SRENet: Structure recovery ensemble network for single image deraining
Applied Intelligence(2024)
摘要
Single-image deraining remains a formidable challenge. Its objective is not only to eliminate rain streaks from the target image but also to restore its spatial details and high-level contextual structure. Recently, numerous CNN-based methods have been introduced for this purpose. While these methods can adeptly remove rain streaks, they often face difficulties in restoring high-quality, rain-free images while preserving clear and precise structures—especially when those structures resemble rain streaks. To extract a diverse array of feature information for image structure restoration, we introduce a network called the Structure Recovery Ensemble Network (SRENet). This network comprises three learners, each designed with three parallel subnetworks. This arrangement allows for the acquisition of a broader range of structural features by transitioning from deep vertical networks to horizontal parallelization across multiple subnetworks. To extract structural features without interference from rain streak information, we employ an independent loss strategy in which each learner is trained to address different specific challenges in the image deraining process, such as rain removal and structure restoration. Additionally, we design a guided fusion module to seamlessly integrate features from the various learners and subnetworks. Comprehensive experiments on benchmark datasets confirm that our proposed method sets new state-of-the-art standards. On the synthetic datasets, SRENet achieved average improvements of 0.85
更多查看译文
关键词
Single image deraining,Structure recovery,Ensemble network,Guided fusion
AI 理解论文
溯源树
样例
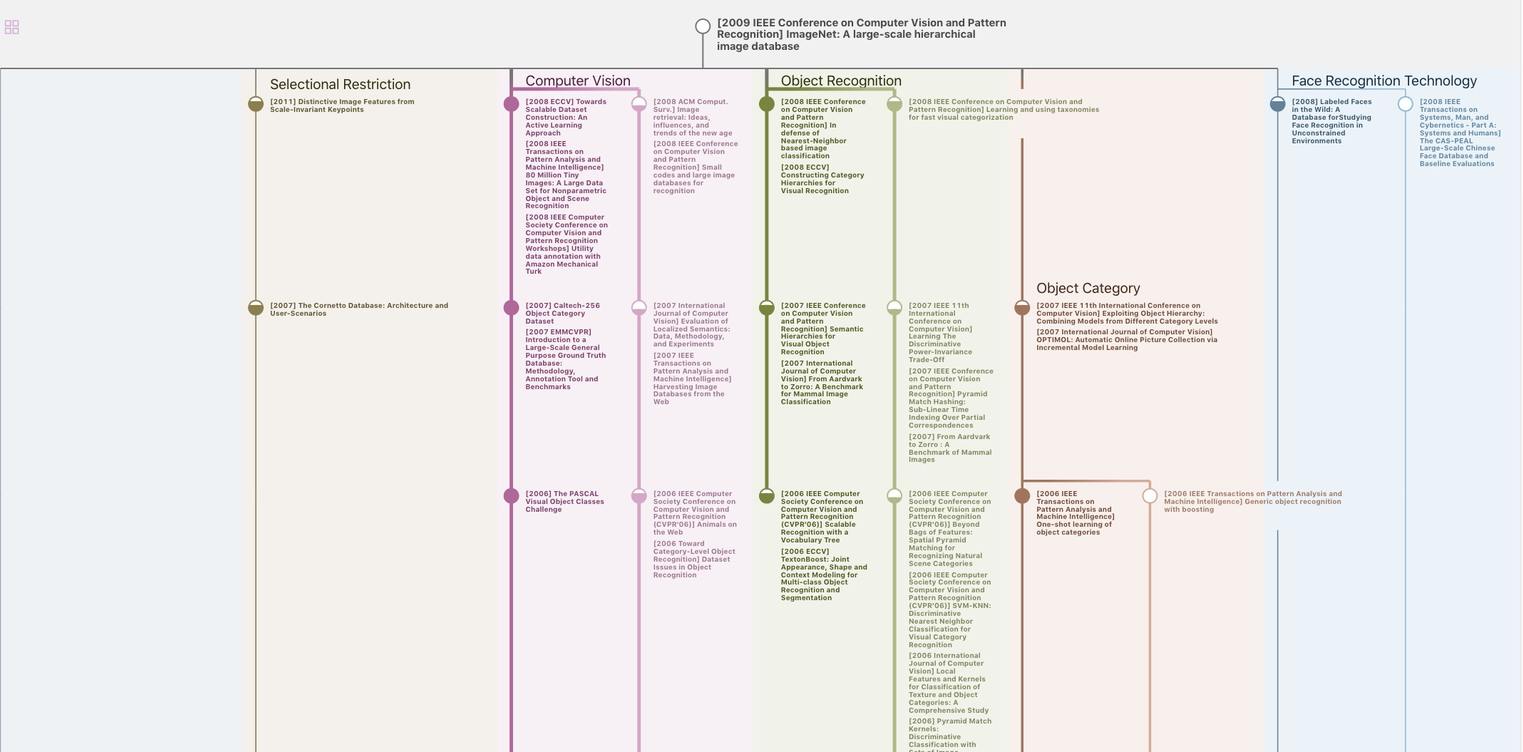
生成溯源树,研究论文发展脉络
Chat Paper
正在生成论文摘要