Better than Random: Reliable NLG Human Evaluation with Constrained Active Sampling
AAAI 2024(2024)
摘要
Human evaluation is viewed as a reliable evaluation method for NLG which is expensive and time-consuming. To save labor and costs, researchers usually perform human evaluation on a small subset of data sampled from the whole dataset in practice. However, different selection subsets will lead to different rankings of the systems. To give a more correct inter-system ranking and make the gold standard human evaluation more reliable, we propose a Constrained Active Sampling Framework (CASF) for reliable human judgment. CASF operates through a Learner, a Systematic Sampler and a Constrained Controller to select representative samples for getting a more correct inter-system ranking. Experiment results on 137 real NLG evaluation setups with 44 human evaluation metrics across 16 datasets and 5 NLG tasks demonstrate CASF receives 93.18\% top-ranked system recognition accuracy and ranks first or ranks second on 90.91\% of the human metrics with 0.83 overall inter-system ranking Kendall correlation. Code and data are publicly available online.
更多查看译文
关键词
NLP: Generation,NLP: Interpretability, Analysis, and Evaluation of NLP Models
AI 理解论文
溯源树
样例
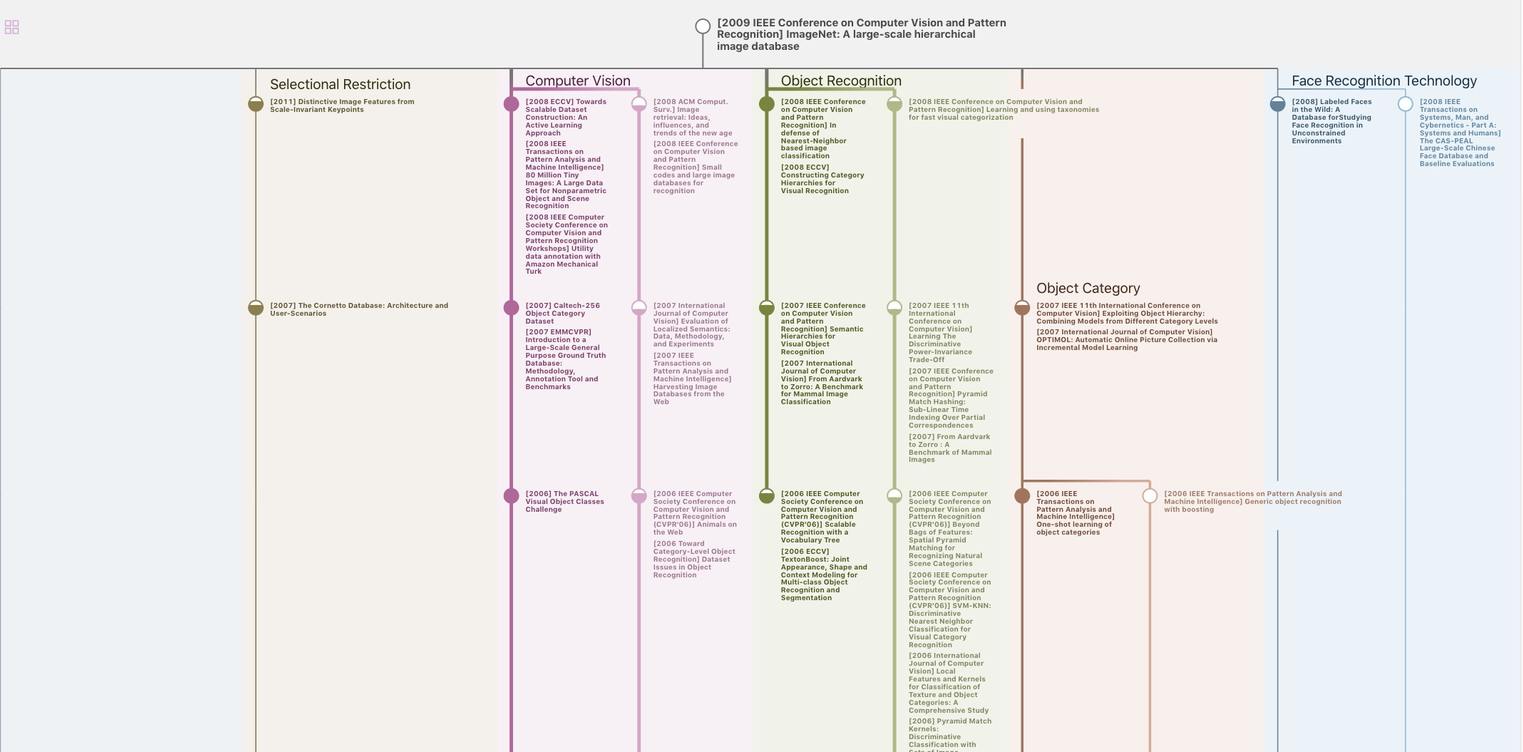
生成溯源树,研究论文发展脉络
Chat Paper
正在生成论文摘要