Enhancing Path Information with Reinforcement Learning for Few-shot Knowledge Graph Completion.
International Conference on Parallel and Distributed Systems(2023)
摘要
The emergence of big data has made knowledge graphs (KGs) an effective means of representing structured knowledge, and few-shot knowledge graph completion (FKGC) has recently received increasing attention, which attempts to forecast missing information for relations with few-shot related facts. In this regard, several deep learning-based and embedding-based methods have been proposed for FKGC. However, most existing methods overlook multi-hop path information and only utilize the immediate neighbors of relevant entities when encoding and matching entity pairs, potentially limiting their performance. In this paper, we propose an Enhancing Path Information with Reinforcement Learning (EPIRL) approach for FKGC. Specifically, we introduce a reinforcement learning framework to construct a reasoning subgraph, aiming to thoroughly uncover the inferential path rules between support and query triples. Then, we utilize an interaction focused matching model to capture the inherent connections among these reasoning paths. To further improve performance, we incorporate a relational attention mechanism aimed at emphasizing the influence of pivotal paths. Extensive experiments demonstrate that our model outperforms several state-of-the-art methods on the frequently-used benchmark datasets FB15k237-One and NELL-One.
更多查看译文
关键词
Knowledge graph completion,Few-shot learning,Reinforcement learning
AI 理解论文
溯源树
样例
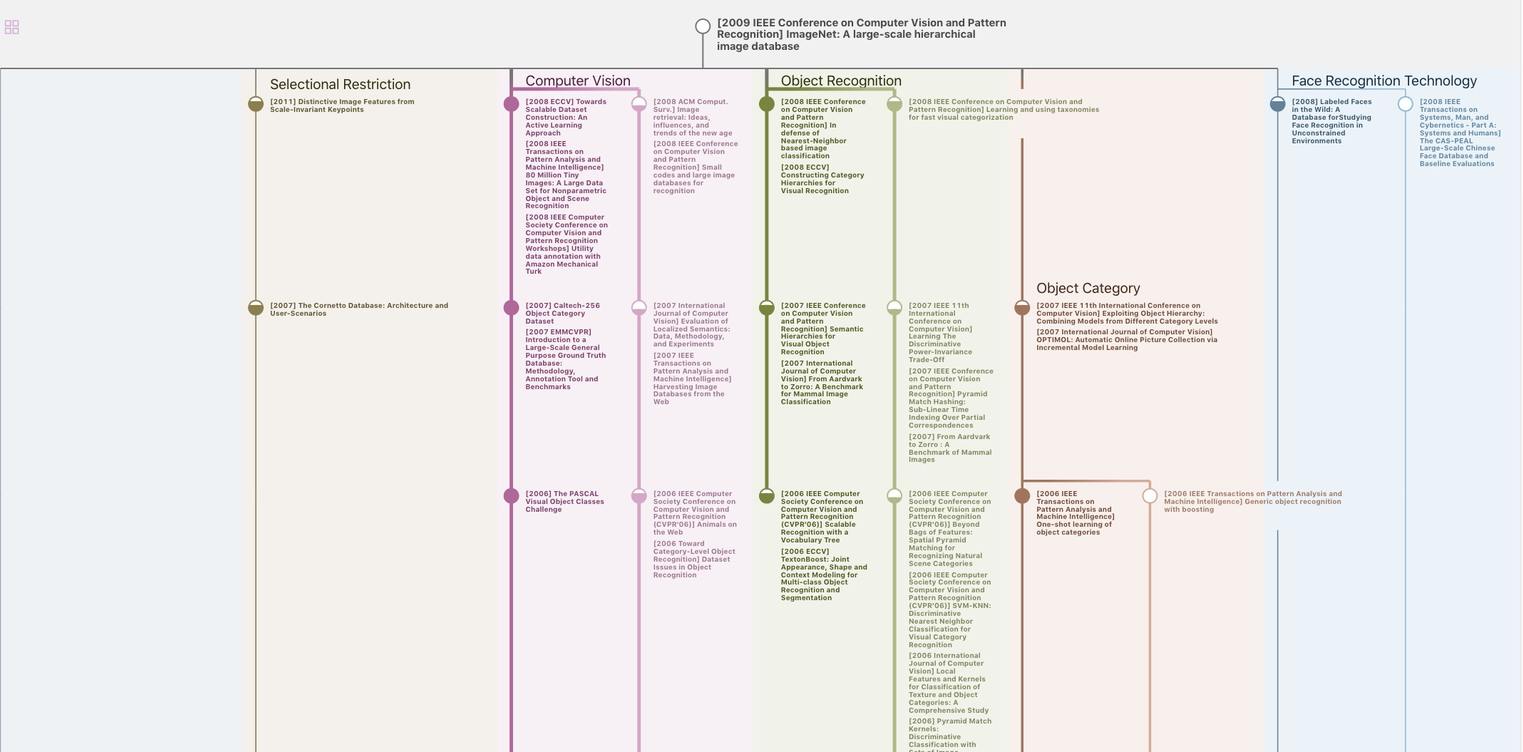
生成溯源树,研究论文发展脉络
Chat Paper
正在生成论文摘要