Faster Stochastic Variance Reduction Methods for Compositional MiniMax Optimization
AAAI 2024(2024)
摘要
This paper delves into the realm of stochastic optimization for compositional minimax optimization—a pivotal challenge across various machine learning domains, including deep AUC and reinforcement learning policy evaluation. Despite its significance, the problem of compositional minimax optimization is still under-explored. Adding to the complexity, current methods of compositional minimax optimization are plagued by sub-optimal complexities or heavy reliance on sizable batch sizes. To respond to these constraints, this paper introduces a novel method, called Nested STOchastic Recursive Momentum (NSTORM), which can achieve the optimal sample complexity and obtain the nearly accuracy solution, matching the existing minimax methods. We also demonstrate that NSTORM can achieve the same sample complexity under the Polyak-Lojasiewicz (PL)-condition—an insightful extension of its capabilities. Yet, NSTORM encounters an issue with its requirement for low learning rates, potentially constraining its real-world applicability in machine learning. To overcome this hurdle, we present ADAptive NSTORM (ADA-NSTORM) with adaptive learning rates. We demonstrate that ADA-NSTORM can achieve the same sample complexity but the experimental results show its more effectiveness. All the proposed complexities indicate that our proposed methods can match lower bounds to existing minimax optimizations, without requiring a large batch size in each iteration. Extensive experiments support the efficiency of our proposed methods.
更多查看译文
关键词
ML: Optimization,SO: Non-convex Optimization
AI 理解论文
溯源树
样例
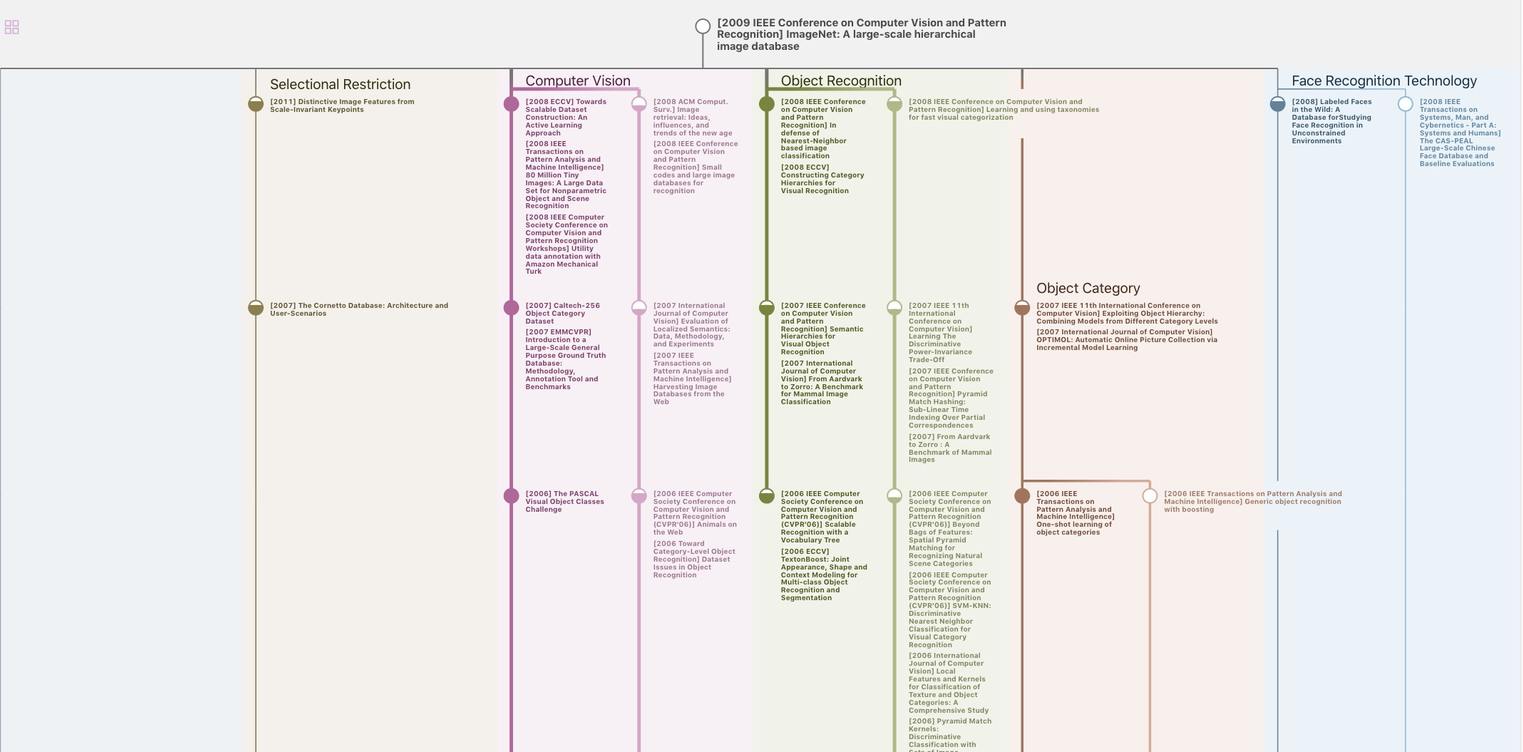
生成溯源树,研究论文发展脉络
Chat Paper
正在生成论文摘要