Neural Preassigned Performance Control for State-Constrained Nonlinear Systems Subject to Disturbances.
IEEE transactions on neural networks and learning systems(2024)
摘要
This article addresses the finite-time neural predefined performance control (PPC) issue for state-constrained nonlinear systems (NSs) with exogenous disturbances. By integrating the predefined-time performance function (PTPF) and the conventional barrier Lyapunov function (BLF), a new set of time-varying BLFs is designed to constrain the error variables. This establishes conditions for satisfying full-state constraints while ensuring that the tracking error meets the predefined performance indicators (PPIs) within a predefined time. Additionally, the incorporation of the nonlinear disturbance observer technique (NDOT) in the control design significantly enhances the ability of the system to reject disturbances and improves overall robustness. Leveraging recursive design based on dynamic surface control (DSC), a finite-time neural adaptive PPC strategy is devised to ensure that the closed-loop system is semi-globally practically finite-time stable (SPFS) and achieves the desired PPIs. Finally, the simulation results of two practical examples validate the efficacy and viability of the proposed approach.
更多查看译文
关键词
Barrier Lyapunov function (BLF),finite-time control,nonlinear disturbance observer,predefined performance control (PPC),state-constrained system
AI 理解论文
溯源树
样例
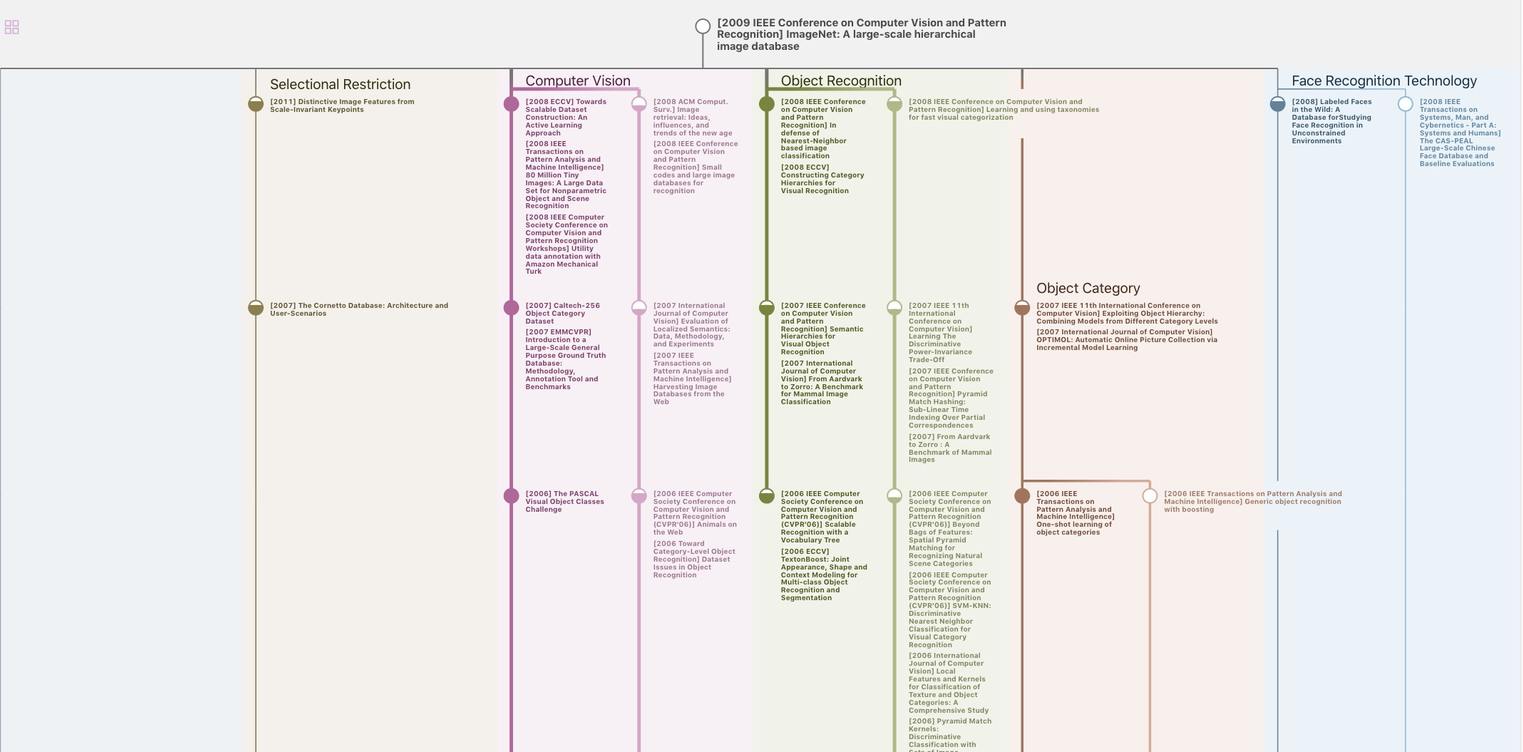
生成溯源树,研究论文发展脉络
Chat Paper
正在生成论文摘要