Practical Acceleration of the Condat-Vũ Algorithm
arxiv(2024)
摘要
The Condat-Vũ algorithm is a widely used primal-dual method for optimizing
composite objectives of three functions. Several algorithms for optimizing
composite objectives of two functions are special cases of Condat-Vũ,
including proximal gradient descent (PGD). It is well-known that PGD exhibits
suboptimal performance, and a simple adjustment to PGD can accelerate its
convergence rate from 𝒪(1/T) to 𝒪(1/T^2) on convex
objectives, and this accelerated rate is optimal. In this work, we show that a
simple adjustment to the Condat-Vũ algorithm allows it to recover accelerated
PGD (APGD) as a special case, instead of PGD. We prove that this accelerated
Condat–Vũ algorithm achieves optimal convergence rates and significantly
outperforms the traditional Condat-Vũ algorithm in regimes where the
Condat–Vũ algorithm approximates the dynamics of PGD. We demonstrate the
effectiveness of our approach in various applications in machine learning and
computational imaging.
更多查看译文
AI 理解论文
溯源树
样例
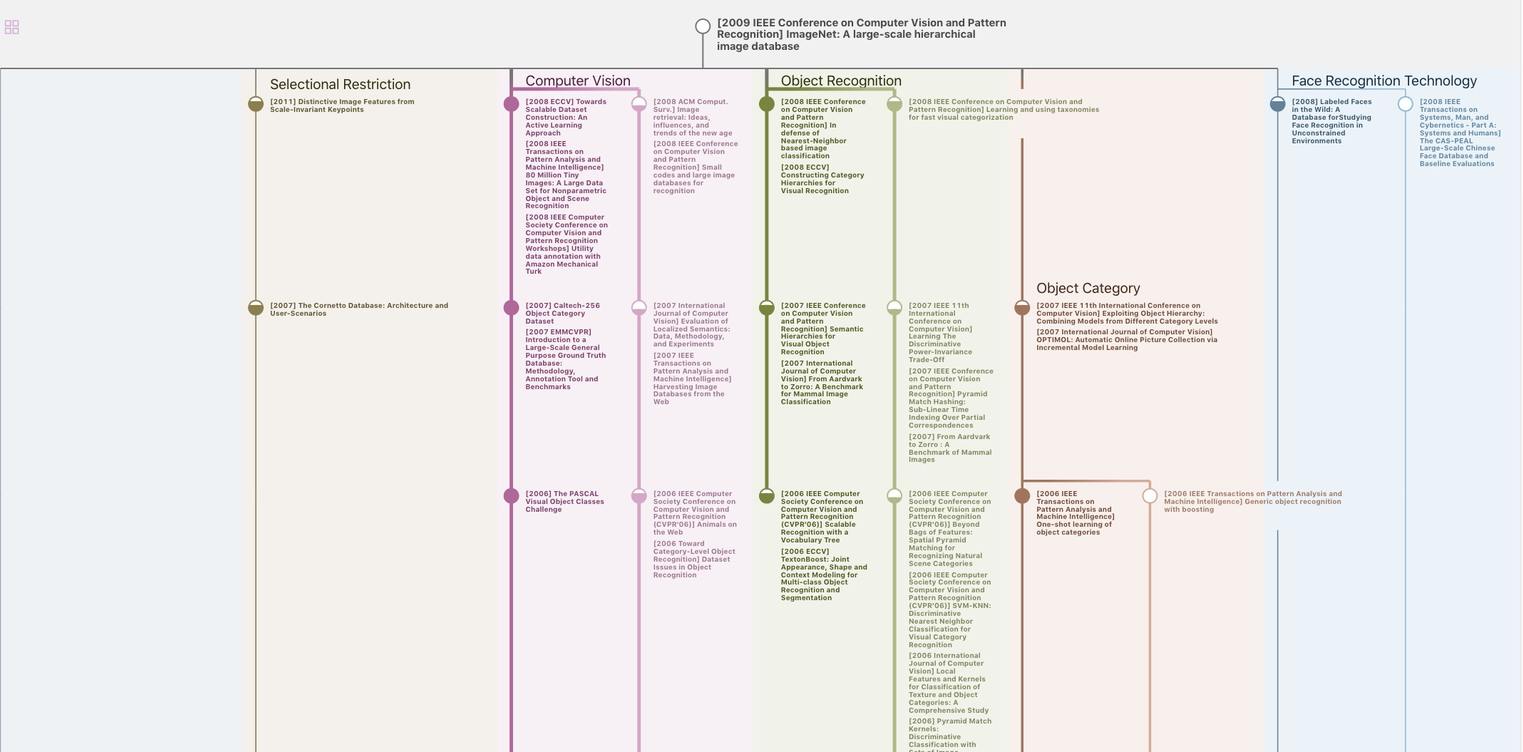
生成溯源树,研究论文发展脉络
Chat Paper
正在生成论文摘要