An efficient training method to learn a model of turbulence
The European Physical Journal Plus(2024)
摘要
Turbulence is the paradigm of complex systems, notably for its non-equilibrium multi-scale character. Since it is rarely possible to have access to direct simulations of turbulence, the development of efficient models is crucial. The use of machine learning tools has recently received ample attention, yet learning such a complex system is a challenging task. In the present work, we explore the limitations of machine learning in describing high-order statistics, which are essential for understanding turbulence structure. We will focus on shell models of turbulence. They have a manageable dimension and display the same characteristics as Navier–Stokes turbulence, such as Kolmogorov scaling and intermittency. We aim to develop a robust model using machine learning strategies, focusing on analysing recurrent and multi-layer networks. We have found that the standard protocol for training a recurrent neural network is not satisfactory for the description of rare events. The standard training approach creates a discrepancy between what the model learns and what the model has to be able to do. We propose a new training method called Forecast Training. Our extensive statistical analysis shows that this method significantly improves performance. We can train a model that gives excellent agreement with the actual truth, even with limited training data.
更多查看译文
AI 理解论文
溯源树
样例
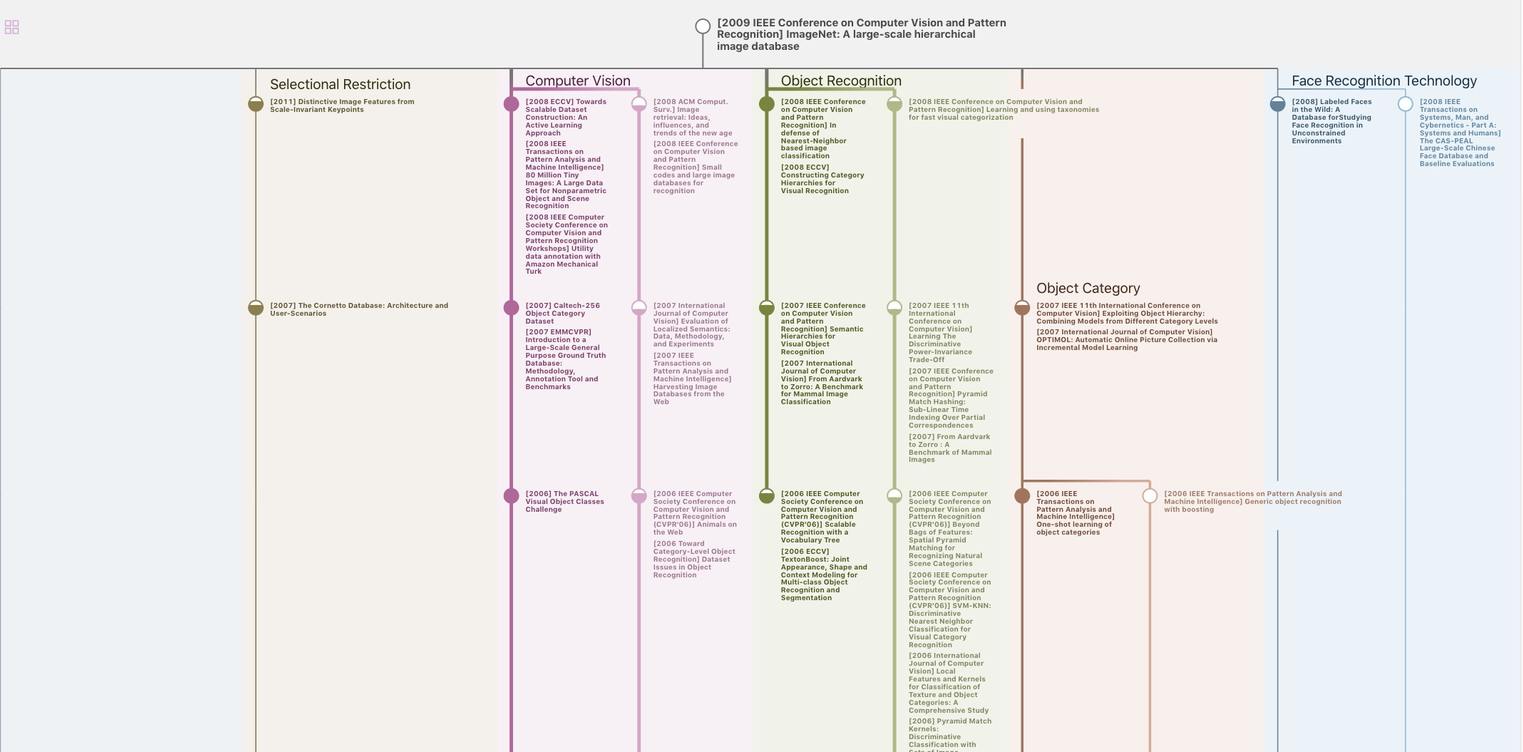
生成溯源树,研究论文发展脉络
Chat Paper
正在生成论文摘要