SDAN-YOLO:Self-attention domain adaptive network for YOLOv7.
International Conference on Parallel and Distributed Systems(2023)
摘要
Most of the existing unsupervised domain adaptive object detection methods are based on twostage object detectors such as Faster-RCNN because the region proposal network(RPN) module, which onestage detectors don’t have, can help the model achieve instance-level local domain adaption. Although this type of methods have good detection accuracy, the real-time performance cannot meet the requirements of scenarios such as autonomous driving. To this end, we propose a self-attention domain adaptive network suitable for the one-stage detector YOLOv7, which performs the self-attention to extract the position information related to the foreground target as RPN to do instance-level local domain adaptation. At the same time, we also propose a local self-attention augmentation method to suppress the attention noise so that the model can pay attention to the foreground target accurately. Considering the fact that the detection ability of model is from weak to robust during training, we apply coarse-to-fine alignment that global domain adaptation first aligns the the global feature coarsely and transferring to local domain adaptation gradually to finely align the local feature of the foreground target. Our proposed method is validated on Cityspaces, Foggy Cityspaces, KITTI and Sim10K datasets, outperforming existing domain adaptive object detection methods.
更多查看译文
关键词
Domain adaptation,Object detection,Self-attention,YOLOv7,Coarse-to-fine alignment
AI 理解论文
溯源树
样例
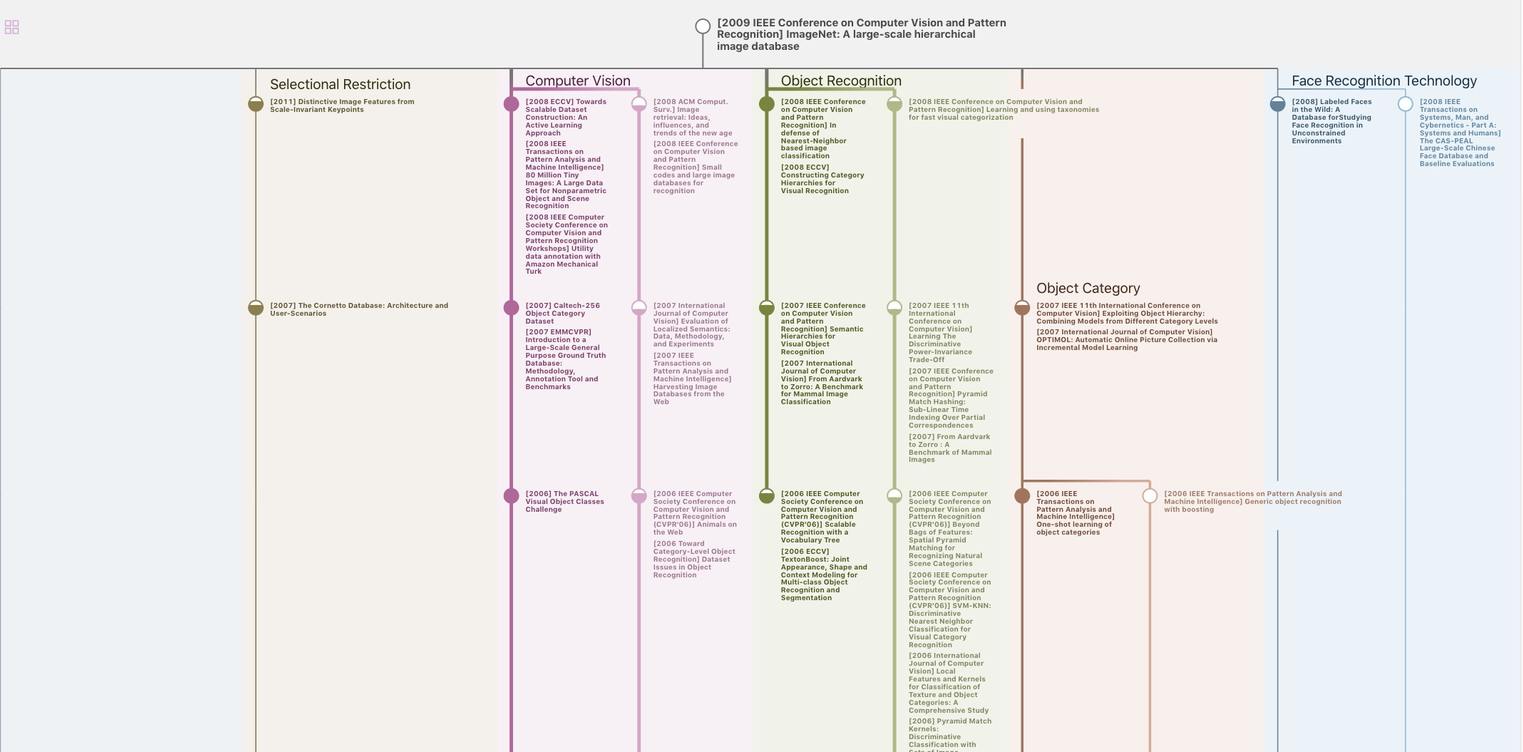
生成溯源树,研究论文发展脉络
Chat Paper
正在生成论文摘要