StockMixer: A Simple Yet Strong MLP-Based Architecture for Stock Price Forecasting
AAAI 2024(2024)
摘要
Stock price forecasting is a fundamental yet challenging task in quantitative investment. Various researchers have developed a combination of neural network models (e.g., RNNs, GNNs, Transformers) for capturing complex indicator, temporal and stock correlations of the stock data.While complex architectures are highly expressive, they are often difficult to optimize and the performances are often compromised by the limited stock data. In this paper, we propose a simple MLP-based architecture named StockMixer which is easy to optimize and enjoys strong predictive performance. StockMixer performs indicator mixing, followed by time mixing, and finally stock mixing. Unlike the standard MLP-based mixing, we devise the time mixing to exchange multi-scale time patch information and realize the stock mixing by exploiting stock-to-market and market-to-stock influences explicitly. Extensive experiments on real stock benchmarks demonstrate our proposed StockMixer outperforms various state-of-the-art forecasting methods with a notable margin while reducing memory usage and runtime cost.Code is available at https://github.com/SJTU-Quant/StockMixer.
更多查看译文
关键词
DMKM: Mining of Spatial, Temporal or Spatio-Temporal Data,DMKM: Applications
AI 理解论文
溯源树
样例
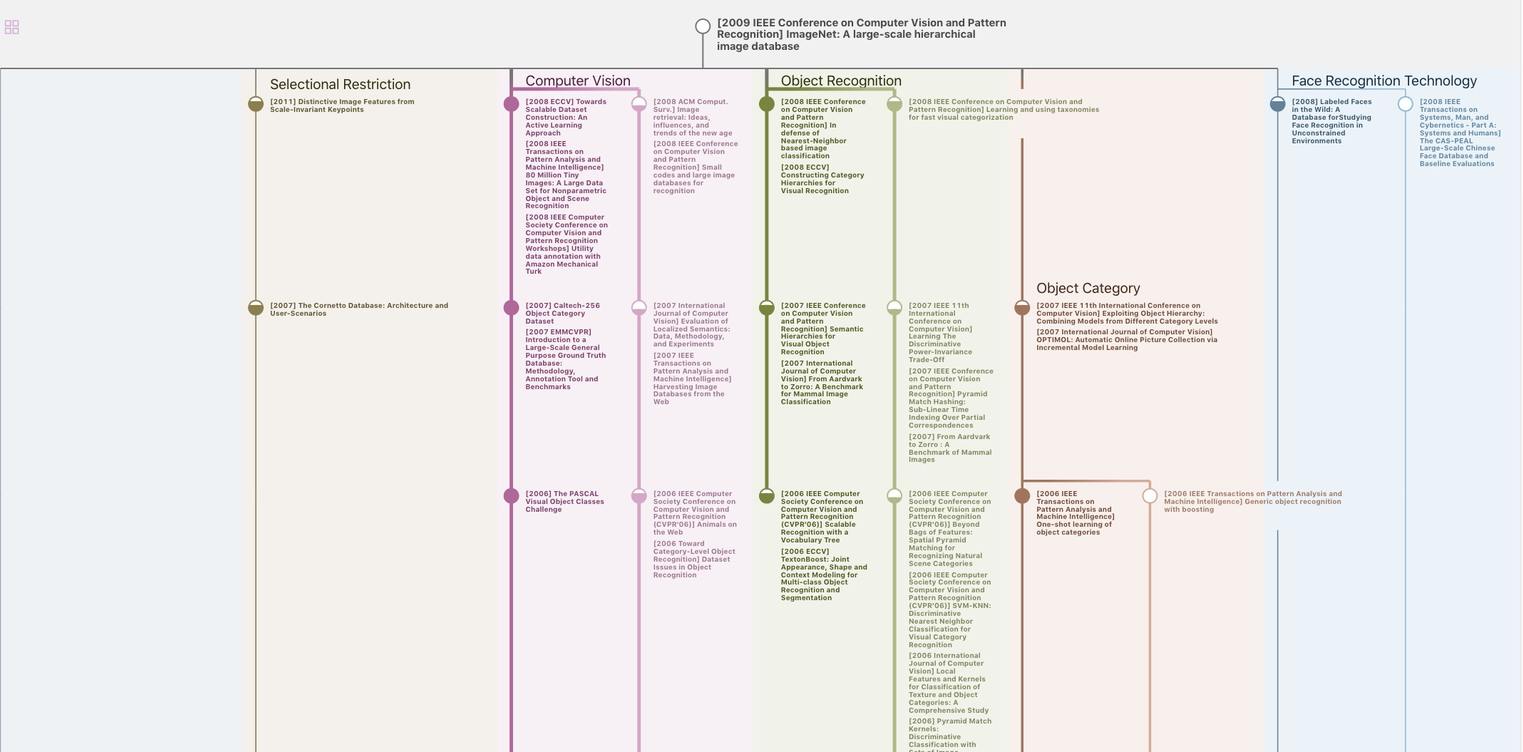
生成溯源树,研究论文发展脉络
Chat Paper
正在生成论文摘要