pFedTD: Personalized federated learning using global and local knowledge distillation.
International Conference on Parallel and Distributed Systems(2023)
摘要
Federated learning (FL), as a machine learning method for multi-client model aggregation, faces performance problems caused by data heterogeneity. At the same time, global model aggregation will also cause customers to forget their own personalized knowledge, resulting in poor local training results. To this end, we propose a double distillation personalized federated learning framework (pFedTD) that combines local self-knowledge distillation and global non-ground truth class knowledge distillation. This method effectively balances personalization and global performance by extracting the personalized and global historical knowledge of each client and using a parameter adaptation method to weigh the intensity of self-distillation and global distillation. pFedTD can also perform stably on large-scale heterogeneous data and can alleviate local and global forgetting problems. Our experiments demonstrate that our method outperforms other baselines even on non-IID data.
更多查看译文
关键词
Federated learning,personalization,knowledge distillation
AI 理解论文
溯源树
样例
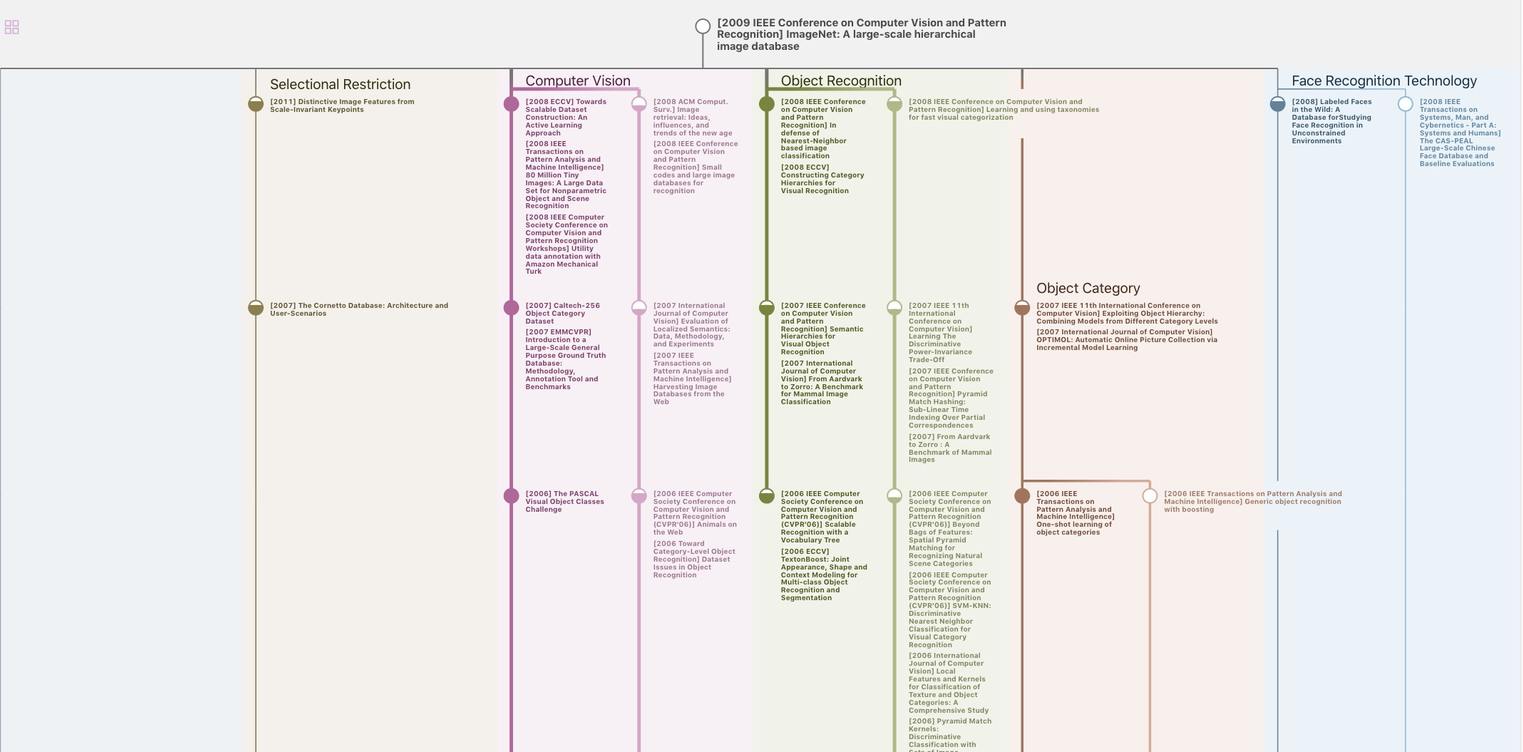
生成溯源树,研究论文发展脉络
Chat Paper
正在生成论文摘要