Spotting the Unseen: Reciprocal Consensus Network Guided by Visual Archetypes
AAAI 2024(2024)
摘要
Humans often require only a few visual archetypes to spot novel objects. Based on this observation, we present a strategy rooted in ``spotting the unseen" by establishing dense correspondences between potential query image regions and a visual archetype, and we propose the Consensus Network (CoNet). Our method leverages relational patterns intra and inter images via Auto-Correlation Representation (ACR) and Mutual-Correlation Representation (MCR). Within each image, the ACR module is capable of encoding both local self-similarity and global context simultaneously. Between the query and support images, the MCR module computes the cross-correlation across two image representations and introduces a reciprocal consistency constraint, which can incorporate to exclude outliers and enhance model robustness. To overcome the challenges of low-resource training data, particularly in one-shot learning scenarios, we incorporate an adaptive margin strategy to better handle diverse instances. The experimental results indicate the effectiveness of the proposed method across diverse domains such as object detection in natural scenes, and text spotting in both historical manuscripts and natural scenes, which demonstrates its sparkling generalization ability. Our code is available at: https://github.com/infinite-hwb/conet.
更多查看译文
关键词
ML: Deep Learning Algorithms,APP: Humanities & Computational Social Science,CV: Applications,CV: Object Detection & Categorization
AI 理解论文
溯源树
样例
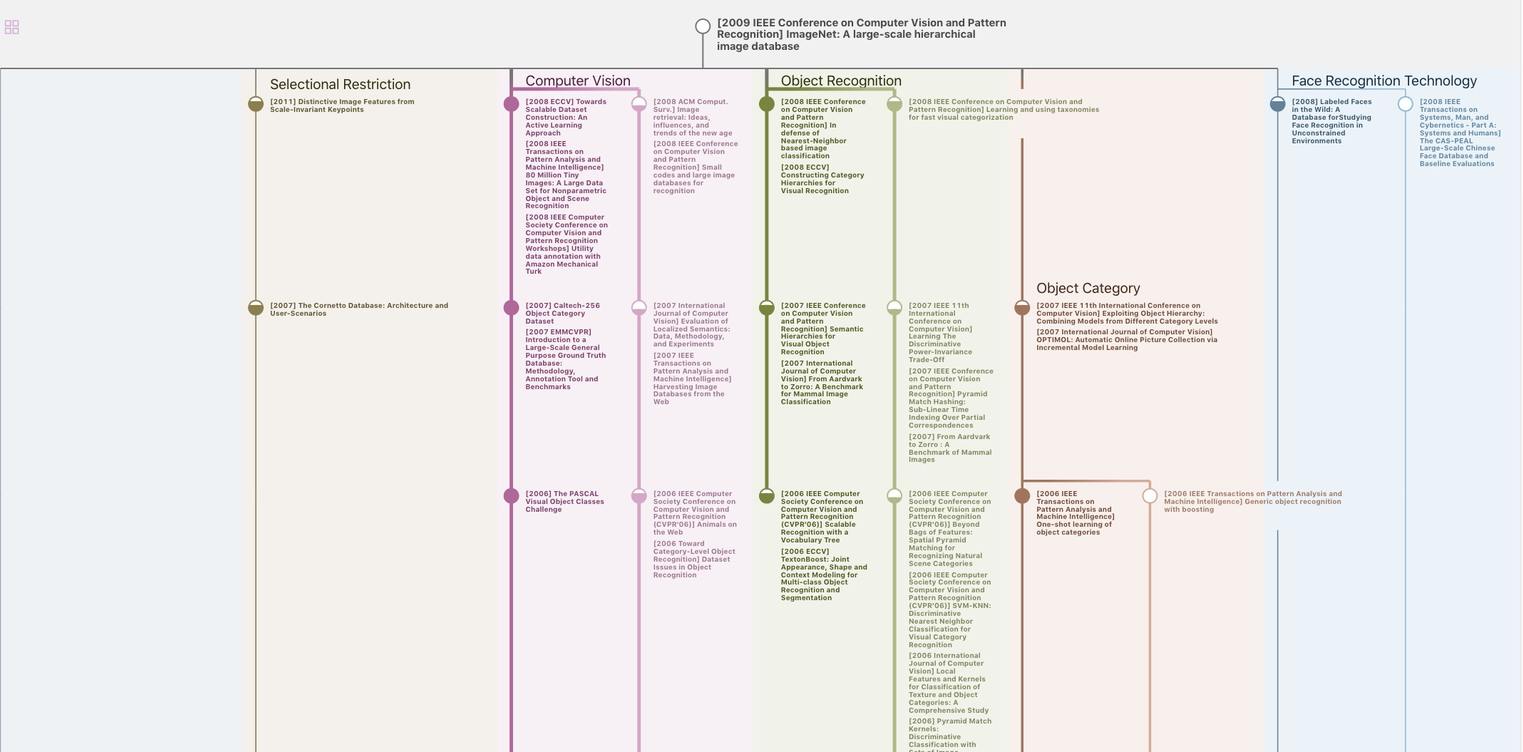
生成溯源树,研究论文发展脉络
Chat Paper
正在生成论文摘要