Feature Engineering for Highly Irregular Network Traffic Prediction.
VCC(2023)
摘要
Predicting network traffic with reasonable accuracy in short-term horizons is often a challenging task due to high randomness. Network traffic data can be decomposed into a number of features creating a highly complex multivariate time series. Usually, this randomness is not equally distributed across all related time series, which decreases the predictability of the whole multivariate time series. This paper presents a novel feature engineering solution that can potentially increase prediction performance for models where the prediction horizon becomes especially short (e.g., in 5 seconds range). Specifically, we propose two feature extraction techniques based on a) IP changes and b) flow direction flips. We compare the predictability performance of our proposed approaches with the baseline scheme (i.e., no feature extraction). The comparison was performed on a network traffic dataset collected from commercial live networks. Although the proposed approaches capture the specifics of the provided dataset, the outcomes of our research can be generalized to any encrypted network traffic. Our results show improvements in MAPE metrics and up to a 93% reduction in computation time with our proposed approaches.
更多查看译文
关键词
Feature Engineering,Flow Characterization,Network Traffic,Machine Learning
AI 理解论文
溯源树
样例
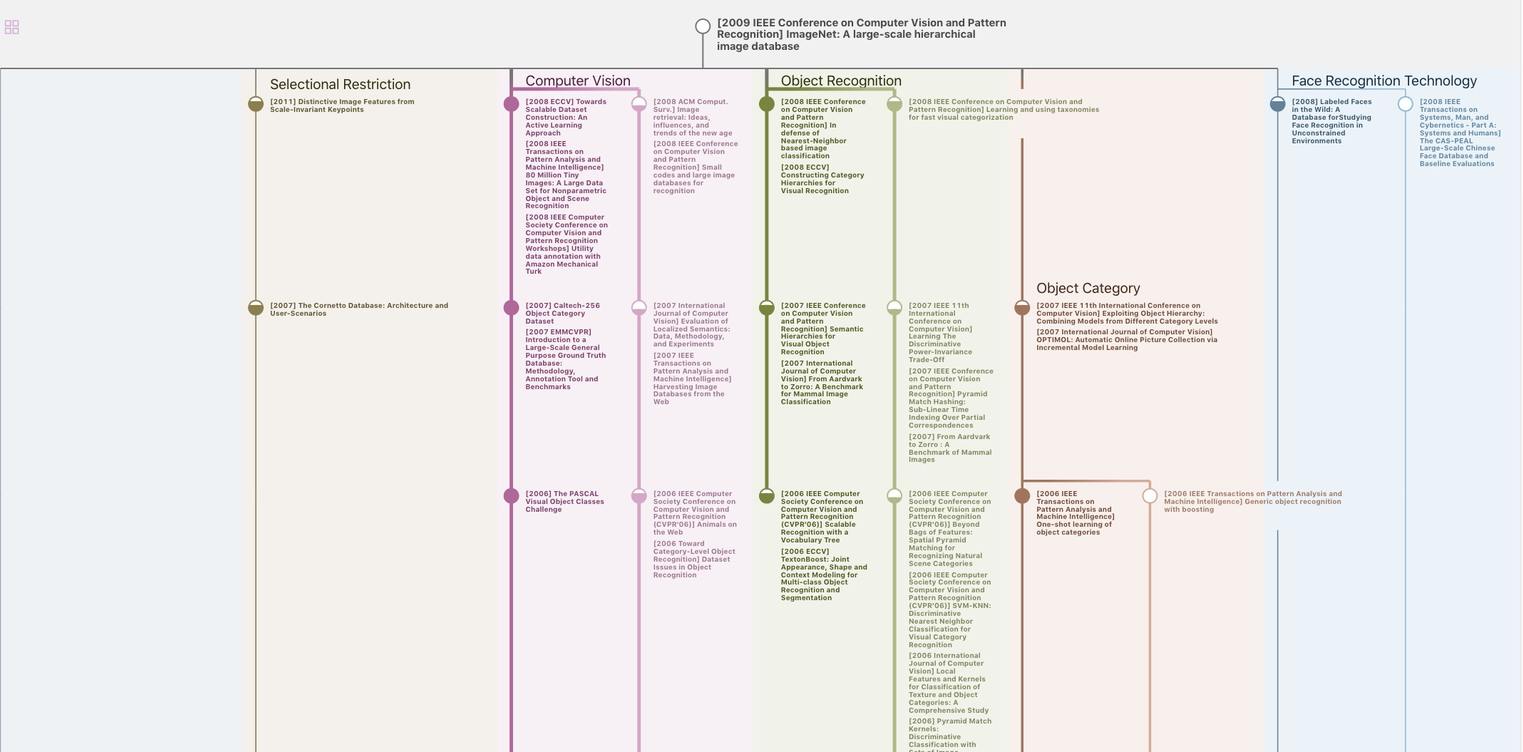
生成溯源树,研究论文发展脉络
Chat Paper
正在生成论文摘要