration of multiple clients. However, imperfect channel estimation and resource constraints of edge devices severely hinder the convergence of typical wireless FL, while the trade-off between communications and computation still lacks in-depth exploration. These factors lead to inefficient communications and hinder the full potential of FL from being unleashed. In this regard, we formulate a joint optimization problem of communications and learning in wireless networks subject to dynamic channel variations. For addressing the formulated problem, we propose an integrated adaptive
$n$
-ary compression and resource management framework (ANC) that is capable of adjusting the selection of edge devices and compression schemes, and allocates the optimal resource blocks and transmit power to each participating device, which effectively improves the energy efficiency and scalability of FL in resource-constrained environments. Furthermore, an upper bound on the expected global convergence rate is derived in this paper to quantify the impacts of transmitted data volume and wireless propagation on the convergence of FL. Simulation results demonstrate that the proposed adaptive framework achieves much faster convergence while maintaining considerably low communication overhead.
更多查看译文
关键词
Federated learning,communication-computing trade-off,distributed machine learning,joint optimization,model compression
AI 理解论文
溯源树
样例
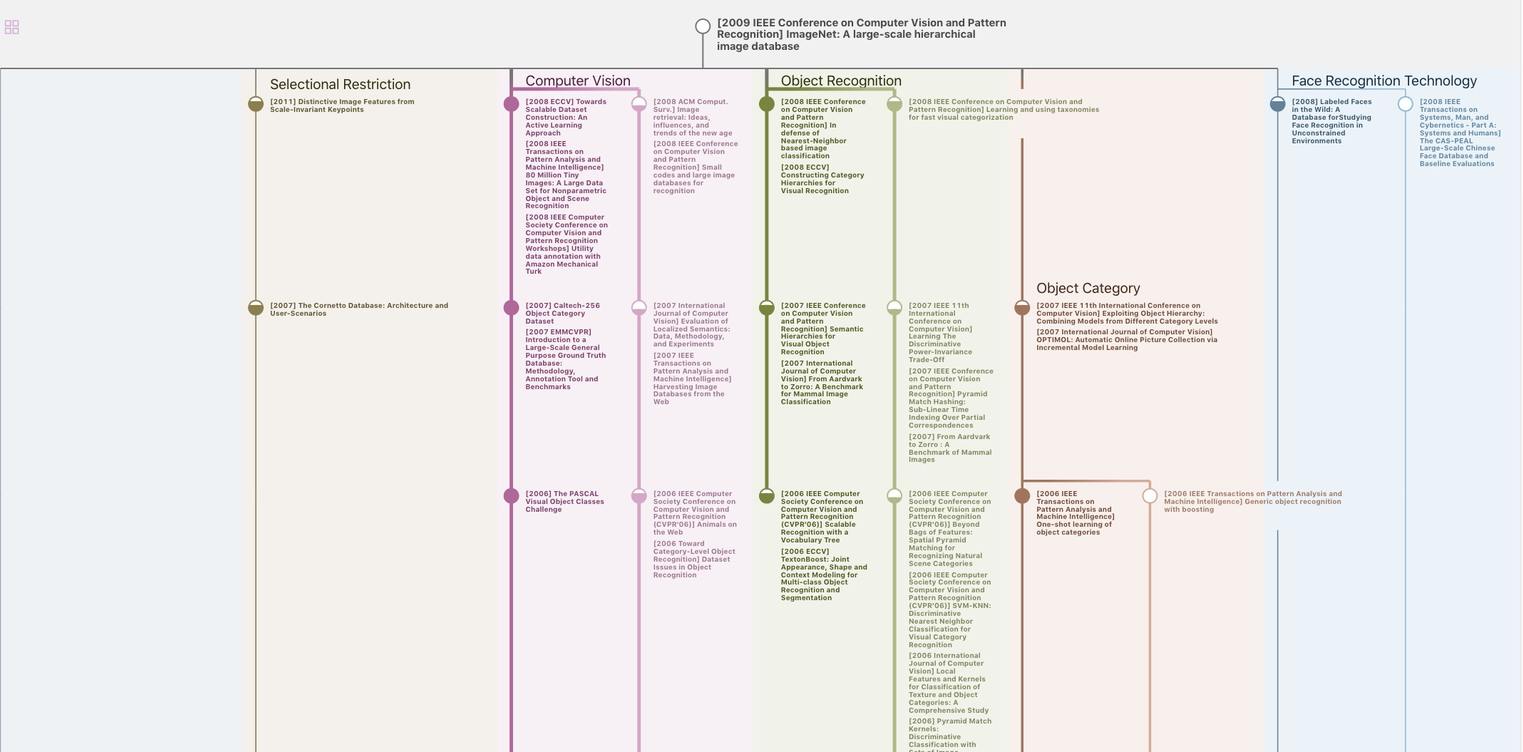
生成溯源树,研究论文发展脉络
Chat Paper
正在生成论文摘要