Exploiting Discrepancy in Feature Statistic for Out-of-Distribution Detection
AAAI 2024(2024)
摘要
Recent studies on out-of-distribution (OOD) detection focus on designing models or scoring functions that can effectively distinguish between unseen OOD data and in-distribution (ID) data. In this paper, we propose a simple yet novel ap-
proach to OOD detection by leveraging the phenomenon that the average of feature vector elements from convolutional neural network (CNN) is typically larger for ID data than for OOD data. Specifically, the average of feature vector elements is used as part of the scoring function to further separate OOD data from ID data. We also provide mathematical analysis to explain this phenomenon. Experimental evaluations demonstrate that, when combined with a strong baseline, our method can achieve state-of-the-art performance on several OOD detection benchmarks. Furthermore, our method can be easily integrated into various CNN architectures and requires less computation. Source code address: https://github.com/SYSU-MIA-GROUP/statistical_discrepancy_ood.
更多查看译文
关键词
PEAI: Safety, Robustness & Trustworthiness,CV: Object Detection & Categorization,CV: Other Foundations of Computer Vision
AI 理解论文
溯源树
样例
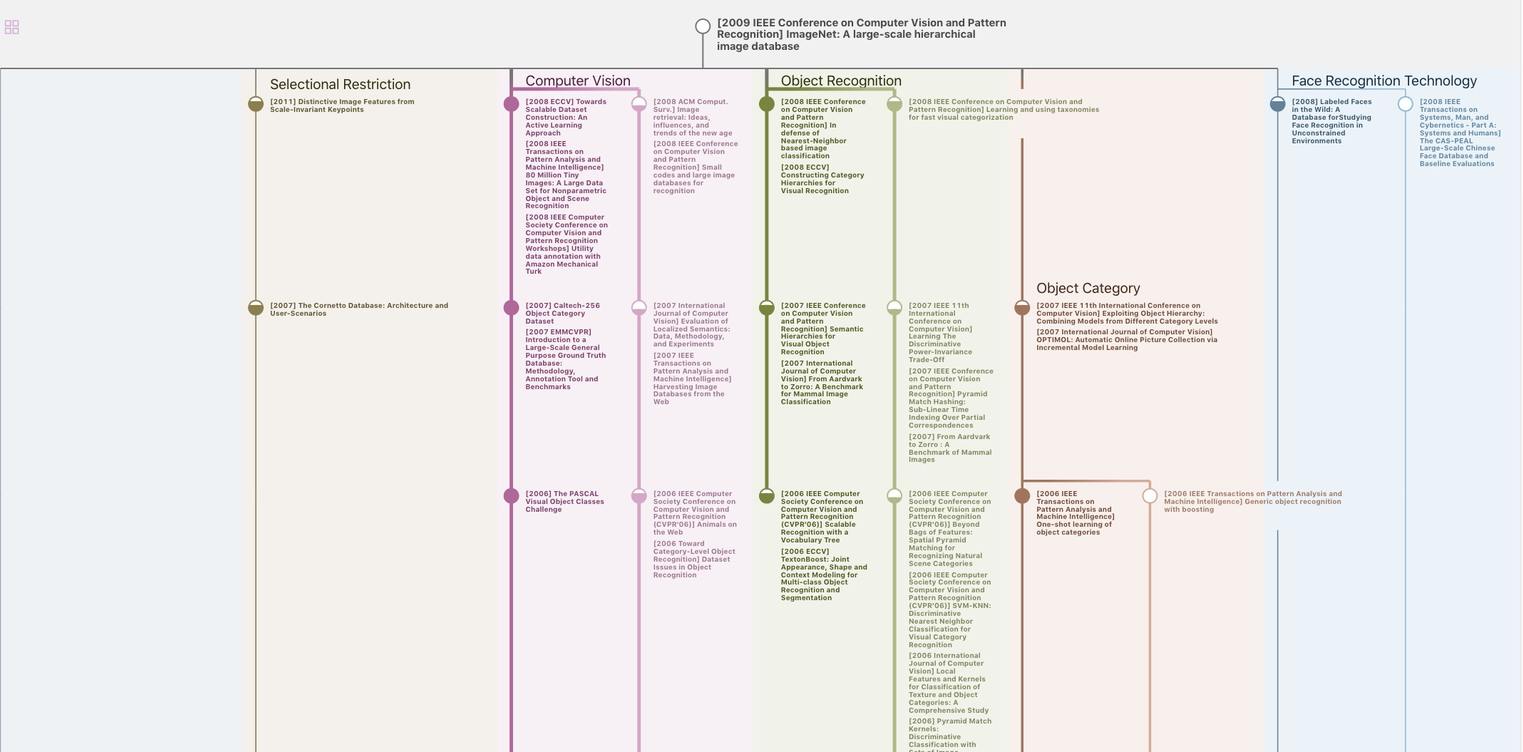
生成溯源树,研究论文发展脉络
Chat Paper
正在生成论文摘要