Dynamic berth allocation under uncertainties based on deep reinforcement learning towards resilient ports
Ocean & Coastal Management(2024)
摘要
With the evolving global trade landscape and the post-pandemic effects, the resilience of ports has become paramount. The unforeseen disturbances bring substantial challenges, especially in berth allocation, a vital task ensuring seamless resilient port operations. The unpredictability of vessel arrivals and the variability in loading/unloading times intensify these issues, pushing traditional static allocation methods beyond their limits. Fortunately, the advent of smart ports has led in an era of big data availability, enabling the application of advanced deep reinforcement learning (DRL) techniques. To capitalize on this shift, this research presents a DRL-based methodology specially designed to solve the berth allocation problem with the uncertainties in vessel arrival and container handling time to enhance port resilience. A Markov Decision Process model (MDP) of the berth allocation problem is established to minimize the mean waiting time with tailored state space, rule-based action space, and reward function to address the issue. An offline training method is designed to train the agent in selecting the optimal action based on the current state of the port berth system at each decision point even in uncertain environments, deep Q-network (DQN) is implemented for this problem. Comprehensive experiments across different problem scales are conducted to validate the effectiveness and generality of the proposed method in solving berth allocation challenges under uncertain conditions. Furthermore, the trained model also performs better than other methods in different vessel congestion levels through learning.
更多查看译文
关键词
Dynamic berth allocation,Uncertainty,Deep reinforcement learning,Resilient port
AI 理解论文
溯源树
样例
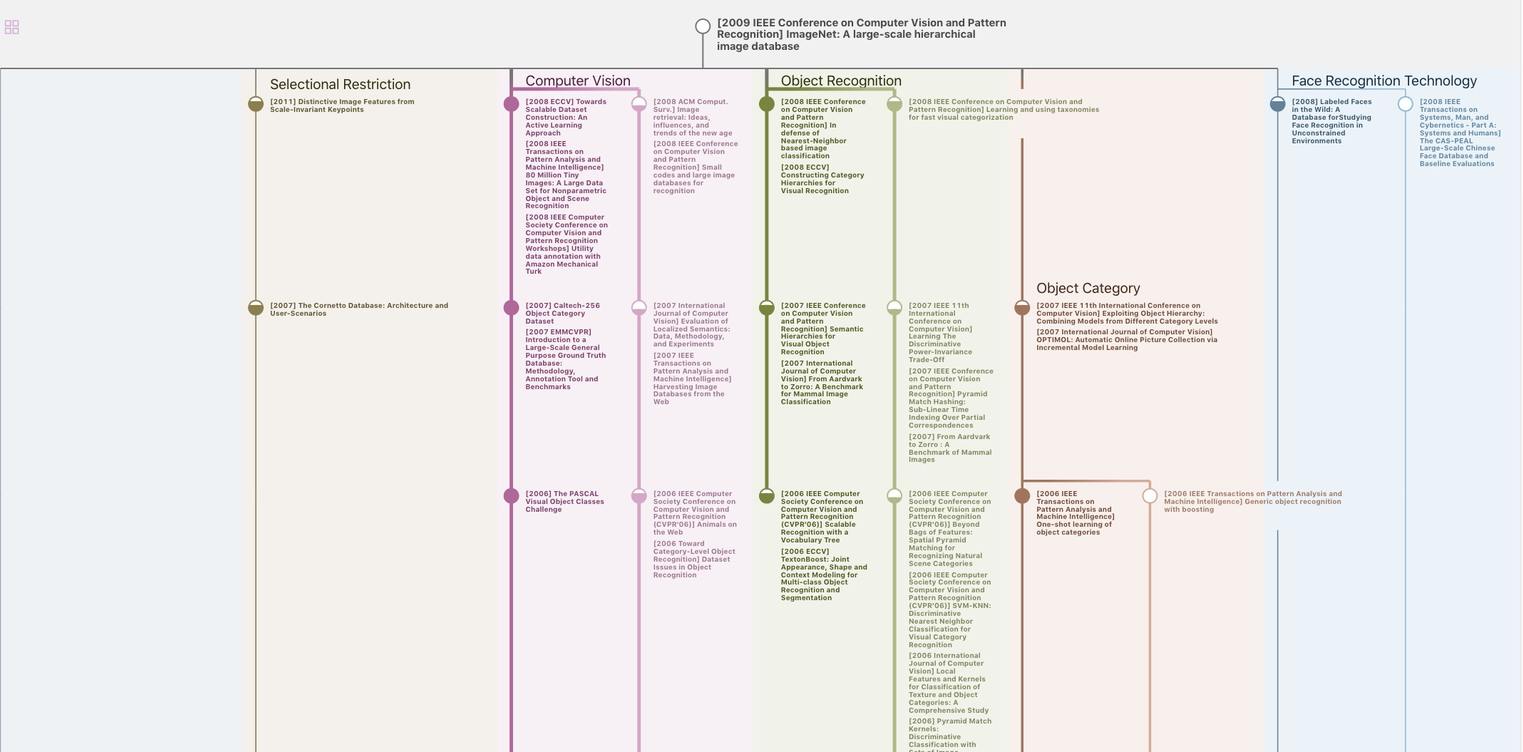
生成溯源树,研究论文发展脉络
Chat Paper
正在生成论文摘要