Incorporating ESO into Deep Koopman Operator Modeling for Control of Autonomous Vehicles
IEEE TRANSACTIONS ON CONTROL SYSTEMS TECHNOLOGY(2024)
摘要
Koopman operator theory is a kind of data-driven modeling approach that accurately captures the nonlinearities of mechatronic systems such as vehicles against physics-based methods. However, the infinite-dimensional Koopman operator is impossible to implement in real-world applications. To approximate the infinite-dimensional Koopman operator through collection dataset rather than manual trial and error, we adopt deep neural networks (DNNs) to extract basis functions by offline training and map the nonlinearities of vehicle planar dynamics into a linear form in the lifted space. Besides, the effects of the dimensions of basis functions on the model accuracy are explored. Furthermore, the extended state observer (ESO) is introduced to online estimate the total disturbance in the lifted space and compensate for the modeling errors and residuals of the learned deep Koopman (DK) operator while also improving its generalization. Then, the proposed model is applied to predict vehicle states within prediction horizons and later formulates the constrained finite-time optimization problem of model predictive control (MPC), i.e., ESO-DKMPC. In terms of the trajectory tracking of autonomous vehicles, the ESO-DKMPC generates the wheel steering angle to govern lateral motions based on the decoupling control structure. The various conditions under the double-lane change scenarios are built on the CarSim/Simulink co-simulation platform, and extensive comparisons are conducted with the linear MPC (LMPC) and nonlinear MPC (NMPC) informed by the physics-based model. The results indicate that the proposed ESO-DKMPC has better tracking performance and moderate efficacy both within linear and nonlinear regions.
更多查看译文
关键词
Aerospace electronics,Vehicle dynamics,Observers,Artificial neural networks,Training,Predictive models,Computational modeling,Autonomous vehicles,deep neural network (DNN),extended state observer (ESO),Koopman operator modeling,model predictive control (MPC),trajectory tracking control
AI 理解论文
溯源树
样例
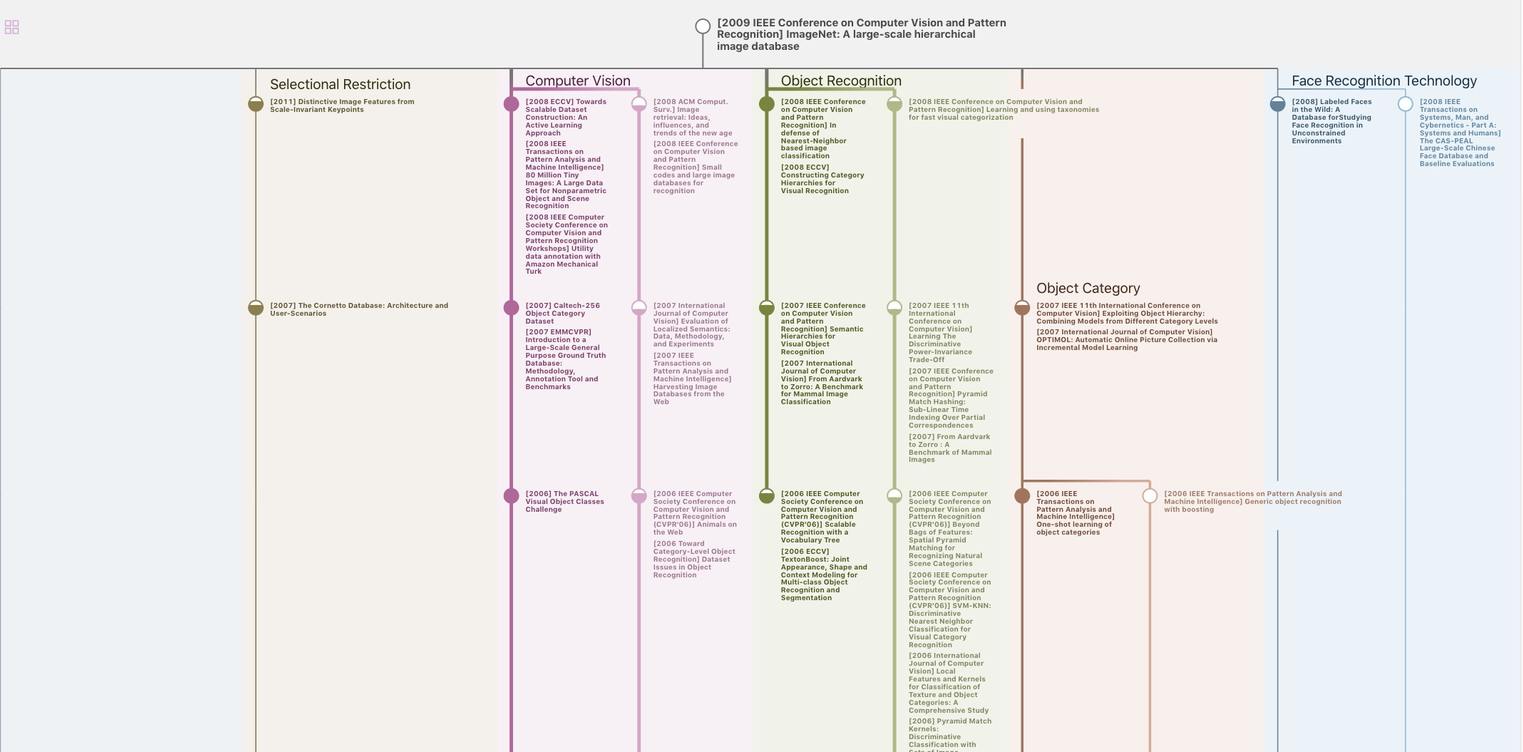
生成溯源树,研究论文发展脉络
Chat Paper
正在生成论文摘要