Intelligent Calibration for Bias Reduction in Sentiment Corpora Annotation Process
AAAI 2024(2024)
摘要
This paper focuses in the inherent anchoring bias present in sequential reviews-sentiment corpora annotation processes. It proposes employing a limited subset of meticulously chosen reviews at the outset of the process, as a means of calibration, effectively mitigating the phenomenon. Through extensive experimentation we validate the phenomenon of sentiment bias in the annotation process and show that its magnitude can be influenced by pre-calibration. Furthermore, we show that the choice of the calibration set matters, hence the need for effective guidelines for choosing the reviews to be included in it. A comparison of annotators performance with the proposed calibration to annotation processes that do not use calibration or use a randomly-picked calibration set, reveals that indeed the calibration set picked is highly effective---it manages to substantially reduce the average absolute error compared to the other cases. Furthermore, the proposed selection guidelines are found to be highly robust in picking an effective calibration set also for domains different than the one based on which these rules were extracted.
更多查看译文
关键词
HAI: Crowd Sourcing and Human Computation
AI 理解论文
溯源树
样例
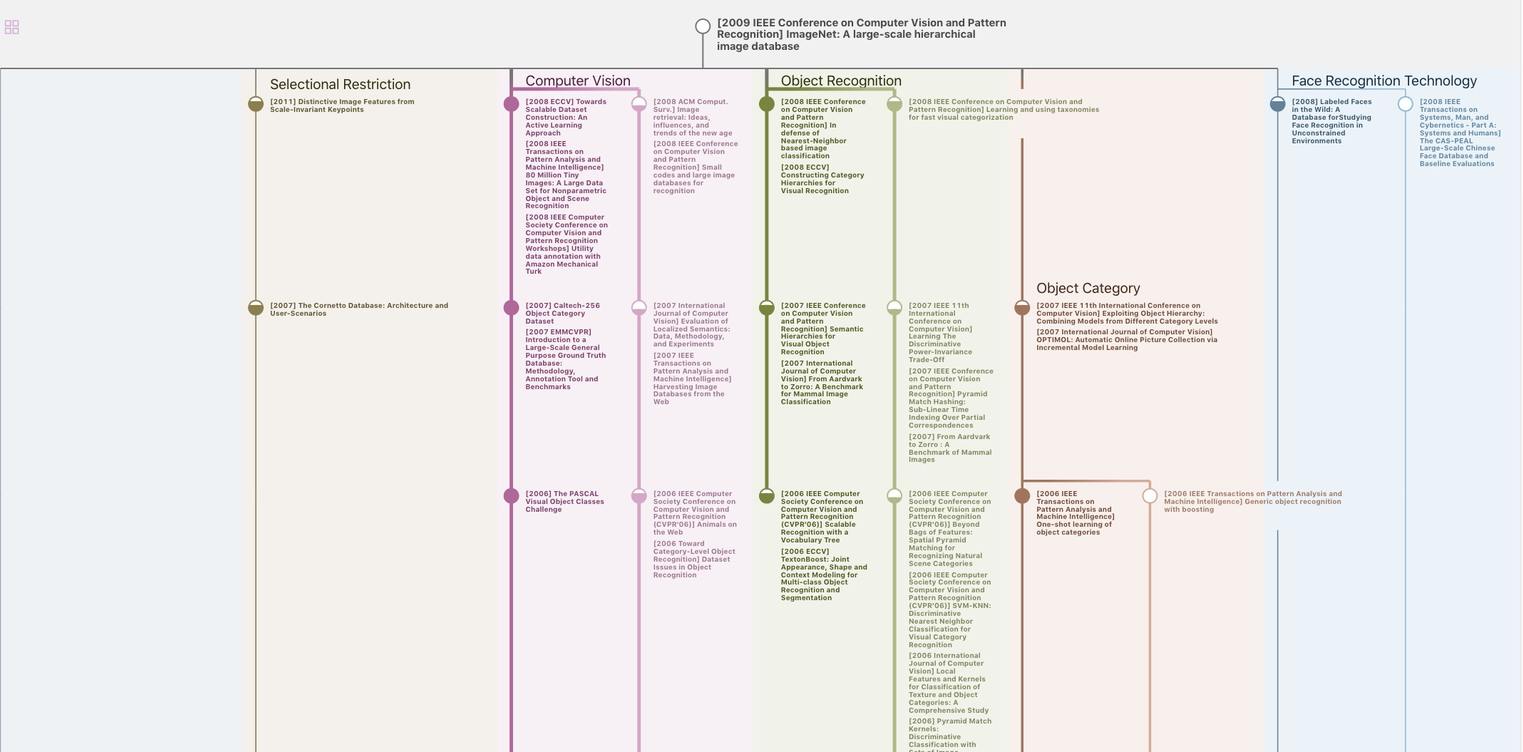
生成溯源树,研究论文发展脉络
Chat Paper
正在生成论文摘要