Personalized Early Warning of Learning Performance for College Students: A Multi-level Approach via Cognitive Ability and Learning State Modeling
IEEE Transactions on Learning Technologies(2024)
摘要
To prevent students from learning risks and improve teachers' teaching quality, it is of great significance to provide accurate early warning of learning performance to students by analyzing their interactions through an e-learning system. In existing research, the correlations between learning risks and students' changing cognitive abilities or learning states are still under-explored, and the personalized early warning are unavailable for students at different levels. To accurately identify the possible learning risks faced by students at different levels, this paper proposes a personalized early warning approach to learning performance for college students via cognitive ability and learning state modeling. In this approach, students' learning process data and historical performance data are analyzed to track students' cognitive abilities in the whole learning process, and model their learning states from four dimensions, i.e., learning quality, learning engagement, latent learning state, and historical learning state. Then, the Adaboost algorithm is used to predict students' learning performance, and an evaluation rule with five levels is designed to dynamically provide multi-level personalized early warning to students. Finally, the comparative experiments based on real-world datasets demonstrate that the proposed approach could effectively predict all students' learning performance, and provide accurate early warning services to them.
更多查看译文
关键词
Cognitive ability modeling,learning performance prediction,learning state modeling,multi-level early warning,personalized early warning
AI 理解论文
溯源树
样例
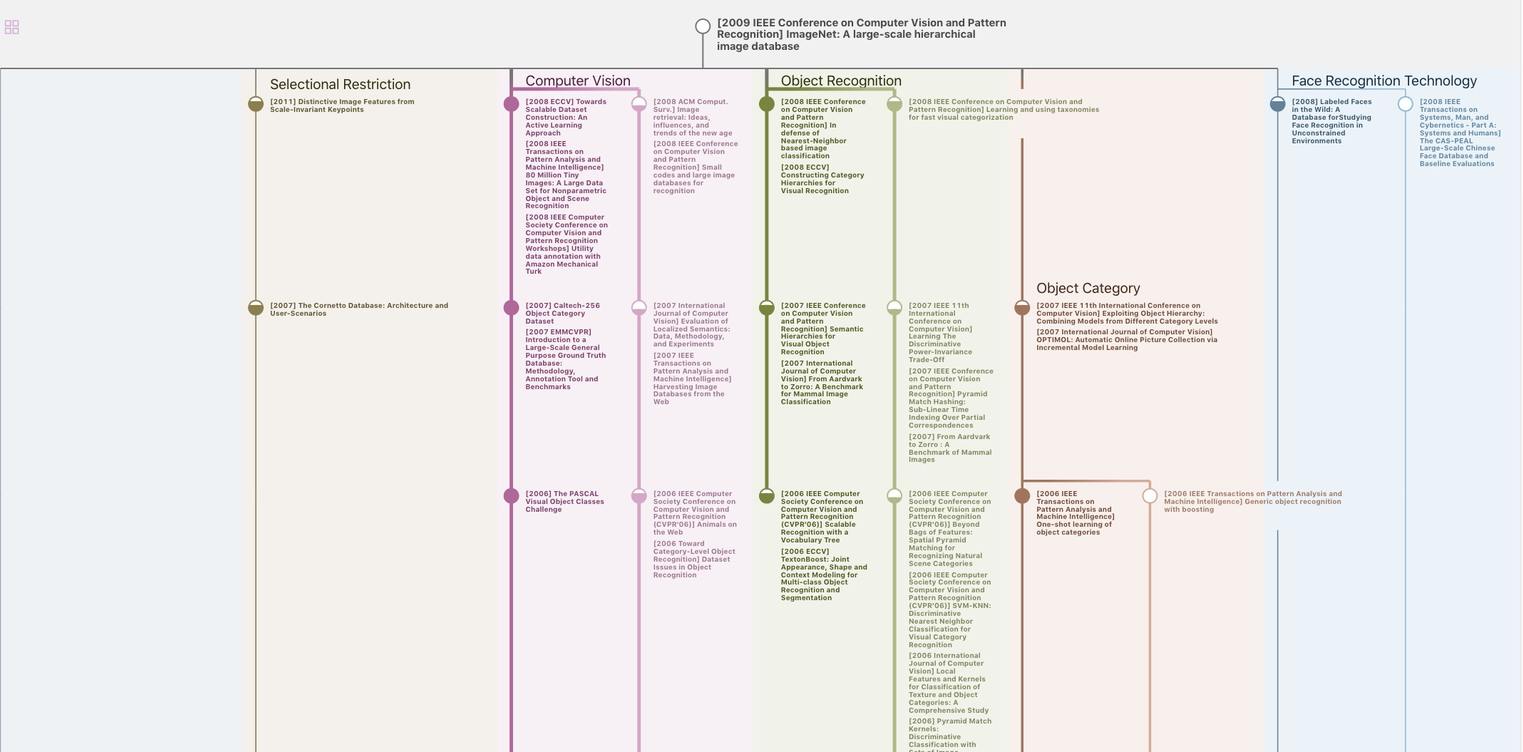
生成溯源树,研究论文发展脉络
Chat Paper
正在生成论文摘要