Deep Reinforcement Learning Based Dynamic Flowlet Switching for DCN
IEEE Transactions on Cloud Computing(2024)
摘要
Flowlet switching has been proven to be an effective technology for fine-grained load balancing in data center networks. However, flowlet detection based on static flowlet timeout values, lacks accuracy and effectiveness in complex network environments. In this paper, we propose a new deep reinforcement learning approach, called DRLet, to dynamically detect flowlets. DRLet offers two advantages: first, it provides dynamic flowlet timeout values to detect bursts into fine-grained flowlets; second, flowlet timeout values are automatically configured by the deep reinforcement learning agent, which only requires simple and measurable network states, instead of any prior knowledge, to achieve the pre-defined goal. With our approach, the flowlet timeout value dynamically matches the network load scenario, ensuring the accuracy and effectiveness of flowlet detection while suppressing packet reordering. Our results show that DRLet achieves superior performance compared to existing schemes based on static flowlet timeout values in both baseline and asymmetric topologies.
更多查看译文
关键词
Flowlet,deep reinforcement learning,load balancing,data center networks
AI 理解论文
溯源树
样例
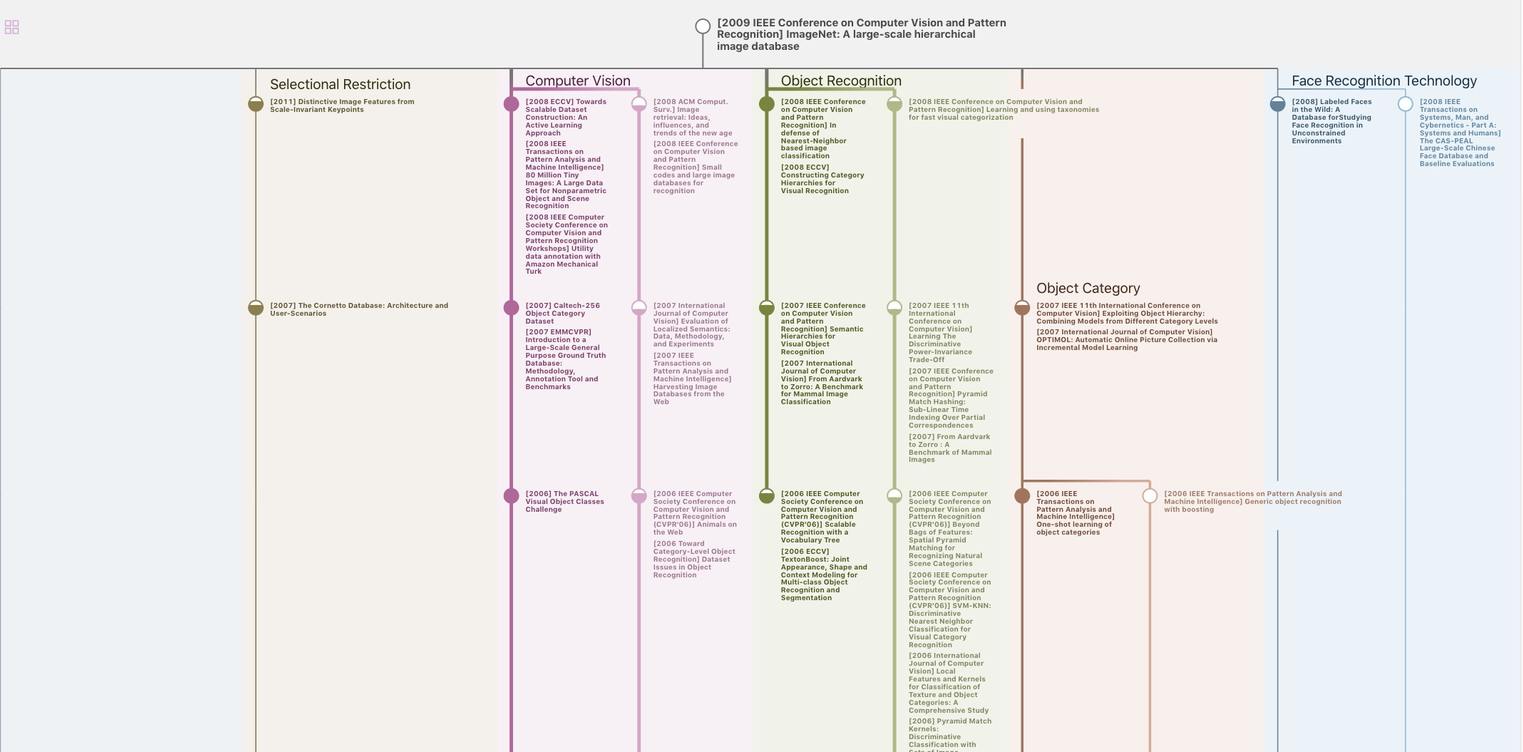
生成溯源树,研究论文发展脉络
Chat Paper
正在生成论文摘要