STGAT: Spatial-Temporal Graph Attention Networks for Traffic Flow Prediction.
International Conference on Parallel and Distributed Systems(2023)
摘要
Accurate traffic flow prediction is of great importance in Intelligent Transportation System (ITS) for improving traffic efficiency, reducing congestion and so on. However, due to the complex spatial and temporal dependencies, achieving the accurate prediction is challenging. Traditional attention-based networks for traffic flow prediction typically use sine and cosine functions to do position encoding, which fail to capture the spatial and temporal dependencies and do not contain the graph structure information. In this paper, we propose a novel model named Spatial-Temporal Graph ATtention networks (STGAT), which leverages structure-aware self-attention mechanism to predict future traffic flow. The model introduces temporal multi-head self-attention modules, and designs spatial multi-head graph self-attention modules with structure-aware graph filters to extract more temporal and spatial information. Besides, our model adopts temporal and spatial position embedding layers to capture the spatial-temporal dependencies in traffic flow data. Experimental results show that STGAT shows better prediction performance than the state-of-art models on three real-world datasets PEMS04, PEMS07 and PEMS08. For example, we observe up to 10.38% improvement in terms of Mean Absolute Percentage Error (MAPE) compared with the well-known model ASTGNN.
更多查看译文
关键词
Traffic flow prediction,self-attention,spatial-temporal,graph attention network
AI 理解论文
溯源树
样例
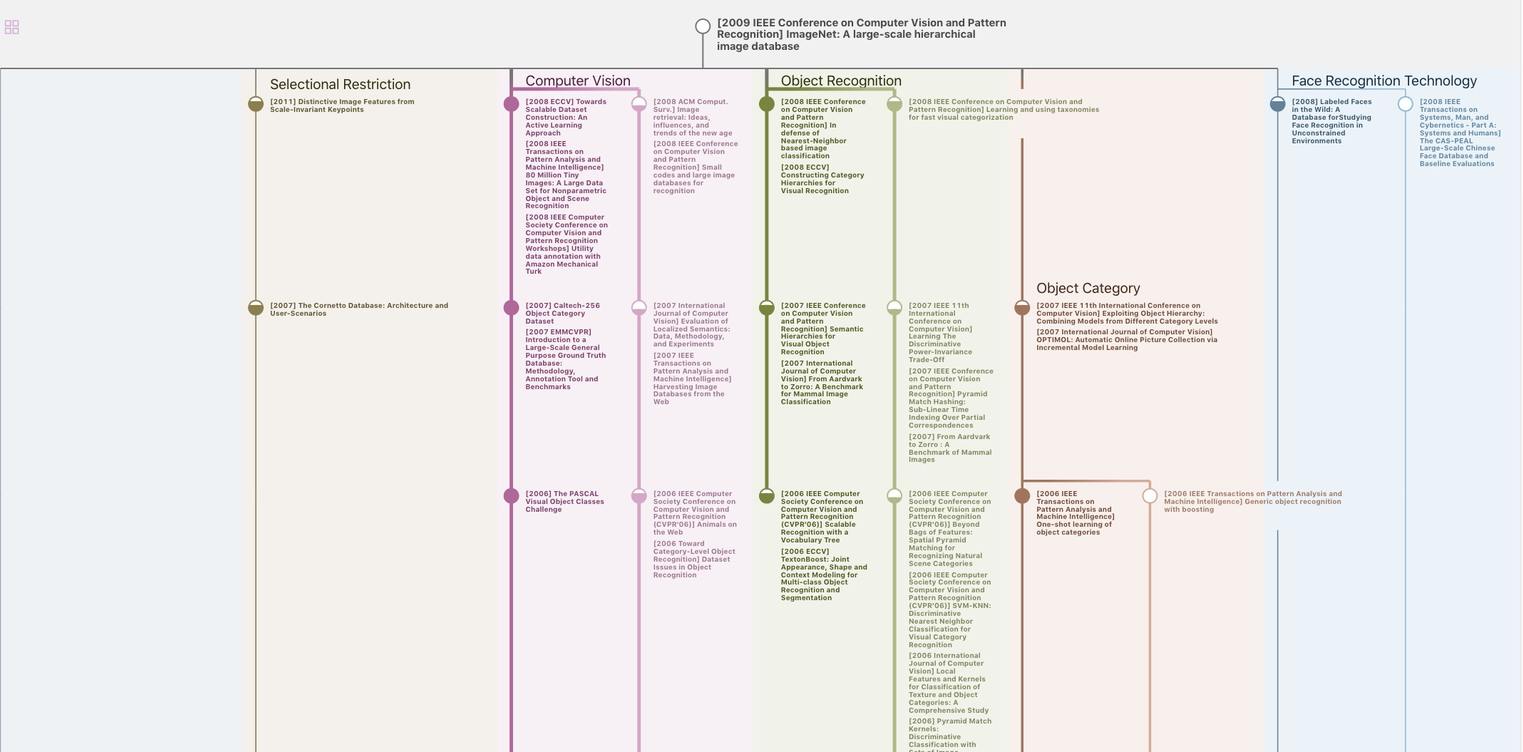
生成溯源树,研究论文发展脉络
Chat Paper
正在生成论文摘要