Spectral mixture analysis for weed traits identification under varying resolutions and growth stages
Computers and Electronics in Agriculture(2024)
摘要
Spectral and spatial resolutions are critical factors in Site-Specific Weed Management (SSWM) applications, which aim to adjust and apply herbicides according to the weed composition and coverage during early growth. Thus, a reliable SSWM requires high spectral and spatial resolution data to create informative maps indicating and spatially localizing the weed species. Besides, upscaling and improving the mapping process are essential to motivate farmers to adopt SSWM practices. However, high-resolution data in spectral and spatial domains is not always available, and mixed pixels are highly probable. Therefore, evaluating approaches that deal with such pixels that pose a significant problem for weed classification tasks is vital. In this regard, Spectral Mixture Analysis (SMA) can exploit subpixel information from coarse-resolution data. However, despite its potential, SMA has not been examined for subpixel weed mapping. In this work, we aim to evaluate the potential advantages of SMA for coverage estimation of different weed traits (weed botanical group, photosynthetic pathway, and species). For this purpose, we first created a dataset containing weed species characterized by different botanical groups and photosynthetic pathways. Then, we evaluated the performance of SMA in estimating the weed’s coverage at varying growth stages and spectral and spatial resolutions. The experimental results revealed that using botanical weed groups as Endmembers (EM) is advantageous over other traits. A quantitative evaluation revealed strong correlation values between the actual and predicted weed coverage and a Mean Absolute Error (MAE) lower than 10 % for the different EM at all resolutions and growth stages. Besides, SMA outperformed the traditional classification-based approach in estimating weed coverage with up to ∼ 30 % lower Mean Absolute Error. Finally, our study establishes a basis for incorporating SMA in various SSWM practices.
更多查看译文
关键词
Hyperspectral Imaging,Site Specific Weed Management,Proximal sensing,Spectral Mixture Analysis,Species Classification
AI 理解论文
溯源树
样例
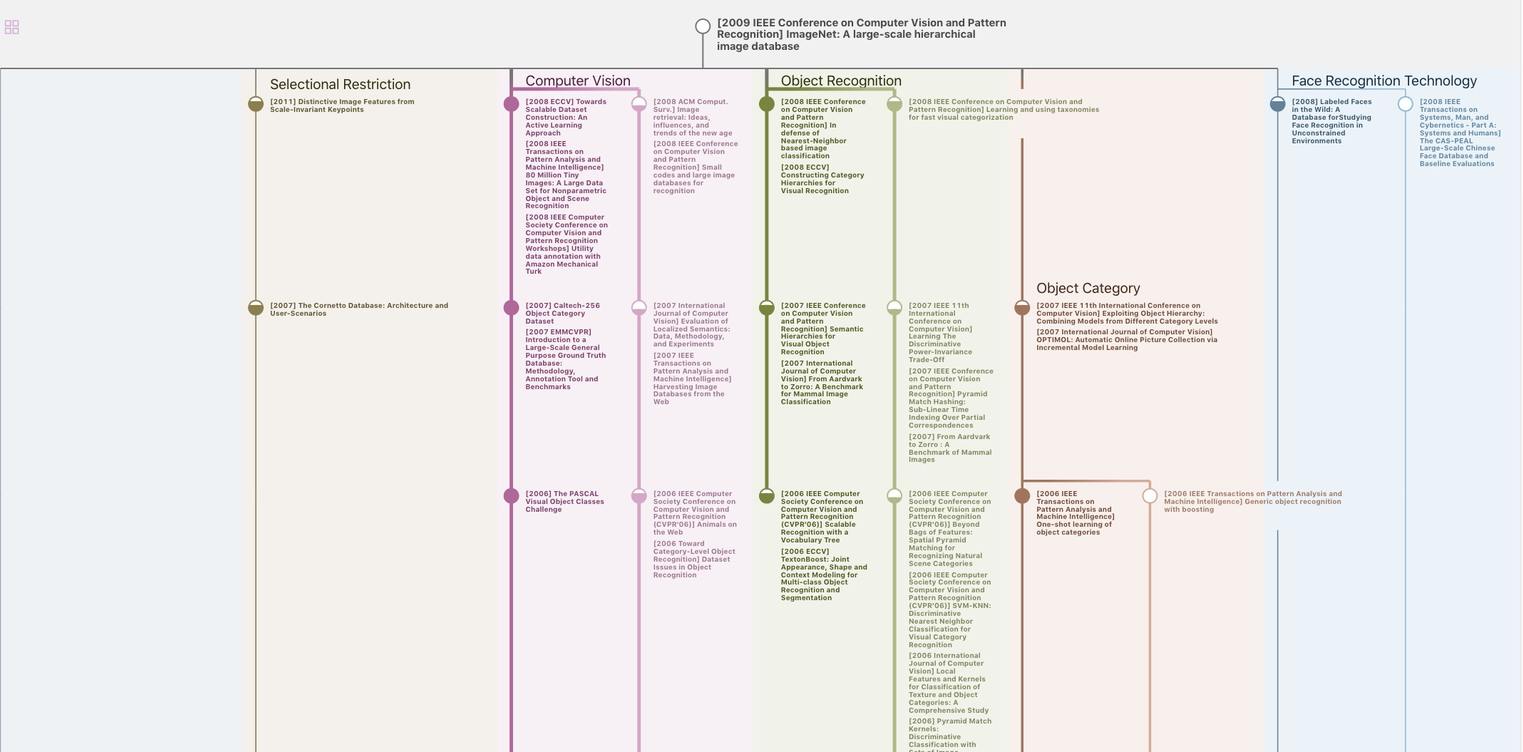
生成溯源树,研究论文发展脉络
Chat Paper
正在生成论文摘要