GDAFormer: Gradient-guided Dual Attention Transformer for Low-Dose CT image denoising
Biomedical Signal Processing and Control(2024)
摘要
Low-Dose Computed Tomography (LDCT) image denoising is an important problem in the research field of CT, as LDCT images exhibit significant noise and artifacts compared to normal-dose CT images. To address this issue, we propose an LDCT image denoising model named Gradient-guided Dual-Attention TransFormer (GDAFormer), which adopts an encoder–decoder structure. Specifically, in the encoding part, the Gradient Denoising Module (GDM) is utilized to obtain the clean gradient map for effectively extracting edge and structure features, and they are fused with LDCT image features by Cross-Attention Transformer (CAT). The decoding part employs a Dual Self-Attention Transformer (DSAT) to capture inter-channel correlations within the fused feature maps and pixel-level correlations within individual channels, thereby improving denoising performance. Experimental results on the MayoLDCT dataset demonstrate that our proposed GDAFormer achieves competitive performance in terms of quantitative metrics and visual perceptual quality.
更多查看译文
关键词
Low-dose CT,Denoising,Gradient-guided,Dual attention transformer
AI 理解论文
溯源树
样例
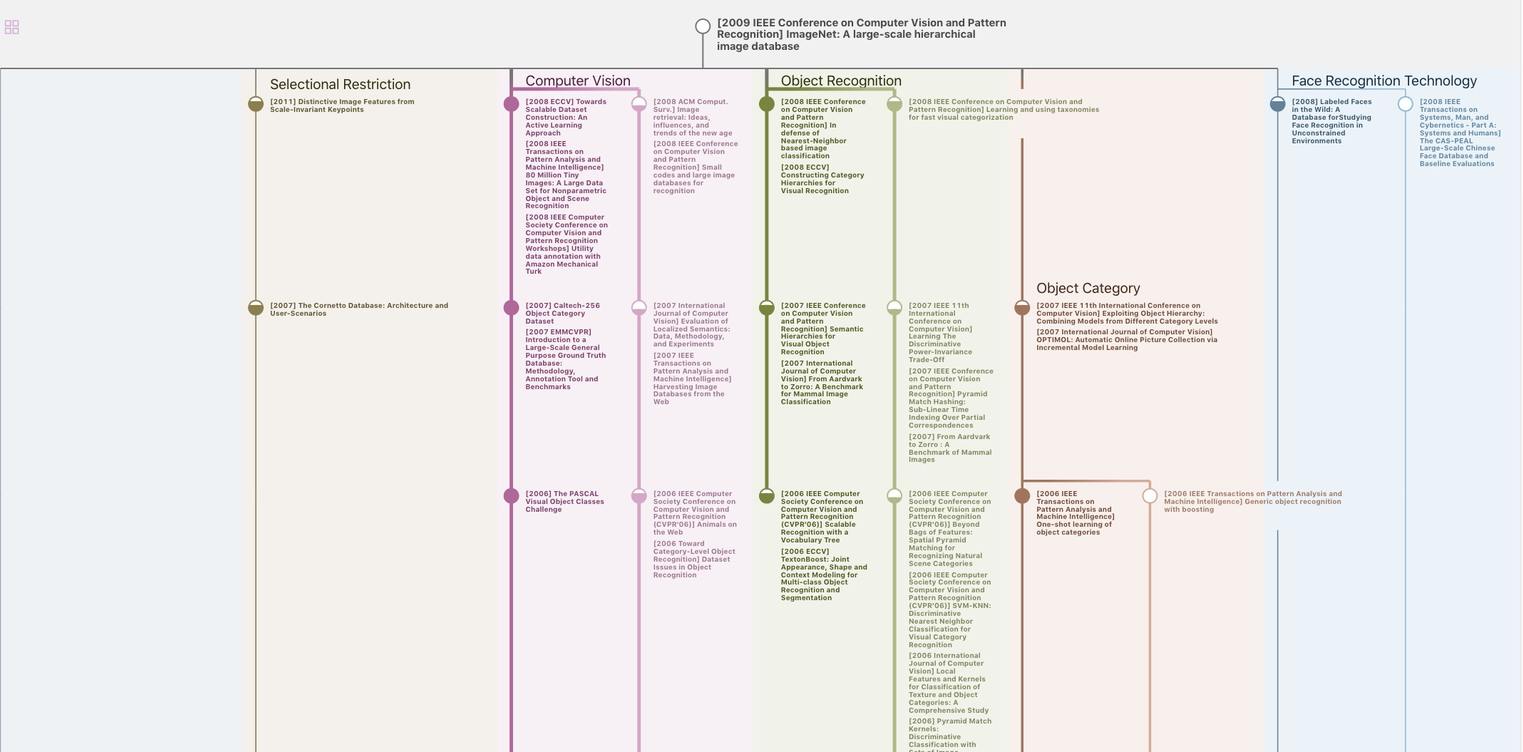
生成溯源树,研究论文发展脉络
Chat Paper
正在生成论文摘要