A novel breast cancer image classification model based on multiscale texture feature analysis and dynamic learning
SCIENTIFIC REPORTS(2024)
摘要
Assistive medical image classifiers can greatly reduce the workload of medical personnel. However, traditional machine learning methods require large amounts of well-labeled data and long learning times to solve medical image classification problems, which can lead to high training costs and poor applicability. To address this problem, a novel unsupervised breast cancer image classification model based on multiscale texture analysis and a dynamic learning strategy for mammograms is proposed in this paper. First, a gray-level cooccurrence matrix and Tamura coarseness are used to transfer images to multiscale texture feature vectors. Then, an unsupervised dynamic learning mechanism is used to classify these vectors. In the simulation experiments with a resolution of 40 pixels, the accuracy, precision, F1-score and AUC of the proposed method reach 91.500%, 92.780%, 91.370%, and 91.500%, respectively. The experimental results show that the proposed method can provide an effective reference for breast cancer diagnosis.
更多查看译文
关键词
Breast cancer image classification,Multi-scale texture analysis,Dynamic learning strategy
AI 理解论文
溯源树
样例
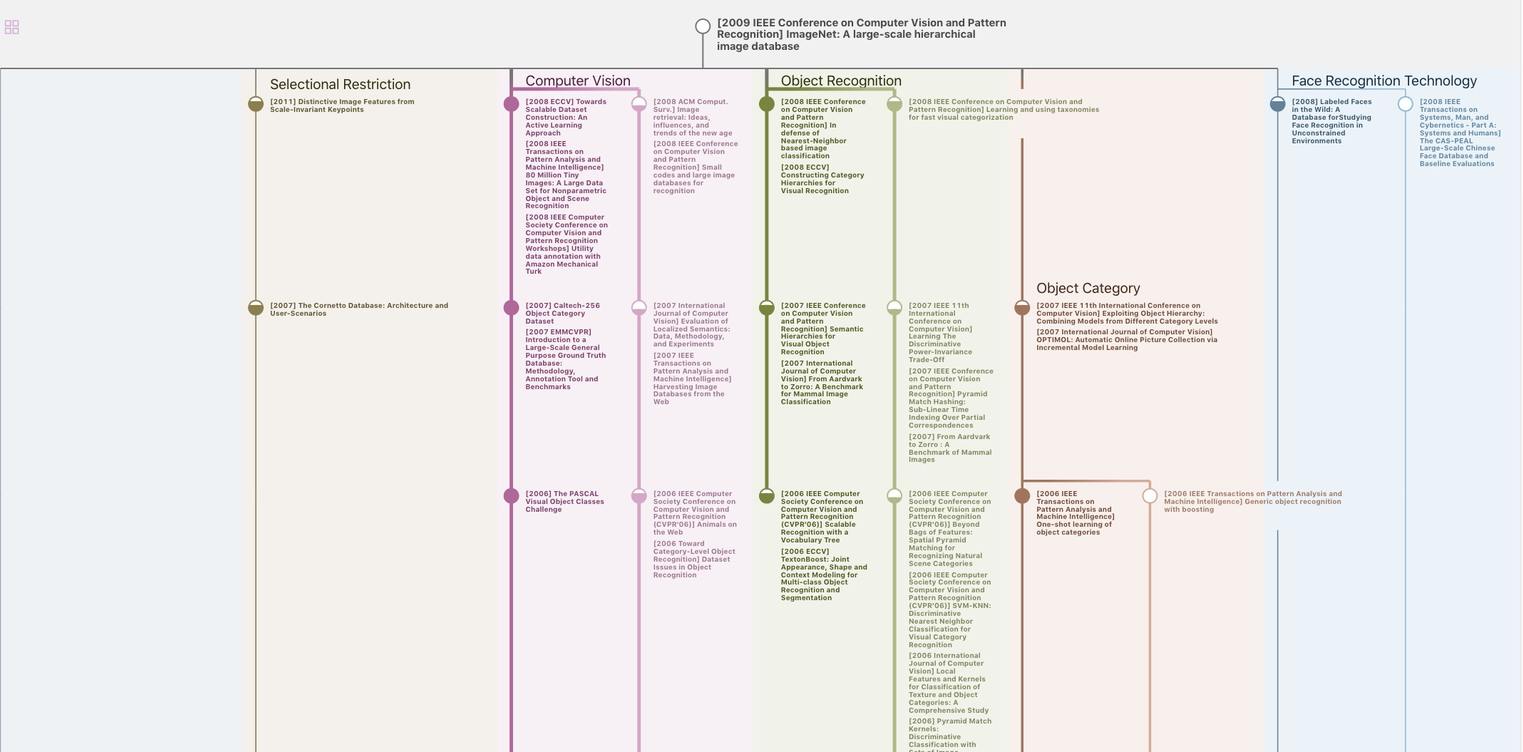
生成溯源树,研究论文发展脉络
Chat Paper
正在生成论文摘要