On the Impact of Black-box Deployment Strategies for Edge AI on Latency and Model Performance
arxiv(2024)
摘要
Deciding what combination of operators to use across the Edge AI tiers to
achieve specific latency and model performance requirements is an open question
for MLOps engineers. This study aims to empirically assess the accuracy vs
inference time trade-off of different black-box Edge AI deployment strategies,
i.e., combinations of deployment operators and deployment tiers. In this paper,
we conduct inference experiments involving 3 deployment operators (i.e.,
Partitioning, Quantization, Early Exit), 3 deployment tiers (i.e., Mobile,
Edge, Cloud) and their combinations on four widely used Computer-Vision models
to investigate the optimal strategies from the point of view of MLOps
developers. Our findings suggest that Edge deployment using the hybrid
Quantization + Early Exit operator could be preferred over non-hybrid operators
(Quantization/Early Exit on Edge, Partition on Mobile-Edge) when faster latency
is a concern at medium accuracy loss. However, when minimizing accuracy loss is
a concern, MLOps engineers should prefer using only a Quantization operator on
edge at a latency reduction or increase, respectively over the Early
Exit/Partition (on edge/mobile-edge) and Quantized Early Exit (on edge)
operators. In scenarios constrained by Mobile CPU/RAM resources, a preference
for Partitioning across mobile and edge tiers is observed over mobile
deployment. For models with smaller input data samples (such as FCN), a
network-constrained cloud deployment can also be a better alternative than
Mobile/Edge deployment and Partitioning strategies. For models with large input
data samples (ResNet, ResNext, DUC), an edge tier having higher
network/computational capabilities than Cloud/Mobile can be a more viable
option than Partitioning and Mobile/Cloud deployment strategies.
更多查看译文
AI 理解论文
溯源树
样例
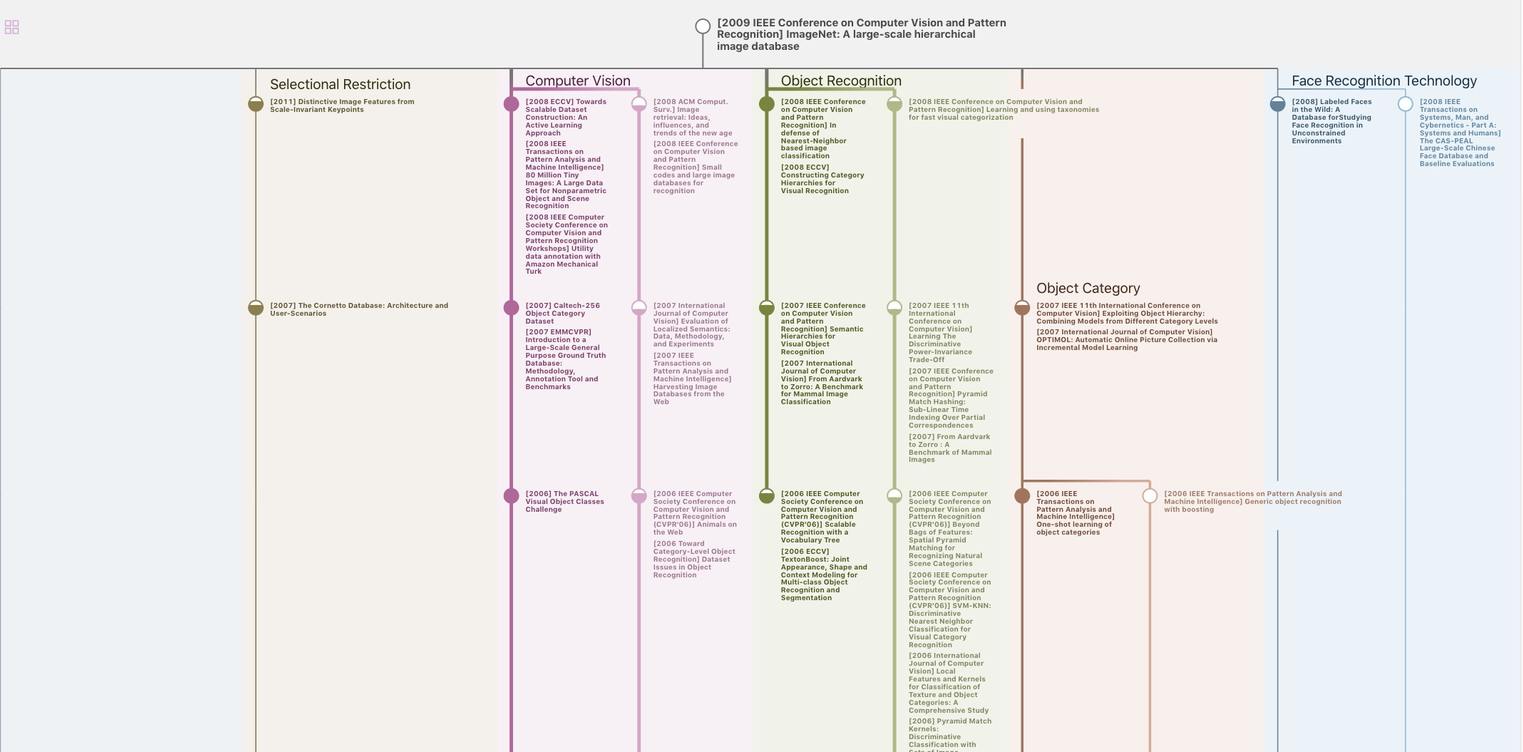
生成溯源树,研究论文发展脉络
Chat Paper
正在生成论文摘要