An Improved VGG-19 Network Induced Enhanced Feature Pooling for Precise Moving Object Detection in Complex Video Scenes
IEEE ACCESS(2024)
摘要
Background subtraction is a crucial stage in many visual surveillance systems. The prime objective of any such system is to detect local changes, and the system could be utilized to face many real-life challenges. Most of the existing methods have addressed the problems of moderate and fast-moving object detection. However, very few literature have addressed the issues of slow moving object detection and these methods need further improvement to enhance the efficacy of detection. Hence, within this article, our significant endeavor involved identifying moving objects in challenging videos through an encoder-decoder architectural design, incorporating an enhanced VGG-19 model alongside a feature pooling framework. The proposed algorithm has various folds of novelties: a pre-trained VGG-19 architecture is modified and is used as an encoder with a transfer learning mechanism. The proposed model learns the weights of the improved VGG-19 model by a transfer-learning mechanism which enhances the model's efficacy. The proposed encoder is designed using a smaller number of layers to extract crucial fine and coarse scale features necessary for detecting the moving objects. The feature pooling framework (FPF) employed is a hybridization of a max-pooling layer, a convolutional layer, and multiple convolutional layers with distinct sampling rates to retain the multi-scale and multi-dimensional features at different scales. The decoder network consists of stacked convolution layers projecting from feature to image space effectively. The developed technique's efficacy is demonstrated against thirty-six state-of-the-art (SOTA) methods. The outcomes acquired by the developed technique are corroborated using subjective as well as objective analysis, which shows superior performance against other SOTA techniques. Additionally, the proposed model demonstrates enhanced accuracy when applied to unseen configurations. Further, the proposed technique (MOD-CVS) attained adequate efficiency for slow, moderate, and fast-moving objects simultaneously.
更多查看译文
关键词
Object detection,Feature extraction,Videos,Surveillance,Visualization,Transfer learning,Lighting,Artificial neural networks,Encoding,Deep neural network,background subtraction,transfer learning,encoder-decoder architecture,feature pooling framework
AI 理解论文
溯源树
样例
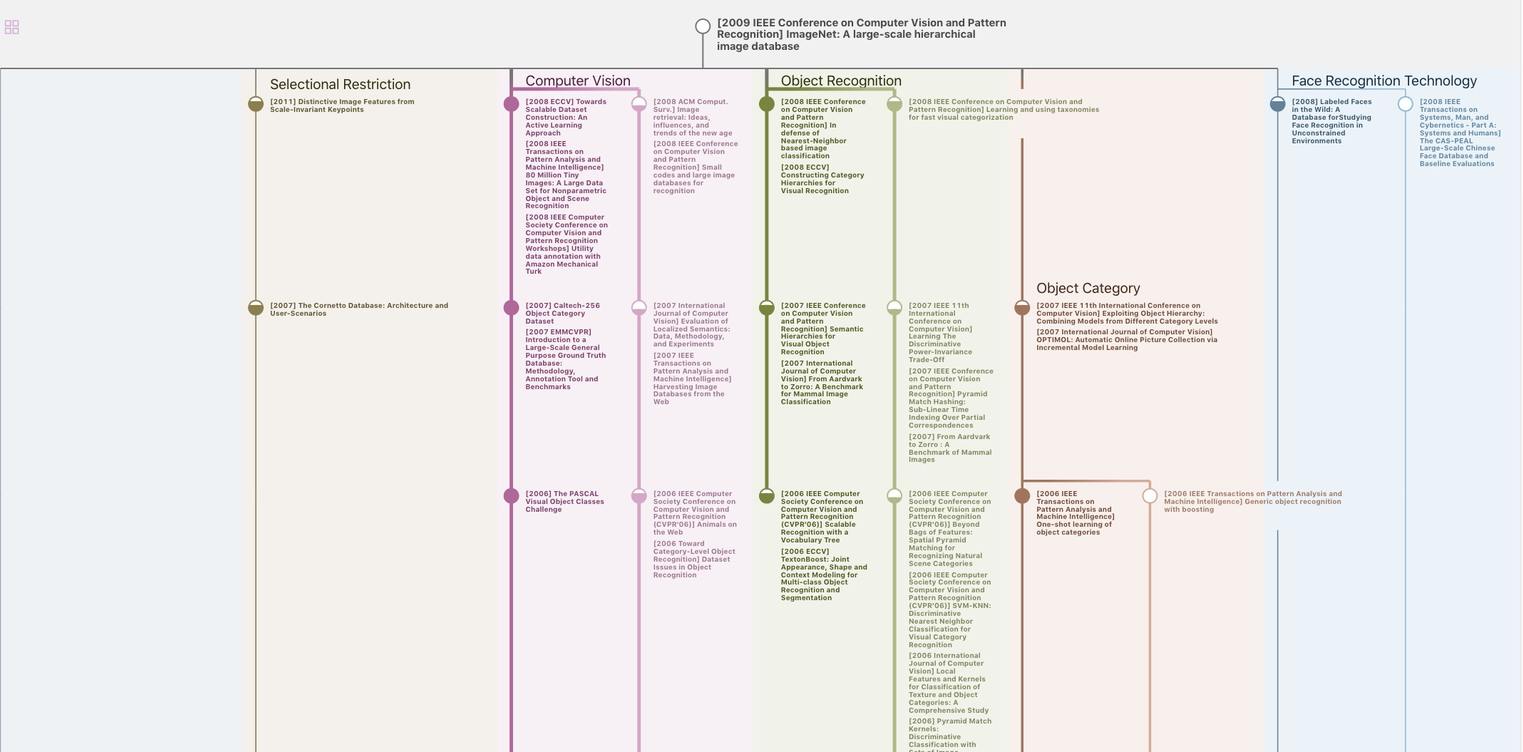
生成溯源树,研究论文发展脉络
Chat Paper
正在生成论文摘要