Advancing Sustainable IoT Appliance Load Monitoring Through Edge-Enabled Federated Transfer Learning
2024 International Conference on Green Energy, Computing and Sustainable Technology (GECOST)(2024)
摘要
Non-intrusive Appliance Load Monitoring (NALM) is essential for efficient electricity consumption tracking in households, promoting eco-friendly practices, and cost reduction. Privacy concerns in real-world NALM implementations can be addressed using federated learning. However, challenges persist, such as limited training data, edge model customization, and resource constraints. We introduce a practical federated learning framework for NALM, leveraging cloud-based model compression, collaborative data collection, and personalized edge and multi-task learning models through unsupervised transfer learning. Our advanced load monitoring model offers precise energy disaggregation through real-world experimentation, making it a leading solution for non-intrusive appliance load monitoring on edge devices. It maintains excellent learning performance and user privacy. Future research should aim to enhance federated learning efficiency and tackle remaining challenges in practical NALM implementations
更多查看译文
关键词
Edge AI,federated learning,non-intrusive load monitoring
AI 理解论文
溯源树
样例
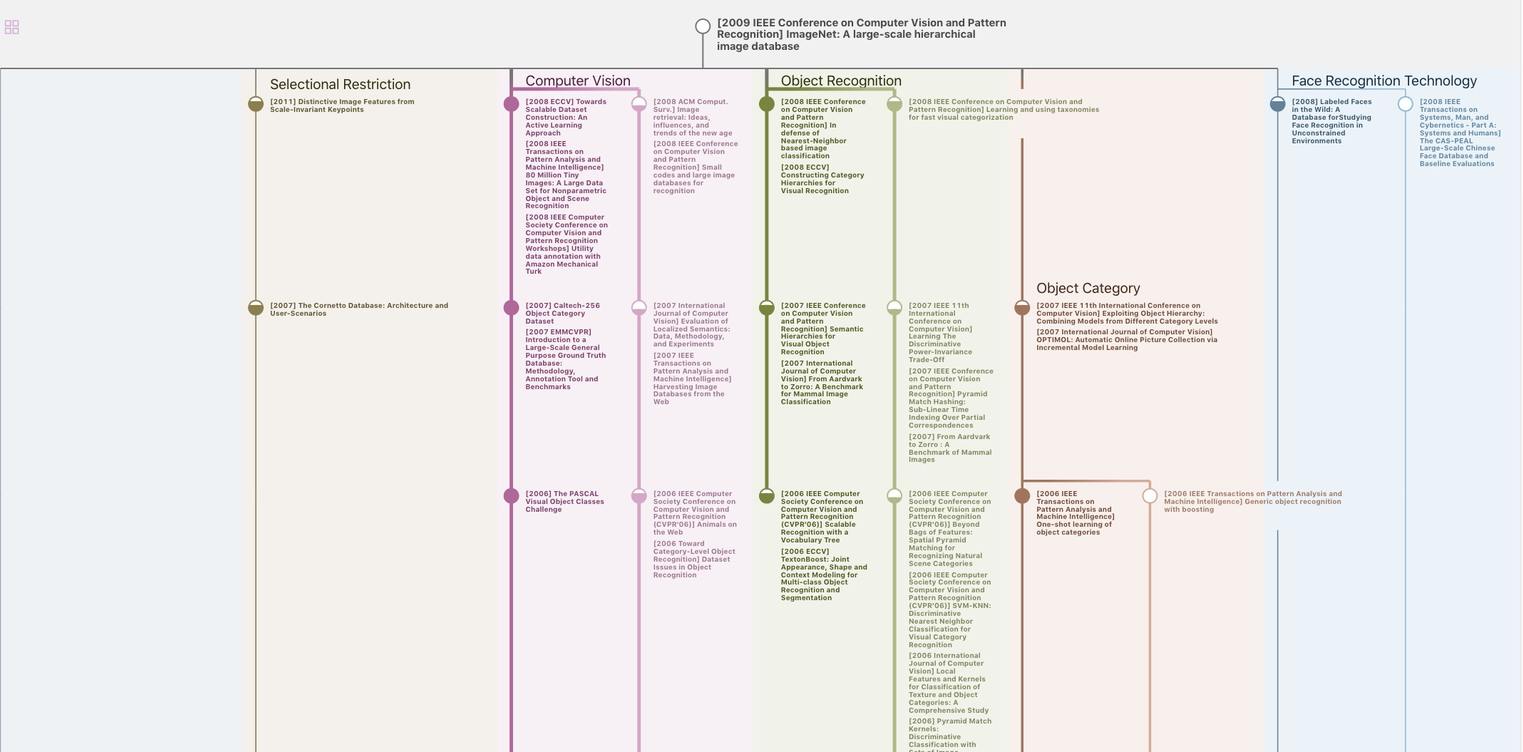
生成溯源树,研究论文发展脉络
Chat Paper
正在生成论文摘要