Assessment of Adversarial Attacks on Traffic Sign Detection for Connected and Autonomous Vehicles.
IEEE International Workshop on Computer Aided Modeling and Design of Communication Links and Networks(2023)
摘要
This paper highlights the vulnerability of Connected and Autonomous Vehicles’ (CAVs) traffic sign detection systems to adversarial attacks - subtle manipulations that misguide machine learning algorithms and pose safety hazards. Particularly, white-box attacks, with full access to the model’s structure, are concerning. To combat this, the paper proposes a resilient neural network using a Bit-Plane segregation system. This mechanism dissects images into bits, removing the compromised parts, and thereby preserving the model’s accuracy. This defense approach requires multiple models trained for a voting-based robust defense. The system comprises a deep neural network for traffic sign detection, adversarial attack modules, a defensive framework, and a voting mechanism. The conducted experiments underline the proposed defense mechanism’s effectiveness in substantially restoring the accuracy compromised due to adversarial attacks.
更多查看译文
关键词
Adversarial attacks,Traffic sign detection,Deep learning,Bit-Plane segregation,CAVs
AI 理解论文
溯源树
样例
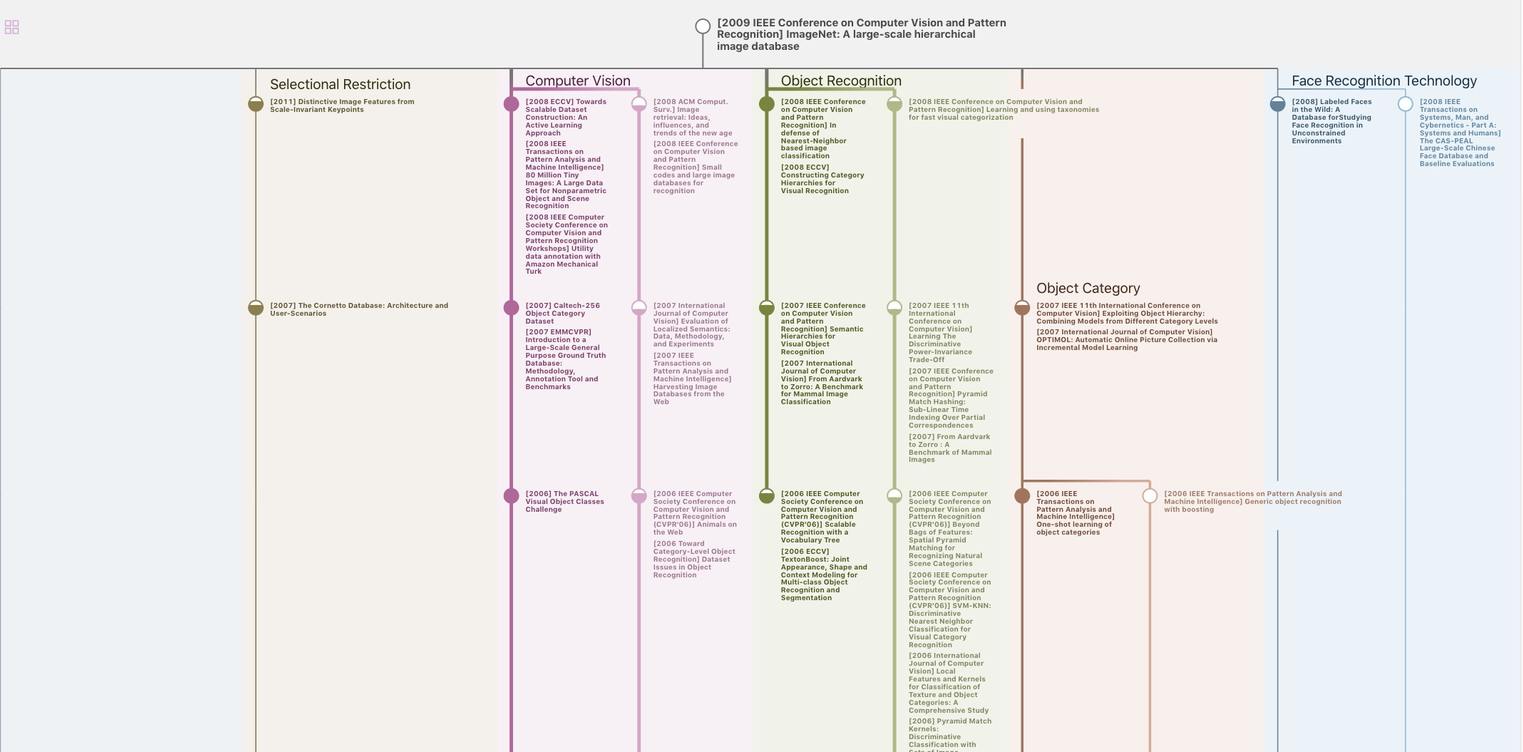
生成溯源树,研究论文发展脉络
Chat Paper
正在生成论文摘要