Addressing Client Heterogeneity in Synchronous Federated Learning: The CHAFL Approach.
International Conference on Parallel and Distributed Systems(2023)
摘要
Federated learning (FL) is proposed to address the security vulnerabilities of conventional distributed deep learning. Since the capabilities of participating FL clients are highly variable in terms of both statistical and system aspects, FL training will face diminished convergence accuracy and speed. Hence, we propose CHAFL (client heterogeneity aware federated learning) to address client heterogeneity (i.e., statistical and system heterogeneity) in synchronous FL. CHAFL selects clients based on global loss with contribution, defined as local loss, enabling higher round-to-accuracy for the global model than previous studies. Additionally, to handle system heterogeneity, it proposes a lightweight algorithm that eliminates the profiling process previously employed to calculate adaptive local epochs in existing studies, thereby improving time-to-accuracy. In order to verify the effectiveness of CHAFL, we exploit three benchmark datasets on non-IID and system heterogeneous setting for empirical evaluation. Compared to the baseline, CHAFL achieves an accuracy improvement of 3.1 to 5.7%, along with 1.04 to 1.73x higher round-to-accuracy and 1.03 to 1.98x higher time-to-accuracy.
更多查看译文
AI 理解论文
溯源树
样例
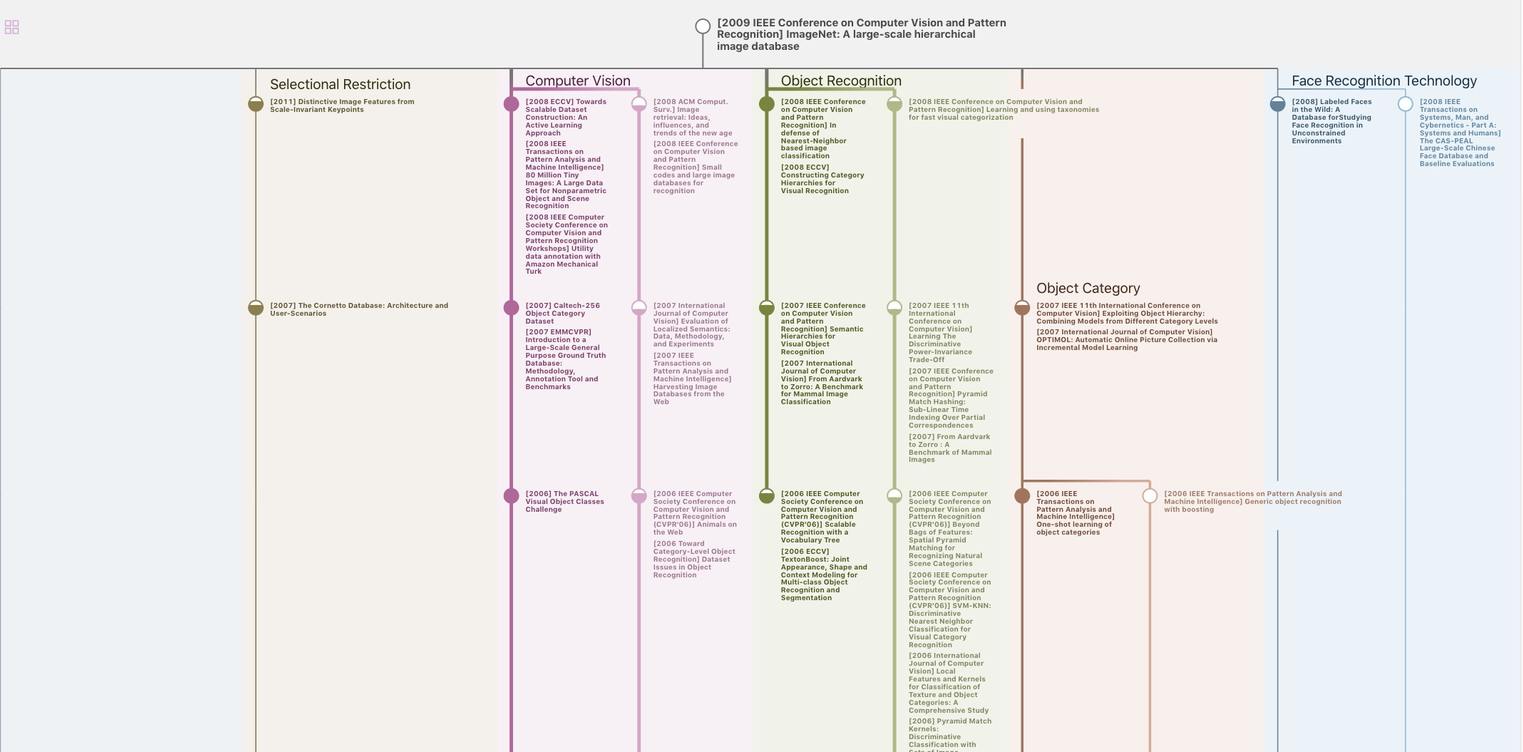
生成溯源树,研究论文发展脉络
Chat Paper
正在生成论文摘要