Focus-Then-Decide: Segmentation-Assisted Reinforcement Learning
AAAI 2024(2024)
摘要
Visual Reinforcement Learning (RL) is a promising approach to achieve human-like intelligence. However, it currently faces challenges in learning efficiently within noisy environments. In contrast, humans can quickly identify task-relevant objects in distraction-filled surroundings by applying previously acquired common knowledge. Recently, foundational models in natural language processing and computer vision have achieved remarkable successes, and the common knowledge within these models can significantly benefit downstream task training. Inspired by these achievements, we aim to incorporate common knowledge from foundational models into visual RL. We propose a novel Focus-Then-Decide (FTD) framework, allowing the agent to make decisions based solely on task-relevant objects. To achieve this, we introduce an attention mechanism to select task-relevant objects from the object set returned by a foundational segmentation model, and only use the task-relevant objects for the subsequent training of the decision module. Additionally, we specifically employed two generic self-supervised objectives to facilitate the rapid learning of this attention mechanism. Experimental results on challenging tasks based on DeepMind Control Suite and Franka Emika Robotics demonstrate that our method can quickly and accurately pinpoint objects of interest in noisy environments. Consequently, it achieves a significant performance improvement over current state-of-the-art algorithms.
Project Page: https://www.lamda.nju.edu.cn/chenc/FTD.html
Code: https://github.com/LAMDA-RL/FTD
更多查看译文
关键词
ML: Reinforcement Learning,CV: Applications
AI 理解论文
溯源树
样例
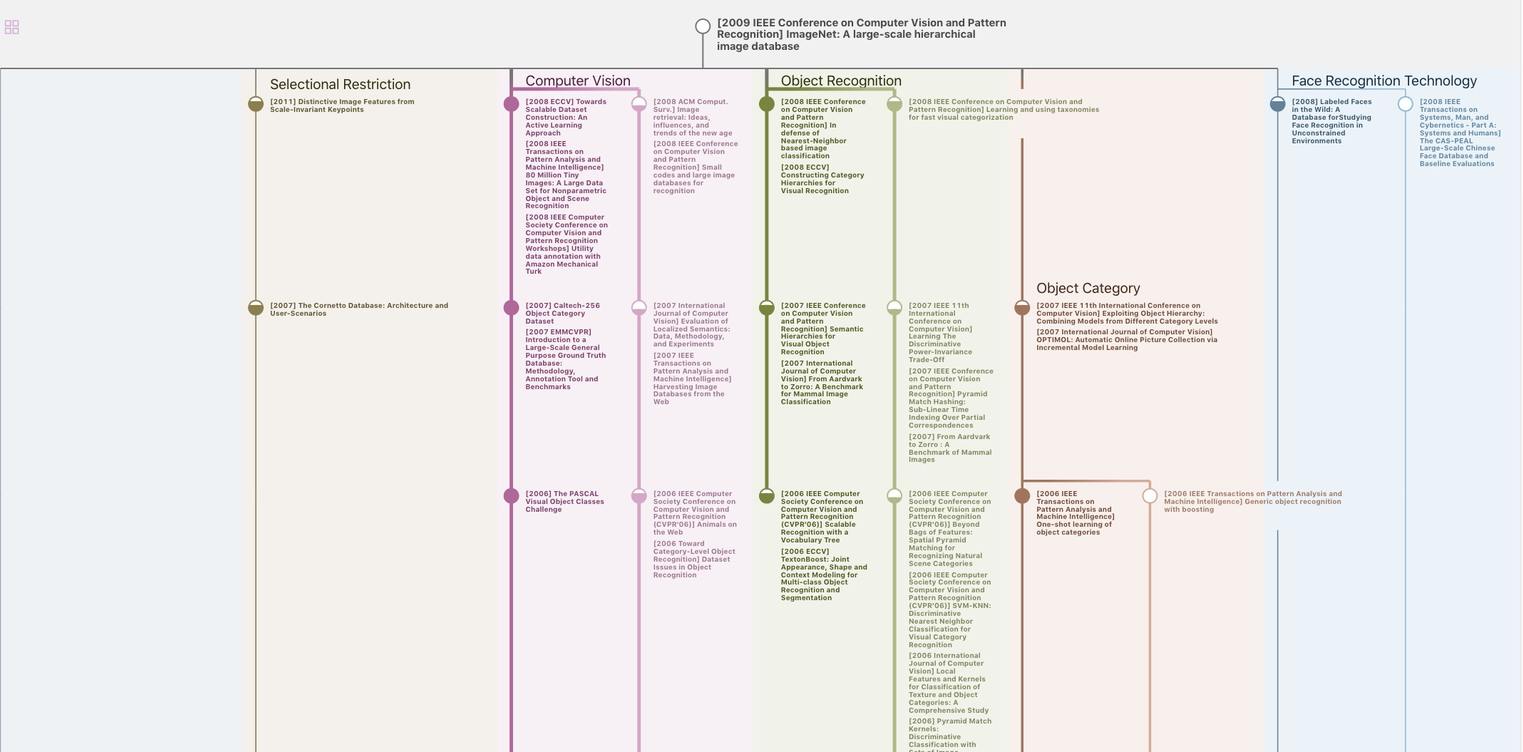
生成溯源树,研究论文发展脉络
Chat Paper
正在生成论文摘要