When CPN Meets AI: Resource Provisioning for Inference Query upon Computing Power Network.
International Conference on Parallel and Distributed Systems(2023)
摘要
Performing machine learning inference at the network edge, named Edge Inference, showing benefits like low latency, reduced data traffic, and improved user privacy, has attracted massive attention. Computing Power Network (CPN) creates opportunities for edge inference, but also poses multiple challenges for service providers, including computing power selections from different enterprises, the time-coupled decision for resource provisioning and the unpredictable CPN network status. To overcome these challenges, this study formulates a time-varying integer program problem whose goal is to minimize long-term costs, involving switching costs, operational costs, communication costs, and queuing costs. Then we design a group of polynomial-time online algorithms to make online decisions, by taking into account stochastic inputs. Our algorithms adaptively make control decisions by solving delicately constructed subproblems based on the inputs predicted via online learning. Specifically, we first obtain fractional solutions which are transformed into integers for deployment with expectations preserving. Furthermore, we conduct a rigorous proof and establish the competitive ratio which highlights the difference between the performance of our proposed algorithms and the offline optimum. Our comprehensive evaluations, using datasets from real systems, demonstrate that our algorithms outperform multiple alternatives, up to an average of 35% cost reduction, confirming the effectiveness of our algorithms.
更多查看译文
关键词
Resource Provisioning,Computing Power Network
AI 理解论文
溯源树
样例
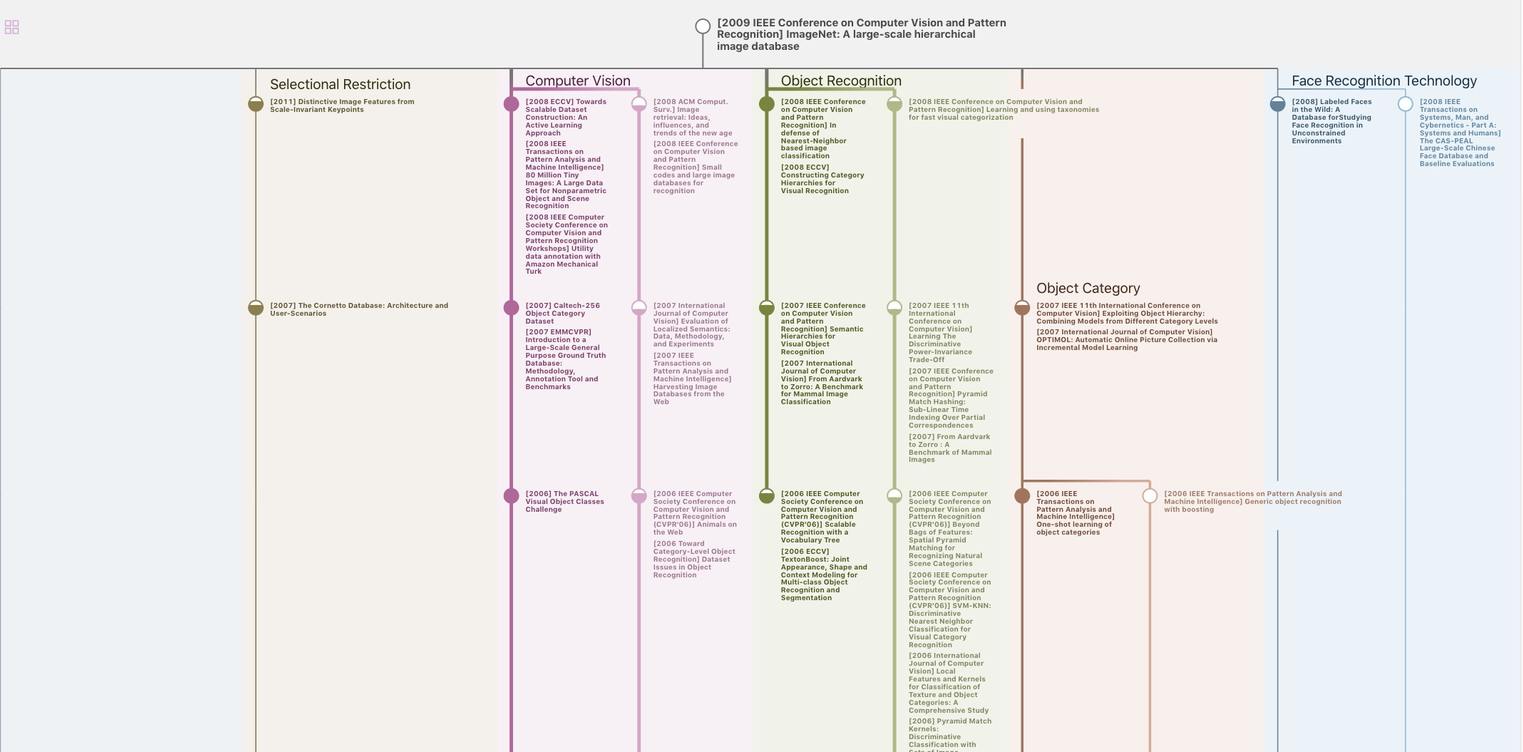
生成溯源树,研究论文发展脉络
Chat Paper
正在生成论文摘要