Binary Malware Detection via Heterogeneous Information Deep Ensemble Learning.
International Conference on Parallel and Distributed Systems(2023)
摘要
Dynamic malware detection refers to detecting mal-ware by inferring the run-time trace of malware, i.e., a sequence of API calls. In this paper, we proposed HeteroNet, a novel dynamic malware detection model. The main idea of HeteroNet is that it integrates multiple deep learning models which use heterogeneous dynamic features of malware samples.Specifically, we implement three heterogeneous deep learning based models to learn various features from three representations, namely API name sequence, API resource graph and API call graph, respectively, each of the representation is built from the run-time trace of malware. Meanwhile, several methods such as attention mechanism and graph neural networks are applied in base models, according to the characteristics of API calls. Finally, an ensemble algorithm is used to integrate the outputs of three base models. We trained and evaluated HeteroNet on a dataset of 28,770 samples. The precision of the model on the testing set reached 98.40%, which is 1.20% higher than the best result of baselines. Moreover, HeteroNet is more robust against concept drift than other baselines.
更多查看译文
AI 理解论文
溯源树
样例
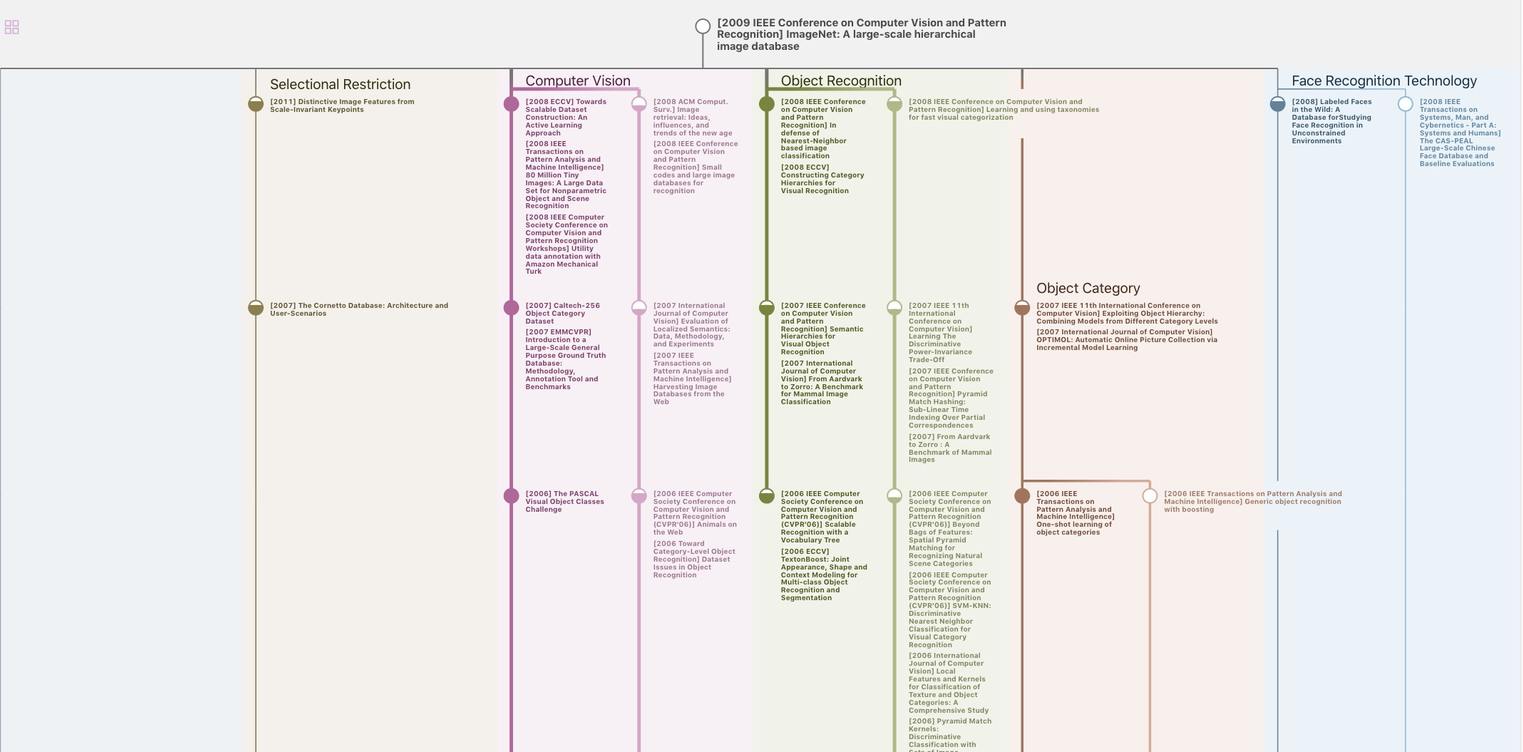
生成溯源树,研究论文发展脉络
Chat Paper
正在生成论文摘要