CL&CD: Contrastive Learning and Cluster Description for Zero-Shot Relation Extraction
Knowledge-Based Systems(2024)
摘要
Zero-shot Relation Extraction (ZRE) is designed to identify new relations when the model is adapted to a new environment in a new domain. The majority of existing ZRE methods employ distant supervision for data labelling, which inevitably leads to incomplete annotations and noise. To handle this problem, we propose a ZRE framework based on Contrastive Learning and Cluster Description (CL&CD), a two-stage contrastive learning method is used to train labelled data which consists pseudo-labelled data and labelled data. The framework can effectively promote the model mapping the instances of the same relations to the adjacent vector space better. The module of clustering description reasonably optimizes the time of manual intervention and enormously reduces the consumption of human resources. Experimental results on Wiki-ZSL and FewRel show the superior performance of the CL&CD, which outperforms all baseline models including the state-of-the-art RCL. Furthermore, CL&CD improves F1-Score by up to 8% in both Wiki-ZSL and FewRel with 15 unseen relations.
更多查看译文
关键词
Relation extraction,Clustering description,Contrastive learning,Zero-shot
AI 理解论文
溯源树
样例
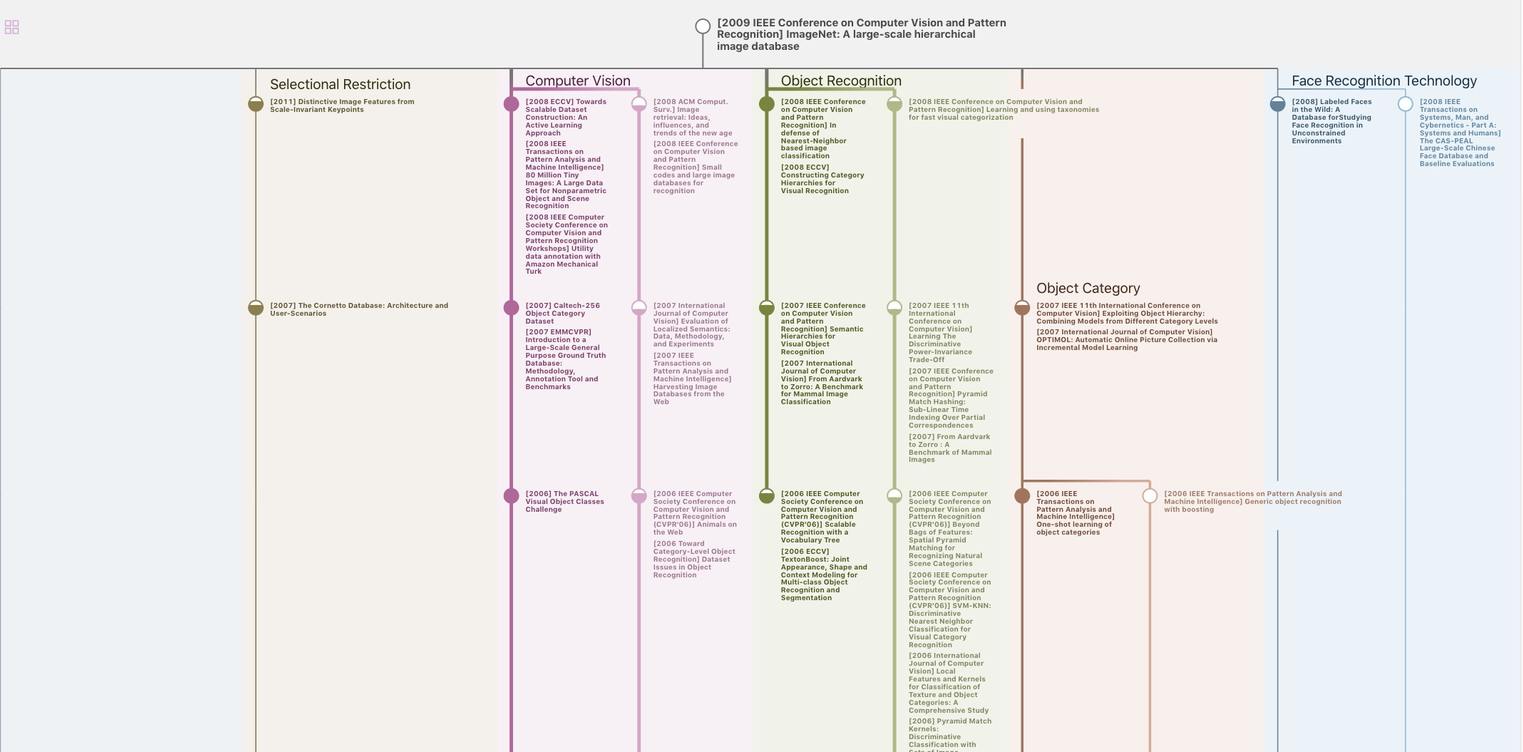
生成溯源树,研究论文发展脉络
Chat Paper
正在生成论文摘要