Distributed Manifold Hashing for Image Set Classification and Retrieval
AAAI 2024(2024)
摘要
Conventional image set methods typically learn from image sets stored in one location. However, in real-world applications, image sets are often distributed or collected across different positions. Learning from such distributed image sets presents a challenge that has not been studied thus far. Moreover, efficiency is seldom addressed in large-scale image set applications. To fulfill these gaps, this paper proposes Distributed Manifold Hashing (DMH), which models distributed image sets as a connected graph. DMH employs Riemannian manifold to effectively represent each image set and further suggests learning hash code for each image set to achieve efficient computation and storage. DMH is formally formulated as a distributed learning problem with local consistency constraint on global variables among neighbor nodes, and can be optimized in parallel. Extensive experiments on three benchmark datasets demonstrate that DMH achieves highly competitive accuracies in a distributed setting and provides faster classification and retrieval than state-of-the-arts.
更多查看译文
关键词
CV: Image and Video Retrieval
AI 理解论文
溯源树
样例
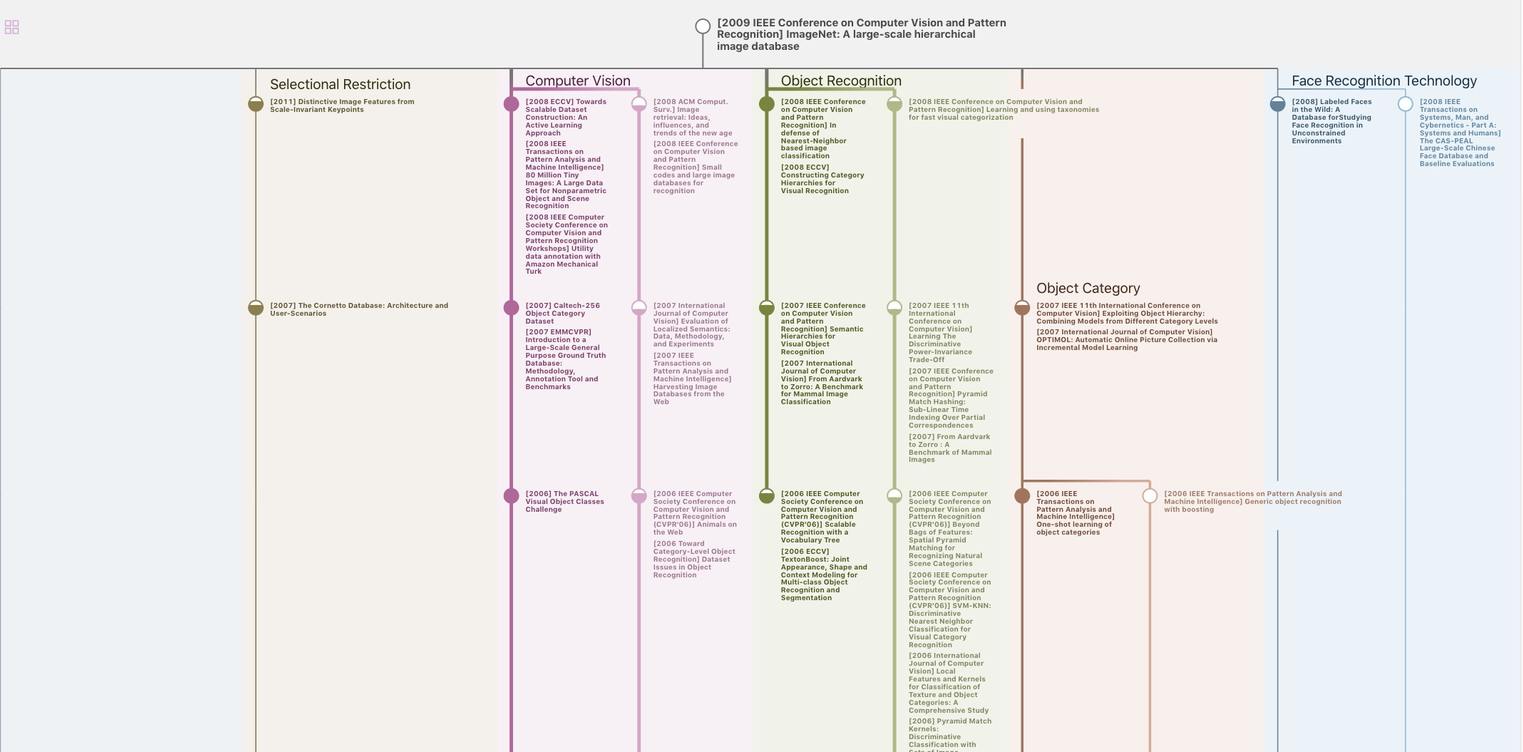
生成溯源树,研究论文发展脉络
Chat Paper
正在生成论文摘要