Reward Shaping in Reinforcement Learning of Multi-Objective Safety Critical Systems
2024 20th CSI International Symposium on Artificial Intelligence and Signal Processing (AISP)(2024)
摘要
Reinforcement learning (RL) depends critically on how well the reward function formulates the aim of the application. In safety-critical systems, several safety properties should be met during the RL process in addition to achieving the primary target. In this paper, we utilize the reward shaping technique to guide the RL algorithm in a way that satisfies the target and safety properties and fulfills other properties, such as efficiency, as much as possible. Reward shaping is a technique to enhance the performance of RL by adding more information to the reward function. Reward shaping functions utilize the system’s main target for the base reward signal and add some other reward signals based on other system properties. Suppose each property has its own reward function. In this case, the reward is a vector rather than a scalar value, and the original reinforcement learning approach converts to multi-objective reinforcement learning (MORL). In MORL, one scalarized objective can be defined by fusing several objectives. This paper proposes an aggregation method based on prioritized aggregation operators for scalarizing multiple reward functions. We illustrate our method with two case studies: cart-pole with an obstacle and lunar-lander. The simulation results show that our method guides the RL algorithm to converge to optimal policies faster than existing approaches.
更多查看译文
关键词
Reinforcement Learning (RL),Reward shaping,Safety-critical systems,Multi-objective RL (MORL)
AI 理解论文
溯源树
样例
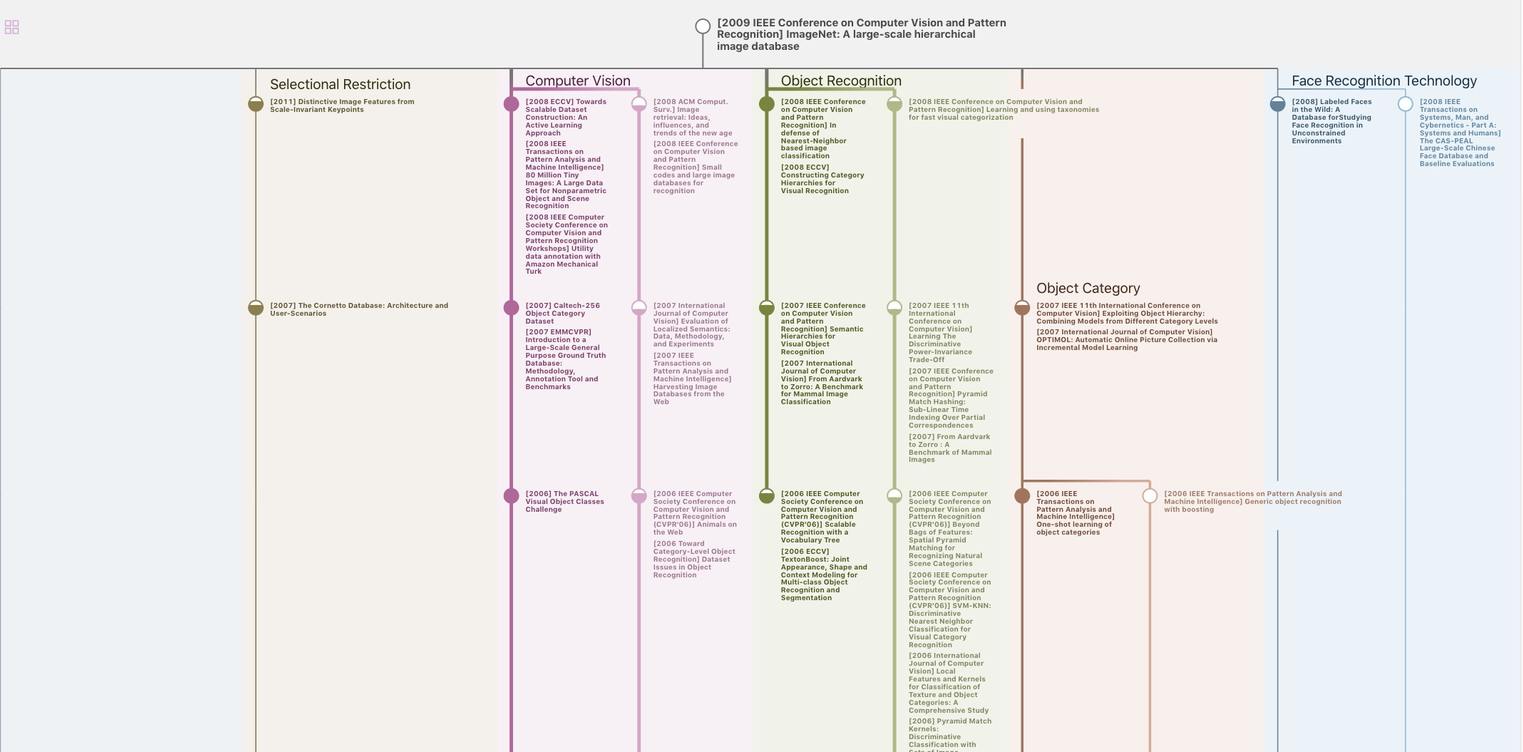
生成溯源树,研究论文发展脉络
Chat Paper
正在生成论文摘要