Lightweight Privacy Protection via Adversarial Sample
Electronics(2024)
摘要
Adversarial sample-based privacy protection has its own advantages compared to traditional privacy protections. Previous adversarial sample privacy protections have mostly been centralized or have not considered the issue of hardware device limitations when conducting privacy protection, especially on the user’s local device. This work attempts to reduce the requirements of adversarial sample privacy protections on devices, making the privacy protection more locally friendly. Adversarial sample-based privacy protections rely on deep learning models, which generally have a large number of parameters, posing challenges for deployment. Fortunately, the model structural pruning technique has been proposed, which can be employed to reduce the parameter count of deep learning models. Based on the model pruning technique Depgraph and existing adversarial sample privacy protections AttriGuard and MemGuard, we design two structural pruning-based adversarial sample privacy protections, in which the user obtains the perturbed data through the pruned deep learning model. Extensive experiments are conducted on four datasets, and the results demonstrate the effectiveness of our adversarial sample privacy protection based on structural pruning.
更多查看译文
关键词
structural pruning,privacy protection,adversarial sample
AI 理解论文
溯源树
样例
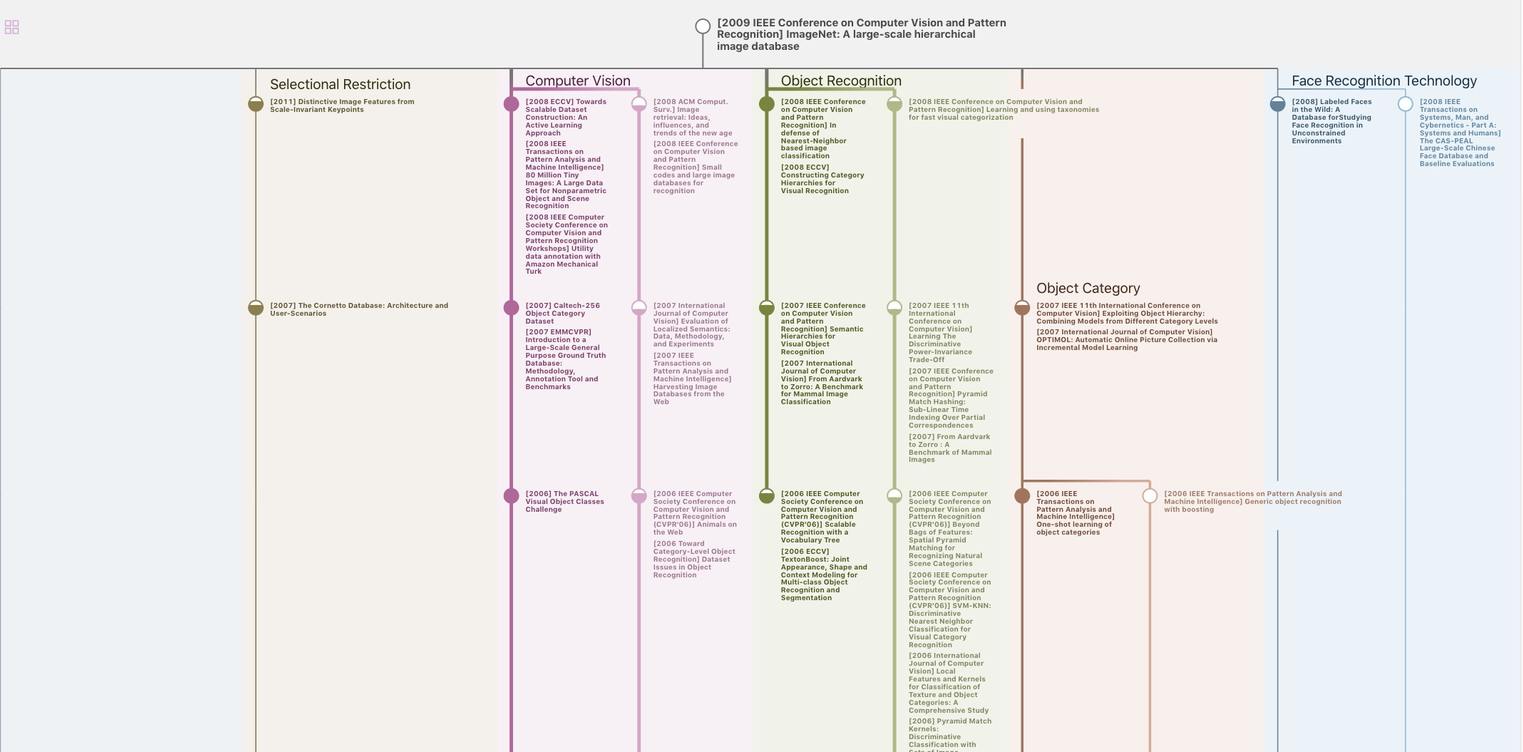
生成溯源树,研究论文发展脉络
Chat Paper
正在生成论文摘要